Medical Image Classifications Using Convolutional Neural Networks: A Survey of Current Methods and Statistical Modeling of the Literature.
MACHINE LEARNING AND KNOWLEDGE EXTRACTION(2024)
关键词
medical imaging,convolutional neural network models,classification,hyperparameter tuning,frameworks,preprocessing,performance metrics,ensemble,activation function
AI 理解论文
溯源树
样例
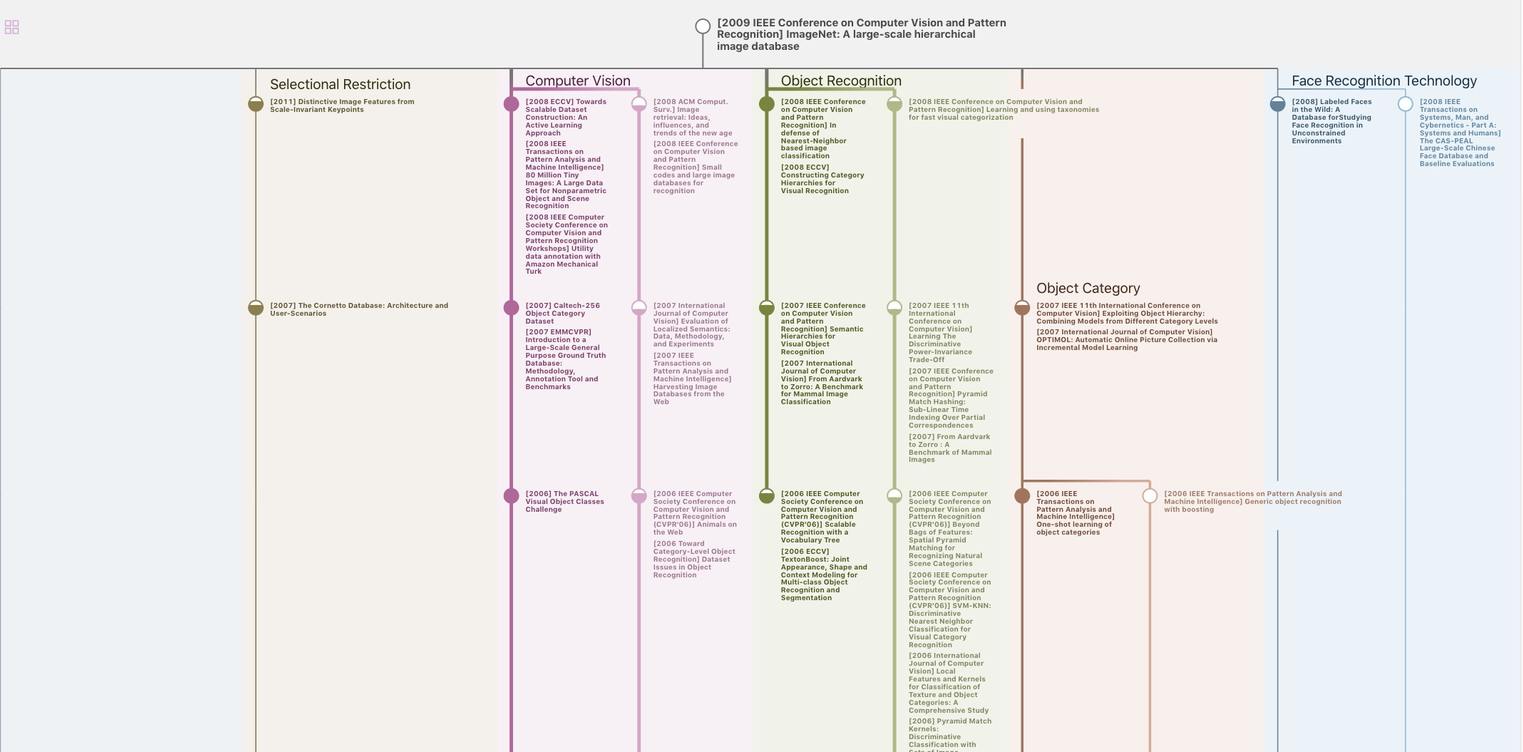
生成溯源树,研究论文发展脉络
Chat Paper
正在生成论文摘要