Highly Accurate and Efficient Prediction of Effective Thermal Conductivity of Sintered Silver Based on Deep Learning Method
International Journal of Heat and Mass Transfer/International journal of heat and mass transfer(2023)
摘要
Effective thermal conductivity (ETC) of sintered Ag is an essential parameter for its die-attach applica-tion in power electronics packaging, which could vary significantly with sintering conditions. The ex-isting ETC evaluation approaches are either of limited accuracy (analytical methods) or resource-and time-consuming (experiments and numerical simulations). In this study, deep learning method based on convolutional neural network (CNN) was first performed to predict the ETC of sintered Ag. The database was created with 6156 realistic microstructures of sintered Ag and corresponding reliable microstructural finite-element simulated ETC values (relative error of 5% with experimental results). Based on the ap-propriate design of CNN architecture, the trained model can accurately predict ETC values of the testing dataset samples with determination-coefficient (R 2 ) of 0.987, which significantly outperforms the con-ventional analytical (R 2 of 0.837) and machine learning methods (R 2 of 0.951). Besides, the prediction by CNN takes merely 0.14 s for an image, which is almost negligible. The methods presented here open a new way to achieve highly accurate and efficient prediction of ETC, which can help to prepare sintered-Ag die-attachment with desired ETC for power devices and also be applicable to investigate other effective -properties of sintered Ag.(c) 2022 Elsevier Ltd. All rights reserved.
更多查看译文
关键词
Sinterted Ag,Power electronics packaging,Effective thermal conductivity,Finite element modeling,Deep learning
AI 理解论文
溯源树
样例
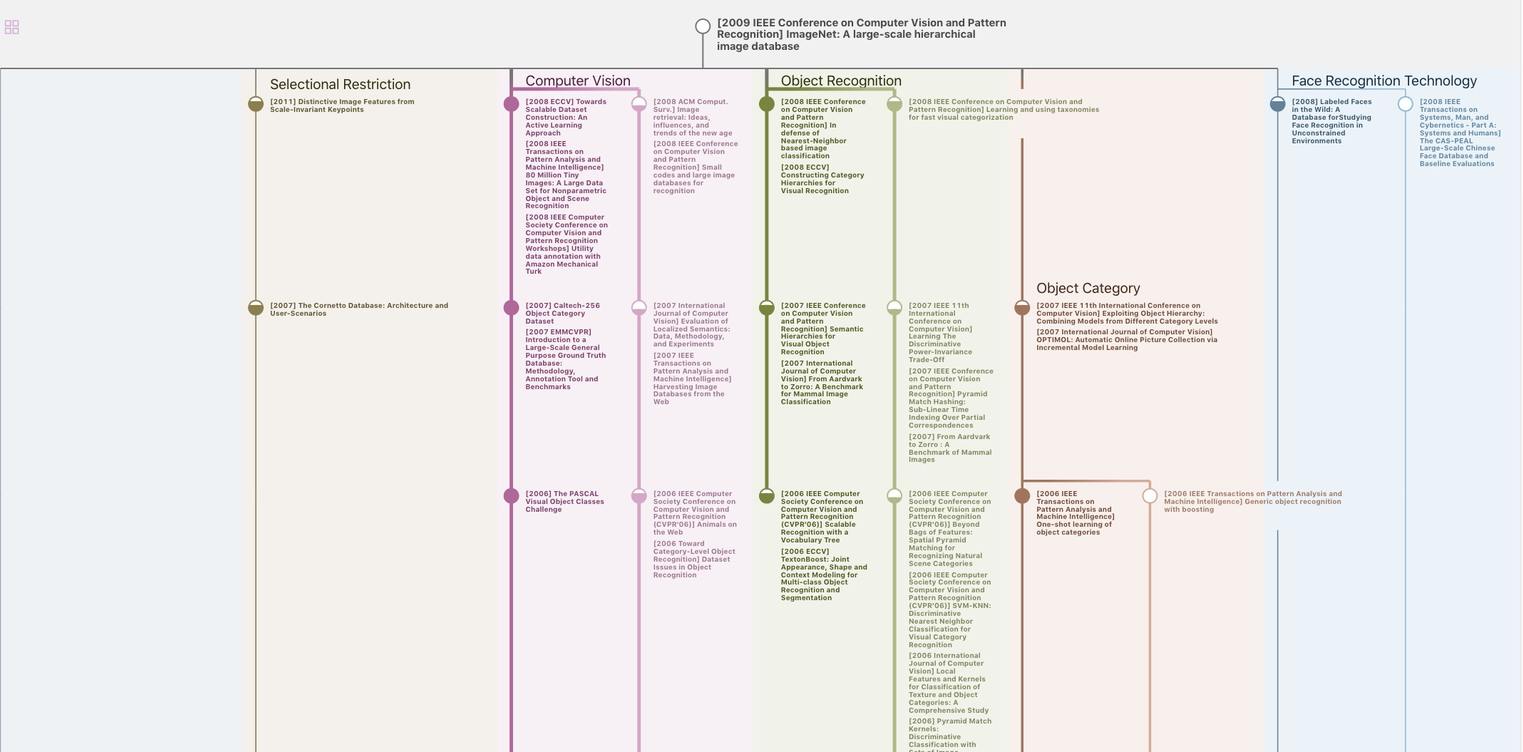
生成溯源树,研究论文发展脉络
Chat Paper
正在生成论文摘要