When are Foundation Models Effective? Understanding the Suitability for Pixel-Level Classification Using Multispectral Imagery
CoRR(2024)
Abstract
Foundation models, i.e., very large deep learning models, have demonstrated
impressive performances in various language and vision tasks that are otherwise
difficult to reach using smaller-size models. The major success of GPT-type of
language models is particularly exciting and raises expectations on the
potential of foundation models in other domains including satellite remote
sensing. In this context, great efforts have been made to build foundation
models to test their capabilities in broader applications, and examples include
Prithvi by NASA-IBM, Segment-Anything-Model, ViT, etc. This leads to an
important question: Are foundation models always a suitable choice for
different remote sensing tasks, and when or when not? This work aims to enhance
the understanding of the status and suitability of foundation models for
pixel-level classification using multispectral imagery at moderate resolution,
through comparisons with traditional machine learning (ML) and regular-size
deep learning models. Interestingly, the results reveal that in many scenarios
traditional ML models still have similar or better performance compared to
foundation models, especially for tasks where texture is less useful for
classification. On the other hand, deep learning models did show more promising
results for tasks where labels partially depend on texture (e.g., burn scar),
while the difference in performance between foundation models and deep learning
models is not obvious. The results conform with our analysis: The suitability
of foundation models depend on the alignment between the self-supervised
learning tasks and the real downstream tasks, and the typical masked
autoencoder paradigm is not necessarily suitable for many remote sensing
problems.
MoreTranslated text
AI Read Science
Must-Reading Tree
Example
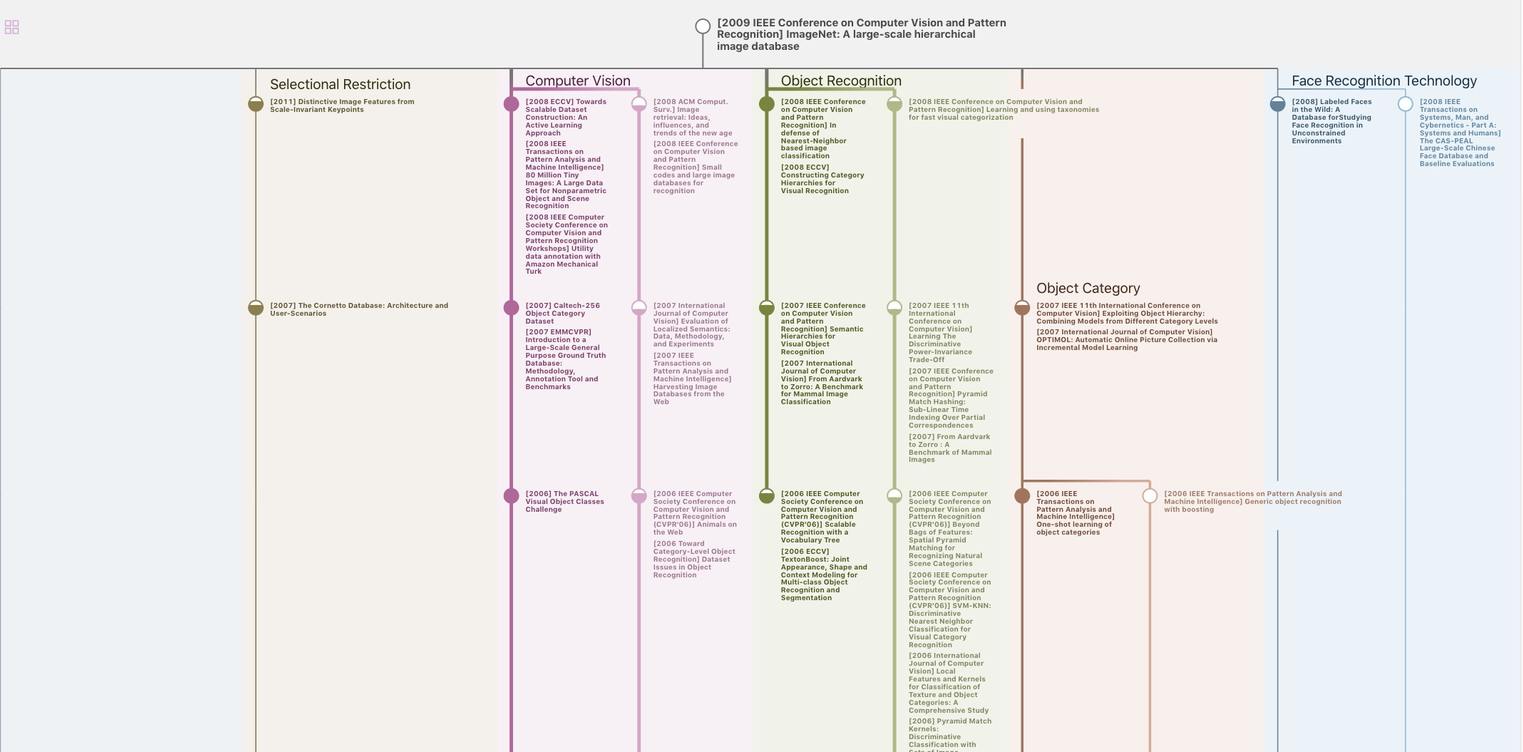
Generate MRT to find the research sequence of this paper
Chat Paper
Summary is being generated by the instructions you defined