Exploring Chitosan Lactate As a Multifunctional Additive: Enhancing Quality and Extending Shelf Life of Whole Wheat Bread
Foods(2024)
Parul Univ | Natl Inst Technol Rourkela | Seoul Natl Univ | Qingdao Agr Univ | Poznan Univ Life Sci
Abstract
The shelf life of whole wheat bread (WWB) significantly impacts its freshness and overall quality. This research investigated the impact of chitosan lactate (CL) on various characteristics influencing the shelf life of WWB, including its physical, chemical, textural, antimicrobial, and sensory attributes. These characteristics were evaluated by conducting various experiments such as physical inspection, moisture, impedance, swelling, color, texture, FTIR, microbiological, and sensory analysis. CL with different concentrations was incorporated into WWB formulations: P0.0 (0.0% w/w CL, control), P0.5 (0.5% w/w CL), P1.0 (1.0% w/w CL), P2.0 (2.0% w/w CL), and P3.0 (3.0% w/w CL). The inclusion of CL promoted the Maillard reaction (MR) compared to P0.0. The promotion of MR resulted in the formation of a shinier crust, which increased as the CL content was increased. P0.5 comprised large-sized pores and exhibited increased loaf height. CL-containing WWB formulations showed an increased moisture content and decreased impedance values compared to the control. FTIR analysis of P0.5 demonstrated the enhanced interaction and bonding of water molecules. P0.5 demonstrated optimal textural, colorimetric, and antimicrobial properties compared to other formulations. The sensory attributes of WWBs remain unchanged despite CL addition. In conclusion, P0.5 exhibited optimal characteristics associated with better quality and prolonged shelf life.
MoreTranslated text
Key words
bakery,chitosan derivatives,overall quality,polysaccharides,storage life,texture
求助PDF
上传PDF
View via Publisher
AI Read Science
AI Summary
AI Summary is the key point extracted automatically understanding the full text of the paper, including the background, methods, results, conclusions, icons and other key content, so that you can get the outline of the paper at a glance.
Example
Background
Key content
Introduction
Methods
Results
Related work
Fund
Key content
- Pretraining has recently greatly promoted the development of natural language processing (NLP)
- We show that M6 outperforms the baselines in multimodal downstream tasks, and the large M6 with 10 parameters can reach a better performance
- We propose a method called M6 that is able to process information of multiple modalities and perform both single-modal and cross-modal understanding and generation
- The model is scaled to large model with 10 billion parameters with sophisticated deployment, and the 10 -parameter M6-large is the largest pretrained model in Chinese
- Experimental results show that our proposed M6 outperforms the baseline in a number of downstream tasks concerning both single modality and multiple modalities We will continue the pretraining of extremely large models by increasing data to explore the limit of its performance
Upload PDF to Generate Summary
Must-Reading Tree
Example
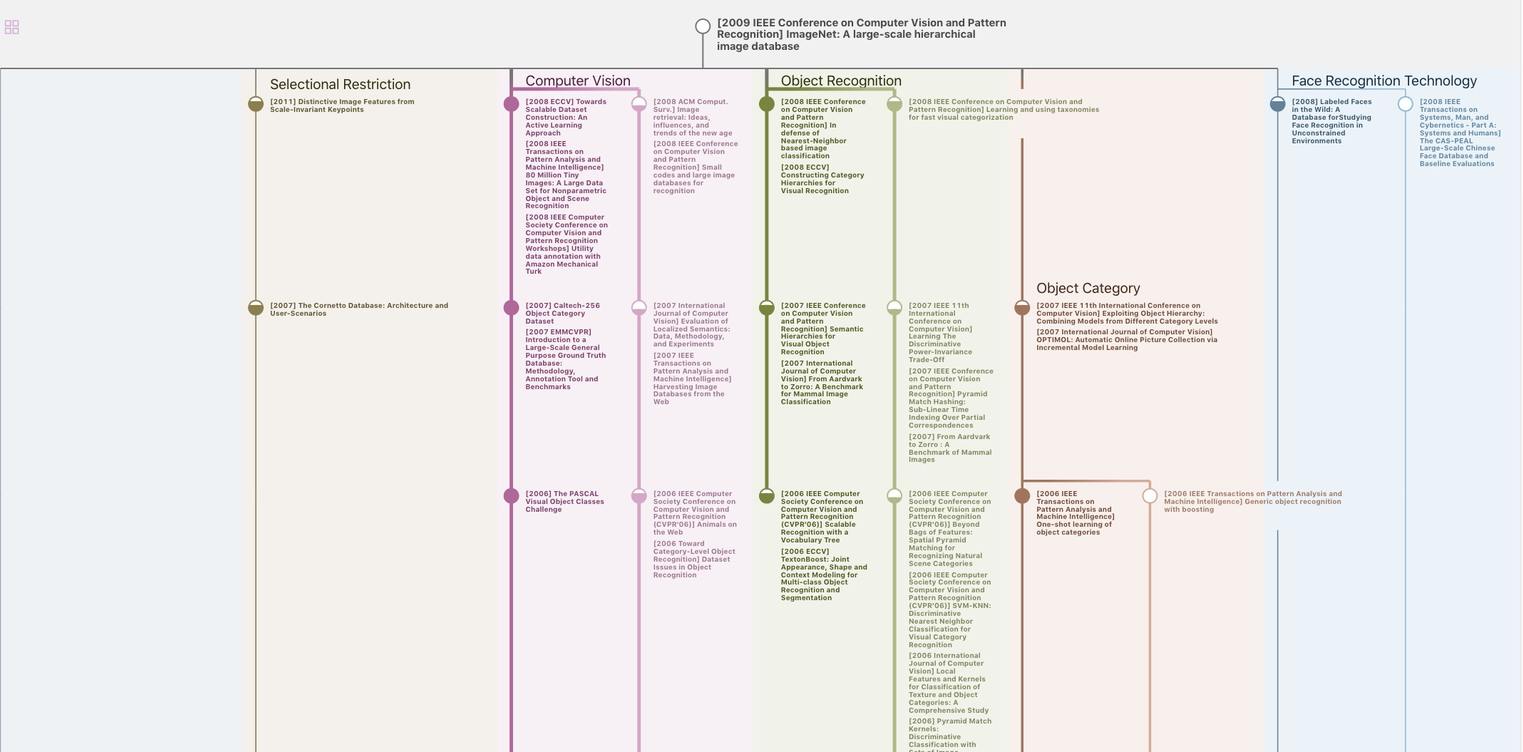
Generate MRT to find the research sequence of this paper
Related Papers
2006
被引用366 | 浏览
2004
被引用80 | 浏览
2002
被引用628 | 浏览
2010
被引用7 | 浏览
2008
被引用190 | 浏览
2007
被引用732 | 浏览
2002
被引用277 | 浏览
2013
被引用23 | 浏览
2016
被引用37 | 浏览
2017
被引用297 | 浏览
2020
被引用26 | 浏览
2021
被引用67 | 浏览
2021
被引用30 | 浏览
2021
被引用7 | 浏览
2024
被引用2 | 浏览
Data Disclaimer
The page data are from open Internet sources, cooperative publishers and automatic analysis results through AI technology. We do not make any commitments and guarantees for the validity, accuracy, correctness, reliability, completeness and timeliness of the page data. If you have any questions, please contact us by email: report@aminer.cn
Chat Paper