Multi-objective Optimization-Oriented Generative Adversarial Design for Multi-principal Element Alloys
Integrating Materials and Manufacturing Innovation(2024)
摘要
The discovery of novel alloys, such as multi-principal element alloys (MPEAs)—inclusive of the so-called high-entropy alloys—remains essential for technological advancement. Multi-principal element alloys can manifest uniquely favorable mechanical properties, but the complexity of their compositions results in their design and performance being challenging to understand. With the emergence of the materials genome concept, there is potential to pursue novel materials using computational design approaches. However, the complexity of such design often requires immense computational power and sophisticated data analysis. In an attempt to address this, we introduce the application of a new framework, the non-dominant sorting optimization-based generative adversarial networks (NSGAN) in the discovery and exploration of novel MPEAs. By harnessing the power of genetic algorithms and generative adversarial networks (GANs), NSGANs offer an effective solution for high-dimensional multi-objective optimization challenges in alloy design. The framework is demonstrated to generate MPEAs according to specific alloy properties. Furthermore, an online web tool/software applies the NSGAN framework to disseminate the methodology to the broader scientific arena (along with the supporting code made available).
更多查看译文
关键词
Alloy design,High-entropy alloys,Multi-principal element alloys,Genetic algorithm,Generative adversarial network,Non-dominant sorting optimization,Machine learning
AI 理解论文
溯源树
样例
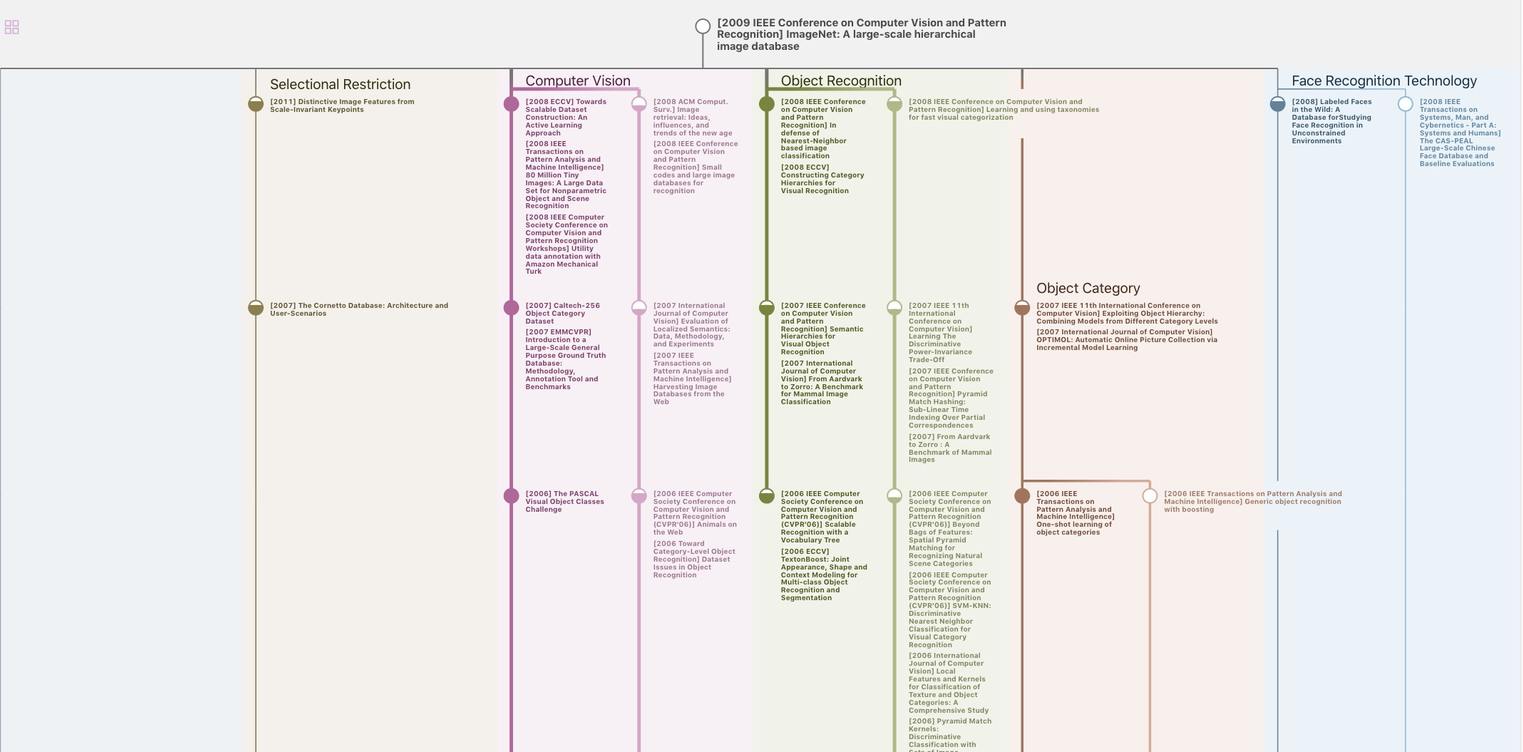
生成溯源树,研究论文发展脉络
Chat Paper
正在生成论文摘要