PolyGET: Accelerating Polymer Simulations by Accurate and Generalizable Forcefield with Equivariant Transformer
CoRR(2023)
摘要
Polymer simulation with both accuracy and efficiency is a challenging task. Machine learning (ML) forcefields have been developed to achieve both the accuracy of ab initio methods and the efficiency of empirical force fields. However, existing ML force fields are usually limited to single-molecule settings, and their simulations are not robust enough. In this paper, we present PolyGET, a new framework for Polymer Forcefields with Generalizable Equivariant Transformers. PolyGET is designed to capture complex quantum interactions between atoms and generalize across various polymer families, using a deep learning model called Equivariant Transformers. We propose a new training paradigm that focuses exclusively on optimizing forces, which is different from existing methods that jointly optimize forces and energy. This simple force-centric objective function avoids competing objectives between energy and forces, thereby allowing for learning a unified forcefield ML model over different polymer families. We evaluated PolyGET on a large-scale dataset of 24 distinct polymer types and demonstrated state-of-the-art performance in force accuracy and robust MD simulations. Furthermore, PolyGET can simulate large polymers with high fidelity to the reference ab initio DFT method while being able to generalize to unseen polymers.
更多查看译文
关键词
polymer simulations,forcefield,equivariant transformer
AI 理解论文
溯源树
样例
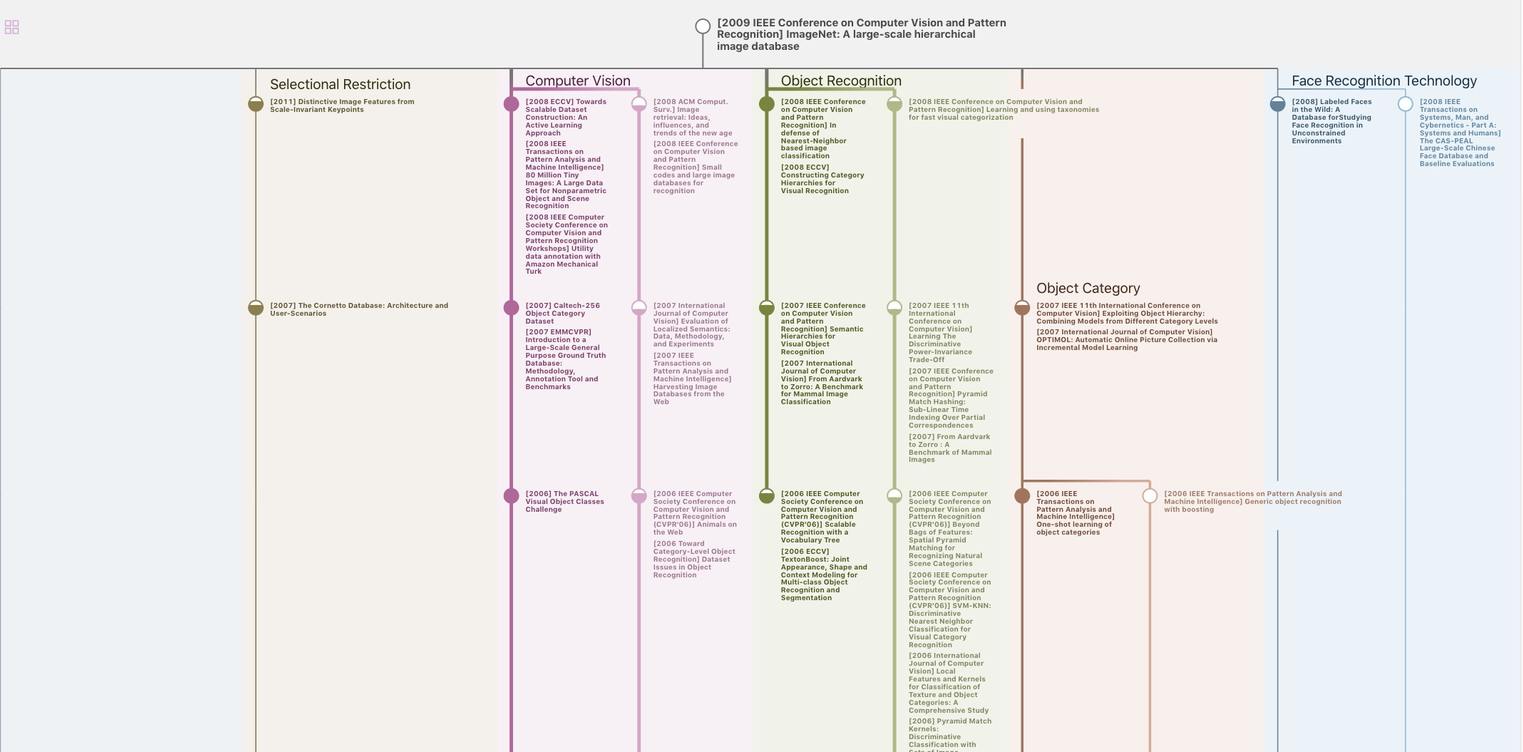
生成溯源树,研究论文发展脉络
Chat Paper
正在生成论文摘要