Reclassification of Clinical Exome Data Leads to Significant Clinical Assessment Changes in Almost Half of the Patients
CUKUROVA MEDICAL JOURNAL(2023)
Baskent Univ
Abstract
Purpose: With the global accumulation of genetic/clinical data, we are understanding the clinical significance of the reclassification of pathogenicity for gene variants. We hypothesized that this evolution in classification(s) may cause clinically-relevant discrepancies in the genetic risk assessment of subjects. In this study, we sought to reclassify the clinical exome sequence (CES) data of our patients to assess whether these changes would have clinical significance.Materials and Methods: The study included CES data of 23 cases diagnosed with cancer or familial cancer predisposition. The variants were first classified in 2020 and then reclassified a year after based on the ACMG database. Chart reviews were performed to record clinical history and interventions.Results: In the first classification of CES data, a total of 80 variants were identified as being not benign (26 likely pathogenic/pathogenic and 54 variants of undetermined significance (VUS)). The clinical significance of fifteen variants (19%) changed after reclassification in 10 patients (43%). The only upgraded variant was the c.9097 dup in exon 23 of BRCA2 gene (likely pathogenic to pathogenic). Fourteen variants were downgraded at reanalysis in 9 patients: from pathogenic to likely pathogenic (2 variants), pathogenic to VUS (2), likely pathogenic to VUS (4), and VUS to benign (6).Conclusion: Considering that the clinical significance of CES data changed due to reclassification in almost half of the studied patients, we believe genetic variant-related data should be assessed at regular intervals, regardless of follow-up status in the clinic.
MoreTranslated text
Key words
Cancer,clinical exome sequencing,likely pathogenic/pathogenic variants
求助PDF
上传PDF
View via Publisher
AI Read Science
AI Summary
AI Summary is the key point extracted automatically understanding the full text of the paper, including the background, methods, results, conclusions, icons and other key content, so that you can get the outline of the paper at a glance.
Example
Background
Key content
Introduction
Methods
Results
Related work
Fund
Key content
- Pretraining has recently greatly promoted the development of natural language processing (NLP)
- We show that M6 outperforms the baselines in multimodal downstream tasks, and the large M6 with 10 parameters can reach a better performance
- We propose a method called M6 that is able to process information of multiple modalities and perform both single-modal and cross-modal understanding and generation
- The model is scaled to large model with 10 billion parameters with sophisticated deployment, and the 10 -parameter M6-large is the largest pretrained model in Chinese
- Experimental results show that our proposed M6 outperforms the baseline in a number of downstream tasks concerning both single modality and multiple modalities We will continue the pretraining of extremely large models by increasing data to explore the limit of its performance
Upload PDF to Generate Summary
Must-Reading Tree
Example
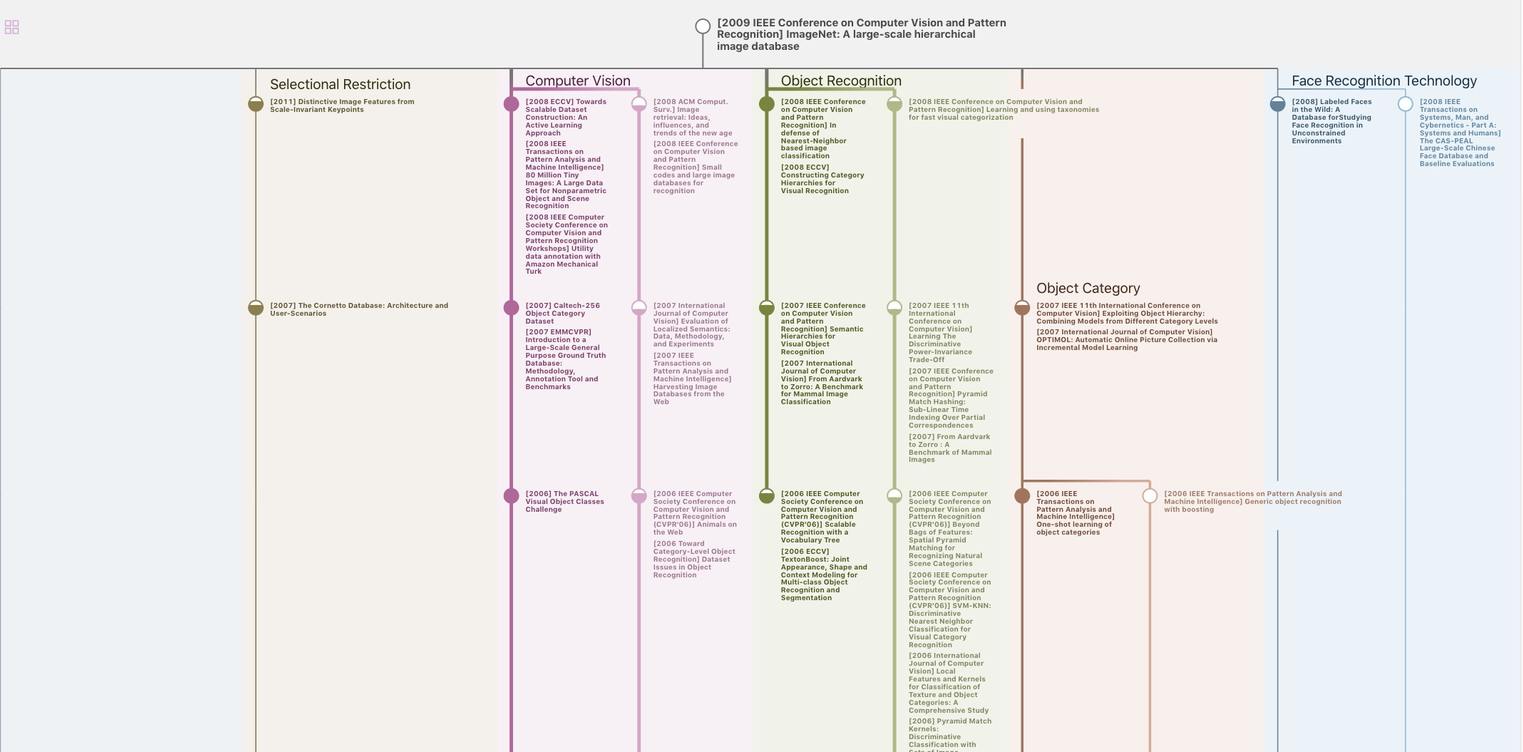
Generate MRT to find the research sequence of this paper
Related Papers
2015
被引用249 | 浏览
2015
被引用57 | 浏览
2015
被引用276 | 浏览
2017
被引用60 | 浏览
2016
被引用8000 | 浏览
2018
被引用57 | 浏览
2018
被引用676 | 浏览
2018
被引用91563 | 浏览
2020
被引用42 | 浏览
2020
被引用4423 | 浏览
2019
被引用618 | 浏览
2021
被引用171 | 浏览
Data Disclaimer
The page data are from open Internet sources, cooperative publishers and automatic analysis results through AI technology. We do not make any commitments and guarantees for the validity, accuracy, correctness, reliability, completeness and timeliness of the page data. If you have any questions, please contact us by email: report@aminer.cn
Chat Paper
GPU is busy, summary generation fails
Rerequest