Ensemble Learning for Pea Yield Estimation Using Unmanned Aerial Vehicles, Red Green Blue, and Multispectral Imagery
DRONES(2024)
摘要
Peas are one of the most important cultivated legumes worldwide, for which early yield estimations are helpful for agricultural planning. The unmanned aerial vehicles (UAVs) have become widely used for crop yield estimations, owing to their operational convenience. In this study, three types of sensor data (red green blue [RGB], multispectral [MS], and a fusion of RGB and MS) across five growth stages were applied to estimate pea yield using ensemble learning (EL) and four base learners (Cubist, elastic net [EN], K nearest neighbor [KNN], and random forest [RF]). The results showed the following: (1) the use of fusion data effectively improved the estimation accuracy in all five growth stages compared to the estimations obtained using a single sensor; (2) the mid filling growth stage provided the highest estimation accuracy, with coefficients of determination (R2) reaching up to 0.81, 0.8, 0.58, and 0.77 for the Cubist, EN, KNN, and RF algorithms, respectively; (3) the EL algorithm achieved the best performance in estimating pea yield than base learners; and (4) the different models were satisfactory and applicable for both investigated pea types. These results indicated that the combination of dual-sensor data (RGB + MS) from UAVs and appropriate algorithms can be used to obtain sufficiently accurate pea yield estimations, which could provide valuable insights for agricultural remote sensing research.
更多查看译文
关键词
machine learning,fusion data,unmanned aerial vehicles,cold-tolerant peas,common peas
AI 理解论文
溯源树
样例
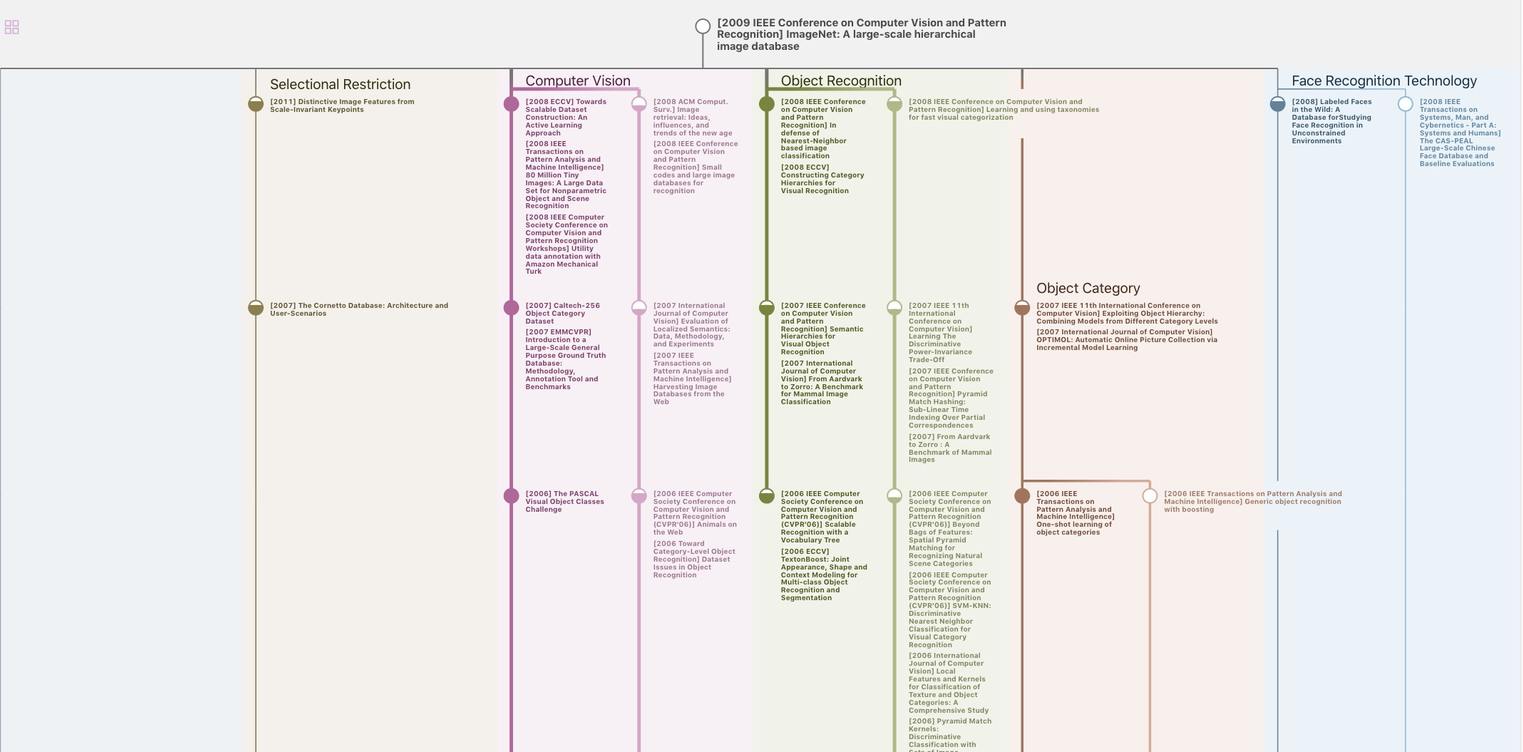
生成溯源树,研究论文发展脉络
Chat Paper
正在生成论文摘要