Sparsity enforced time-frequency decomposition in the Bayesian framework for bearing fault feature extraction under time-varying conditions
MECHANICAL SYSTEMS AND SIGNAL PROCESSING(2023)
摘要
Fault characteristic extraction of rolling bearings is essential for fault diagnosis. Rolling bearings are usually operated at changing speeds, and the nonstationary signals of the bearings are covered by the heavy background noise, making the extraction task of fault features very difficult. To address this issue, a robust fault characteristic extraction approach based on the time-frequency analysis under variable speed conditions is proposed in this paper. Firstly, the sparse property of the time-variant fault characteristics and low-rankness of background noise are explored and utilized in the time-frequency representation (TFR). Then, the sparse and the low-rank components are integrated into a hierarchical Bayesian model, and a random error term is considered to make the Bayesian model more robust. The Gibbs sampler is applied to extract the desired sparsity-enhanced component of the TFR in the Bayesian framework. Eventually, the time-frequency reassignment technique is adopted further to optimize the time-frequency resolution of the sparse component. Two simulated scenarios and a real-data experiment are used to evaluate the suggested approach's performance. It turns out that the proposed approach is robust to noise and can extract the bearing time-varying fault features effectively.
更多查看译文
关键词
Rolling bearing fault diagnosis,Variable speed condition,Sparse time-frequency representation,Hierarchical Bayesian,Gibbs sampler
AI 理解论文
溯源树
样例
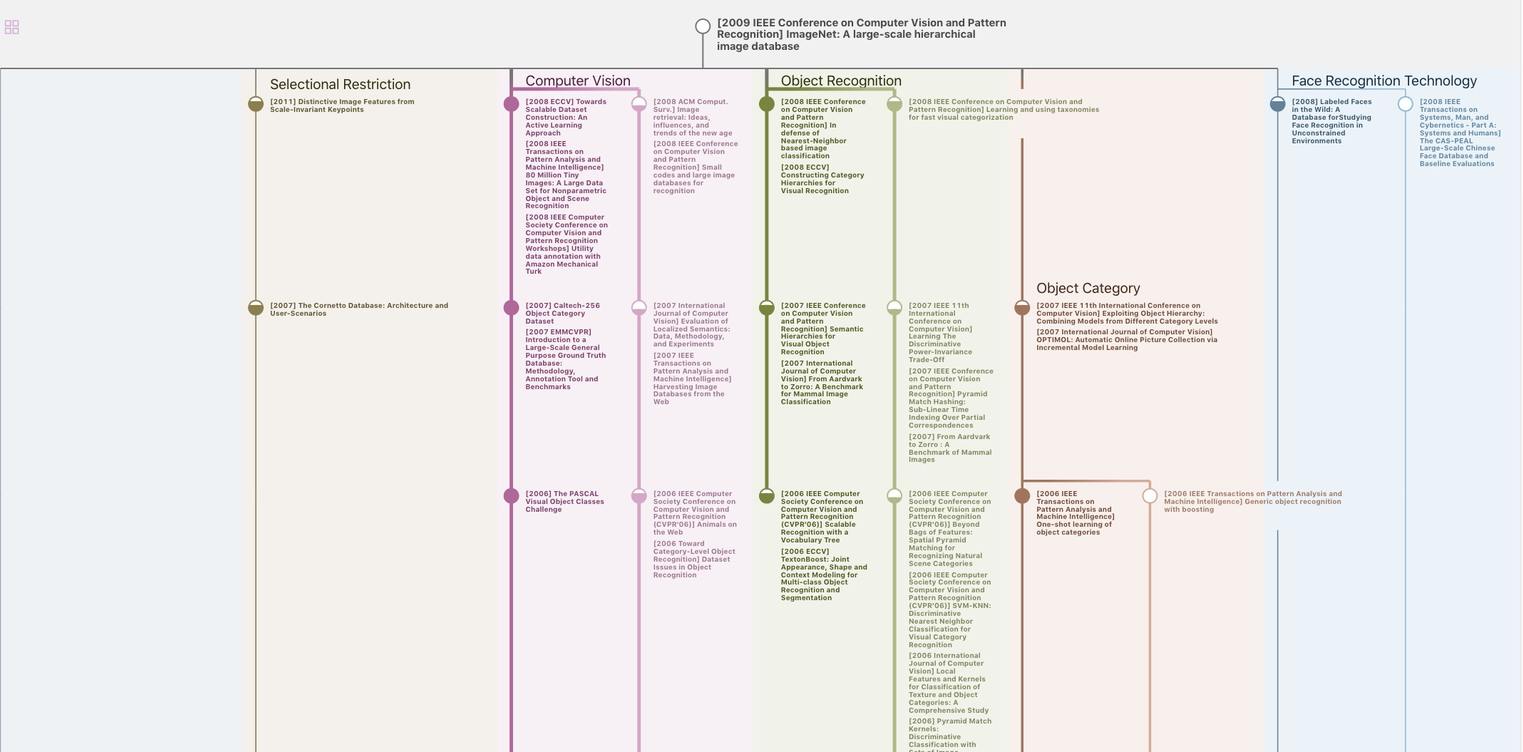
生成溯源树,研究论文发展脉络
Chat Paper
正在生成论文摘要