Neural Directional Encoding for Efficient and Accurate View-Dependent Appearance Modeling
2024 IEEE/CVF Conference on Computer Vision and Pattern Recognition (CVPR)(2024)
摘要
Novel-view synthesis of specular objects like shiny metals or glossy paints remains a significant challenge. Not only the glossy appearance but also global illumination effects, including reflections of other objects in the environment, are critical components to faithfully reproduce a scene. In this paper, we present Neural Directional Encoding (NDE), a view-dependent appearance encoding of neural radiance fields (NeRF) for rendering specular objects. NDE transfers the concept of feature-grid-based spatial encoding to the angular domain, significantly improving the ability to model high-frequency angular signals. In contrast to previous methods that use encoding functions with only angular input, we additionally cone-trace spatial features to obtain a spatially varying directional encoding, which addresses the challenging interreflection effects. Extensive experiments on both synthetic and real datasets show that a NeRF model with NDE (1) outperforms the state of the art on view synthesis of specular objects, and (2) works with small networks to allow fast (real-time) inference. The project webpage and source code are available at: .
更多查看译文
关键词
NeRF,Neural Rendering
AI 理解论文
溯源树
样例
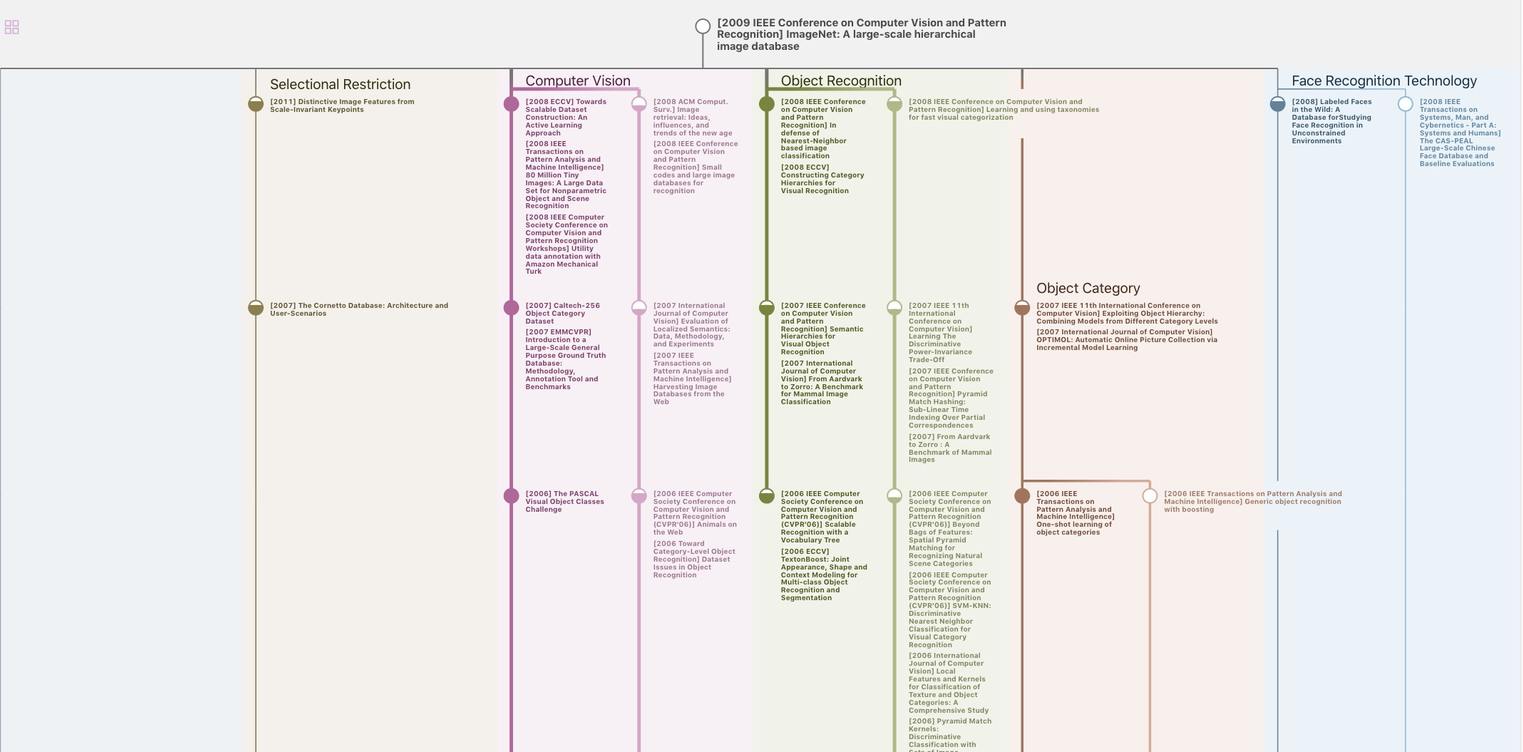
生成溯源树,研究论文发展脉络
Chat Paper
正在生成论文摘要