Dual Supervised Sampling Networks for Real-Time Segmentation of Cervical Cell Nucleus
Computational and Structural Biotechnology Journal(2022)
Abstract
The morphology of the cervical cell nucleus is the most important consideration for pathological cell identification. And a precise segmentation of the cervical cell nucleus determines the performance of the final classification for most traditional algorithms and even some deep learning-based algorithms. Many deep learning-based methods can accurately segment cervical cell nuclei but will cost lots of time, especially when dealing with the whole-slide image (WSI) of tens of thousands of cells. To address this challenge, we propose a dual-supervised sampling network structure, in which a supervised-down sampling module uses compressed images instead of original images for cell nucleus segmentation, and a boundary detection network is introduced to supervise the up-sampling process of the decoding layer for accurate segmentation. This strategy dramatically reduces the convolution calculation in image feature extraction and ensures segmentation accuracy. Experimental results on various cervical cell datasets demonstrate that compared with UNet, the inference speed of the proposed network is increased by 5 times without losing segmentation accuracy. The codes and datasets are available at https://github.com/ldrunning/DSSNet.
MoreTranslated text
Key words
Segmentation,Cervical cell,Dual supervised sampling
求助PDF
上传PDF
View via Publisher
AI Read Science
Must-Reading Tree
Example
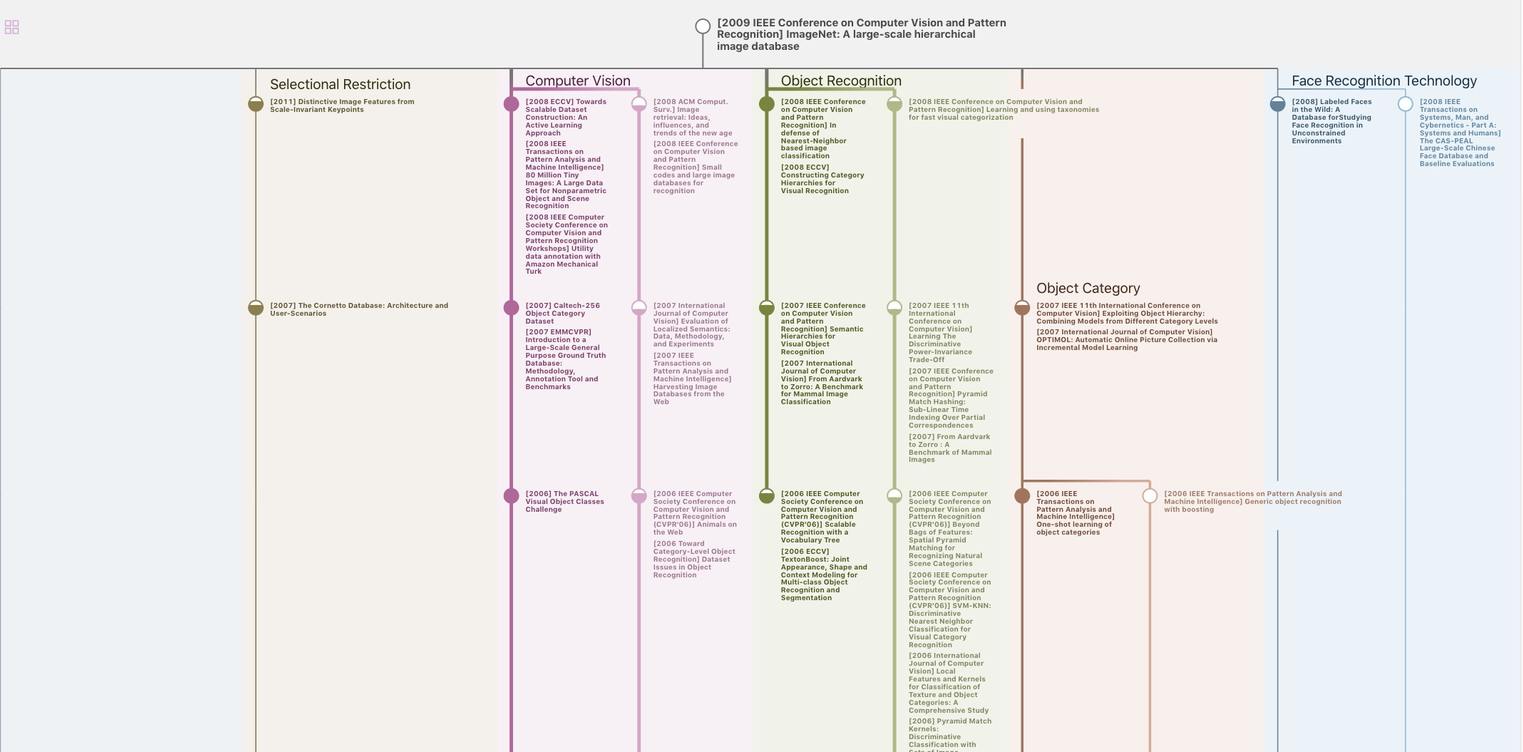
Generate MRT to find the research sequence of this paper
Related Papers
2015
被引用249 | 浏览
2015
被引用57 | 浏览
2015
被引用276 | 浏览
2017
被引用60 | 浏览
2016
被引用8000 | 浏览
2018
被引用57 | 浏览
2018
被引用676 | 浏览
2018
被引用91563 | 浏览
2020
被引用42 | 浏览
2020
被引用4423 | 浏览
2019
被引用618 | 浏览
2021
被引用171 | 浏览
Data Disclaimer
The page data are from open Internet sources, cooperative publishers and automatic analysis results through AI technology. We do not make any commitments and guarantees for the validity, accuracy, correctness, reliability, completeness and timeliness of the page data. If you have any questions, please contact us by email: report@aminer.cn
Chat Paper