181 Presystemic Elimination
Paul Erhardt,Kenneth Bachmann,Donald Birkett,Michael Boberg,Nicholas Bodor,Gordon Gibson,David Hawkins,Gabrielle Hawksworth,Jack Hinson,Daniel Koehler,Brian Kress,Amarjit Luniwal,Hiroshi Masumoto,Raymond Novak,Phillip Portoghese,Jeffrey Sarver,M. Teresa Serafini,Christopher Trabbic,Nico Vermeulen,Steven Wrighton IUPAC Standards Online(2022)
关键词
Drug Target Identification
AI 理解论文
溯源树
样例
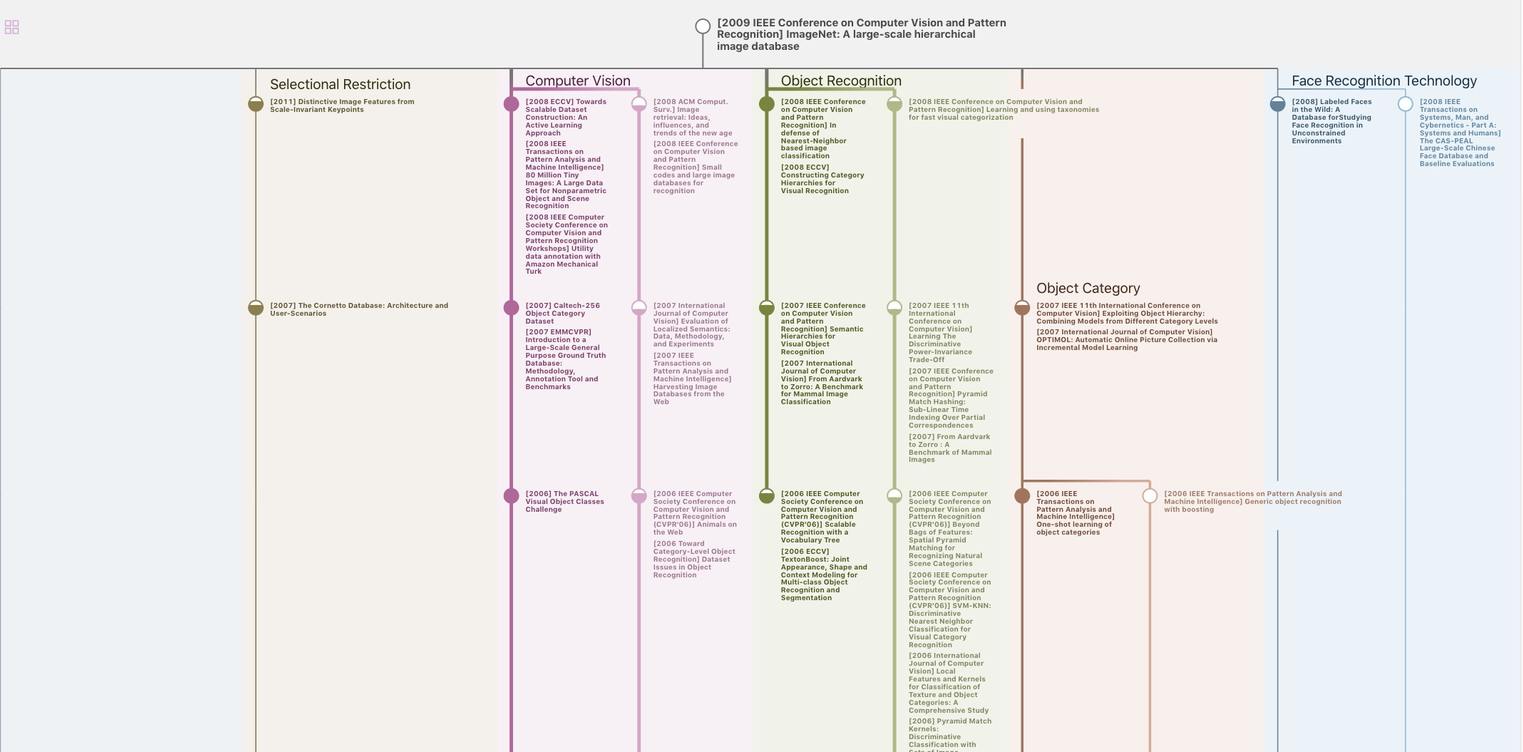