Hyperspectral Vegetation Indices and Their Relationships with Agricultural Crop Characteristics
Remote Sensing of Environment(2000)SCI 1区
Yale Univ | Yale University | International Center for Agricultural Research in the Dry Areas
- Pretraining has recently greatly promoted the development of natural language processing (NLP)
- We show that M6 outperforms the baselines in multimodal downstream tasks, and the large M6 with 10 parameters can reach a better performance
- We propose a method called M6 that is able to process information of multiple modalities and perform both single-modal and cross-modal understanding and generation
- The model is scaled to large model with 10 billion parameters with sophisticated deployment, and the 10 -parameter M6-large is the largest pretrained model in Chinese
- Experimental results show that our proposed M6 outperforms the baseline in a number of downstream tasks concerning both single modality and multiple modalities We will continue the pretraining of extremely large models by increasing data to explore the limit of its performance
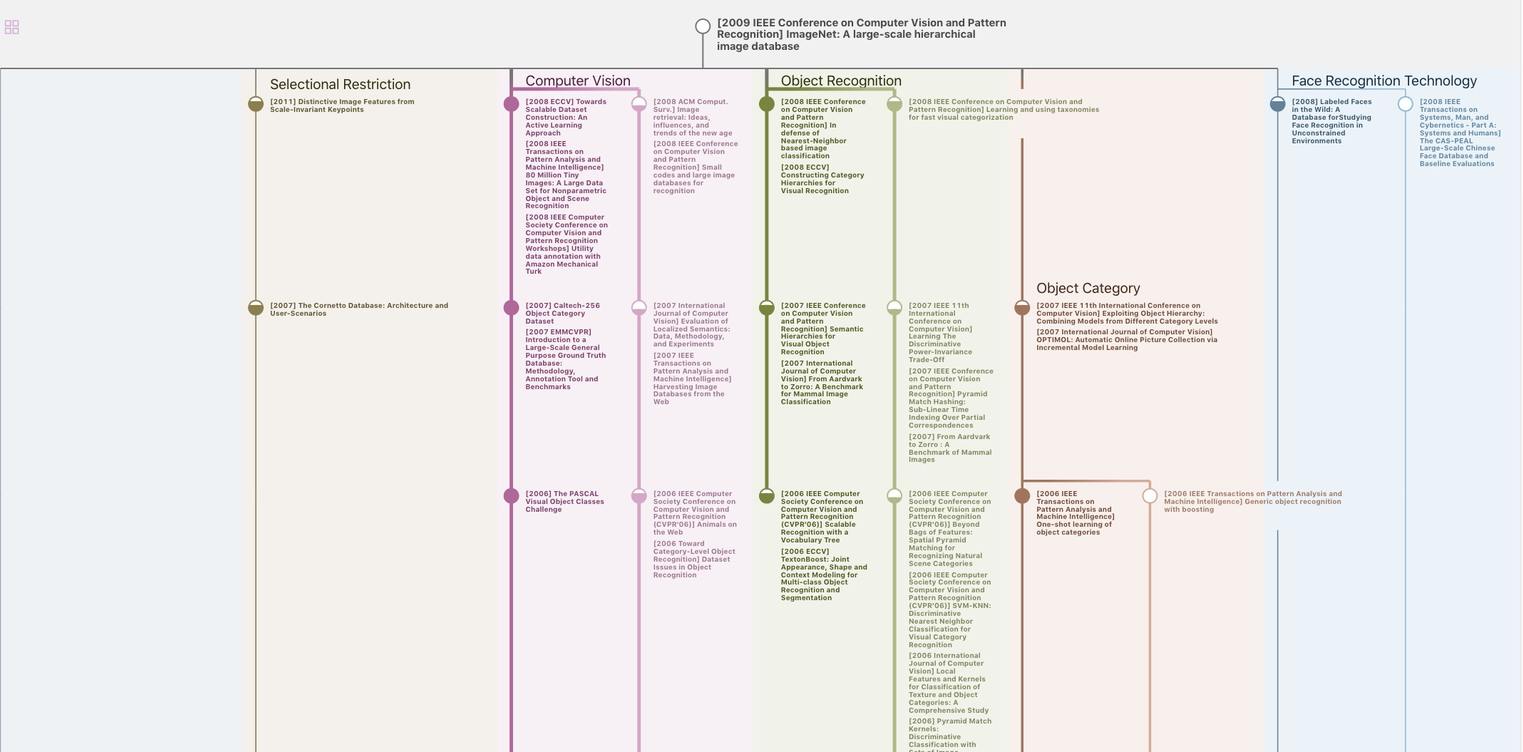
Estimation of Grassland Biomass and Nitrogen Using MERIS Data.
被引用77
被引用96
Assessment of the Nitrogen Management Strategy Using an Optical Sensor for Irrigated Wheat
被引用88
Utility of Hyperspectral Reflectance for Differentiating Soybean (glycine Max) and Six Weed Species
被引用30
Flooding: the Effect of Water Depth on the Spectral Response of Grass Canopies
被引用36
被引用29
Determination of Dry Matter Yield from Legume–Grass Swards by Field Spectroscopy
被引用25
The Estimation Models of Rape Biomass Yield Using Hyperspectral Data
被引用2
被引用148
被引用48
Hyperspectral Versus Multispectral Data for Estimating Leaf Area Index in Four Different Biomes
被引用252
被引用1057
Monitoring Biomass of Water Hyacinth by Using Hyperspectral Remote Sensing
被引用0
被引用46
Generic Wavelet-Based Hyperspectral Classification Applied to Vegetation Stress Detection
被引用44
Spectral and Spatial Variation at Leaf and Patch Scale of Invasive Wetland Weeds
被引用0
Context-aided Tracking with an Adaptive Hyperspectral Sensor
被引用26
被引用40
Determination of Leaf Area Index in Agricultural Systems
被引用31
Estimation of Plant Nitrogen Concentration in Paddy Rice from Field Canopy Spectra
被引用27
Usingin Situhyperspectral Reflectance Data to Distinguish Nine Aquatic Plant Species
被引用7
被引用111
被引用116