In‐Operando Lithium‐Ion Transport Tracking in an All‐Solid‐State Battery
Small(2022)
摘要
An all-solid-state battery is a secondary battery that is charged and discharged by the transport of lithium ions between positive and negative electrodes. To fully realize the significant benefits of this battery technology, for example, higher energy densities, faster charging times, and safer operation, it is essential to understand how lithium ions are transported and distributed in the battery during operation. However, as the third lightest element, methods for quantitatively analyzing lithium during operation of an all-solid-state device are limited such that real-time tracking of lithium transport has not yet been demonstrated. Here, the authors report that the transport of lithium ions in an all-solid-state battery is quantitatively tracked in near real time by utilizing a high-intensity thermal neutron source and lithium-6 as a tracer in a thermal neutron-induced nuclear reaction. Furthermore, the authors show that the lithium-ion migration mechanism and pathway through the solid electrolyte can be determined by in-operando tracking. From these results, the authors suggest that the development of all-solid-state batteries has entered a phase where further advances can be carried out while understanding the transport of lithium ions in the batteries.
更多查看译文
关键词
all-solid-state lithium batteries,lithium transport,lithium-6 tracer,neutron depth profiling,real-time tracking,thermal neutron-induced nuclear reaction
AI 理解论文
溯源树
样例
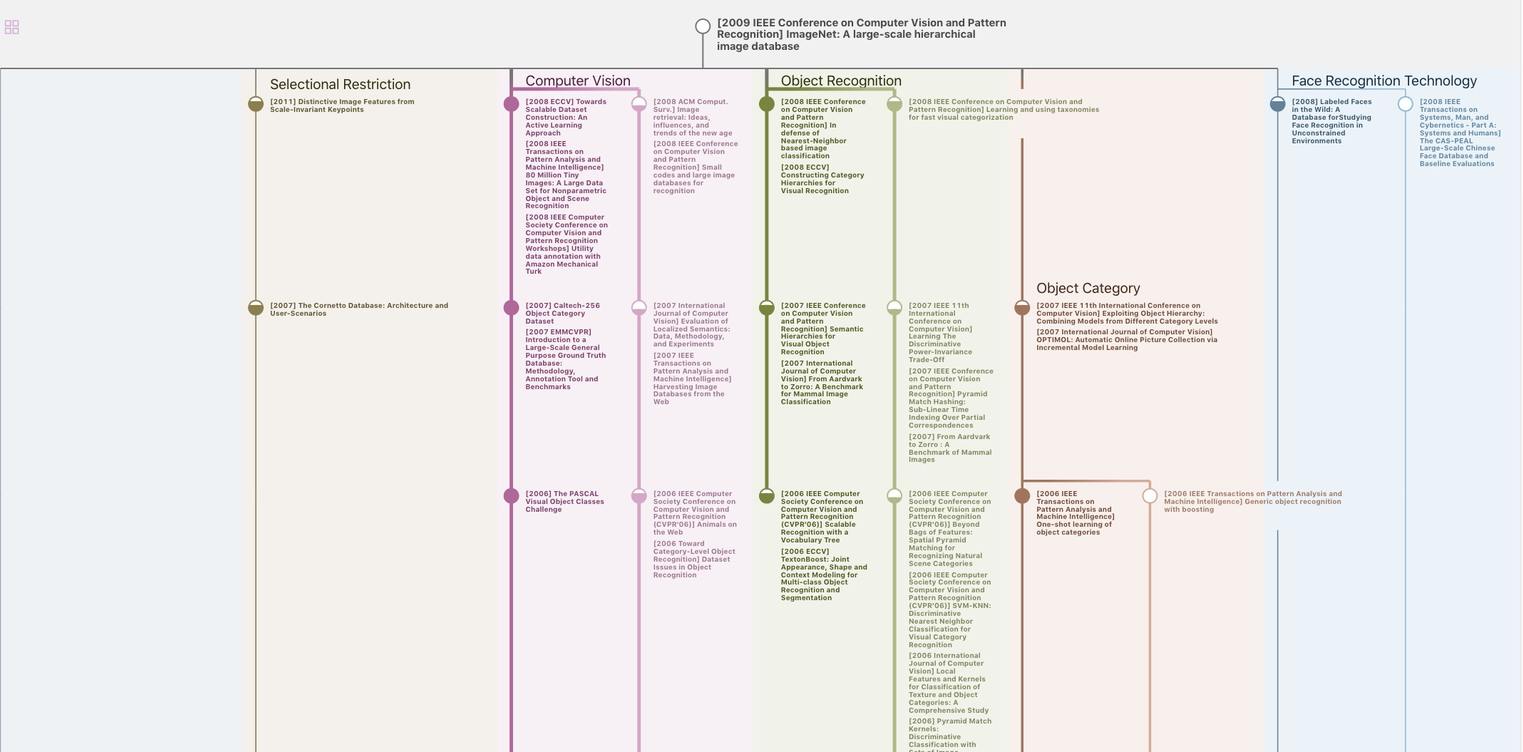
生成溯源树,研究论文发展脉络
Chat Paper
正在生成论文摘要