紫草素对大鼠慢性皮肤溃疡创面愈合及新生血管形成的促进作用及其机制
Medical Journal of Chinese People's Liberation Army(2022)
Abstract
目的 探讨紫草素对大鼠慢性皮肤溃疡创面愈合及新生血管形成的促进作用及其可能机制.方法 健康雄性SD大鼠60只,其中50只构建慢性皮肤溃疡大鼠模型,造模成功40只,随机分为模型组、阳性对照组及紫草素低、高剂量组,每组10只,余10只设为对照组.紫草素低、高剂量组分别用4、8 mg/cm2紫草素混悬液均匀涂抹创面,阳性对照组用1890 U/cm2重组牛碱性成纤维细胞生长因子凝胶涂抹创面,模型组与对照组给予等体积生理盐水外敷.干预第3、7、14天观察大鼠皮肤溃疡创面愈合情况.干预结束后,取大鼠腹主动脉血和溃疡创面肉芽组织,HE染色观察溃疡创面肉芽组织病理学变化,免疫组化染色观察创面肉芽组织中新生血管形成情况;水解法检测创面肉芽组织中羟脯氨酸(HyP)含量,Western blotting检测创面肉芽组织中Notch1信号通路相关蛋白的表达;ELISA法检测血清炎性因子水平.结果 各组大鼠皮肤溃疡创面愈合率均随时间延长而增高,呈时间依赖性(P<0.05).与阳性对照组比较,紫草素低、高剂量组干预第3、7、14天的皮肤溃疡创面愈合率降低(P<0.05);与紫草素低剂量组比较,紫草素高剂量组干预第3、7、14天的皮肤溃疡创面愈合率增高(P<0.05).HE染色显示,模型组可见新生肉芽组织,伴有大量炎性细胞浸润;与模型组比较,紫草素低、高剂量组及阳性对照组可见陈旧性肉芽组织,炎性细胞浸润依次减轻.与阳性对照组比较,紫草素低、高剂量组溃疡创面肉芽组织IOD值降低(104725.45±2062.45 vs.197585.23±2478.42,P<0.05;149752.54±2441.86 vs.197585.23±2478.42,P<0.05),HyP含量降低[(3.62±0.12)μg/mg vs.(6.48±0.14)μg/mg,P<0.05;(4.94±0.15)μg/mg vs.(6.48±0.14)μg/mg,P<0.05];与紫草素低剂量组比较,紫草素高剂量组溃疡创面肉芽组织IOD值、HyP含量增高(P<0.05).Western blotting检测结果显示,与模型组比较,紫草素低、高剂量组溃疡创面肉芽组织中血管内皮细胞生长因子(VEGF)、转化生长因子-β1(TGF-β1)蛋白相对表达量增高,Notch1蛋白相对表达量降低(P<0.05);与紫草素低剂量组比较,紫草素高剂量组溃疡创面肉芽组织中VEGF、TGF-β1蛋白相对表达量增高,Notch1蛋白相对表达量降低(P<0.05).ELISA检测结果显示,与模型组比较,阳性对照组及紫草素低、高剂量组大鼠血清IL-8、TNF-α水平降低(P<0.05);与阳性对照组比较,紫草素低、高剂量组大鼠血清IL-8、TNF-α水平增高(P<0.05);与紫草素低剂量组比较,紫草素高剂量组大鼠血清IL-8、TNF-α水平降低(P<0.05).结论 紫草素可能通过调节Notch1信号通路促进大鼠慢性皮肤溃疡创面愈合及新生血管的形成.
More求助PDF
上传PDF
View via Publisher
AI Read Science
AI Summary
AI Summary is the key point extracted automatically understanding the full text of the paper, including the background, methods, results, conclusions, icons and other key content, so that you can get the outline of the paper at a glance.
Example
Background
Key content
Introduction
Methods
Results
Related work
Fund
Key content
- Pretraining has recently greatly promoted the development of natural language processing (NLP)
- We show that M6 outperforms the baselines in multimodal downstream tasks, and the large M6 with 10 parameters can reach a better performance
- We propose a method called M6 that is able to process information of multiple modalities and perform both single-modal and cross-modal understanding and generation
- The model is scaled to large model with 10 billion parameters with sophisticated deployment, and the 10 -parameter M6-large is the largest pretrained model in Chinese
- Experimental results show that our proposed M6 outperforms the baseline in a number of downstream tasks concerning both single modality and multiple modalities We will continue the pretraining of extremely large models by increasing data to explore the limit of its performance
Upload PDF to Generate Summary
Must-Reading Tree
Example
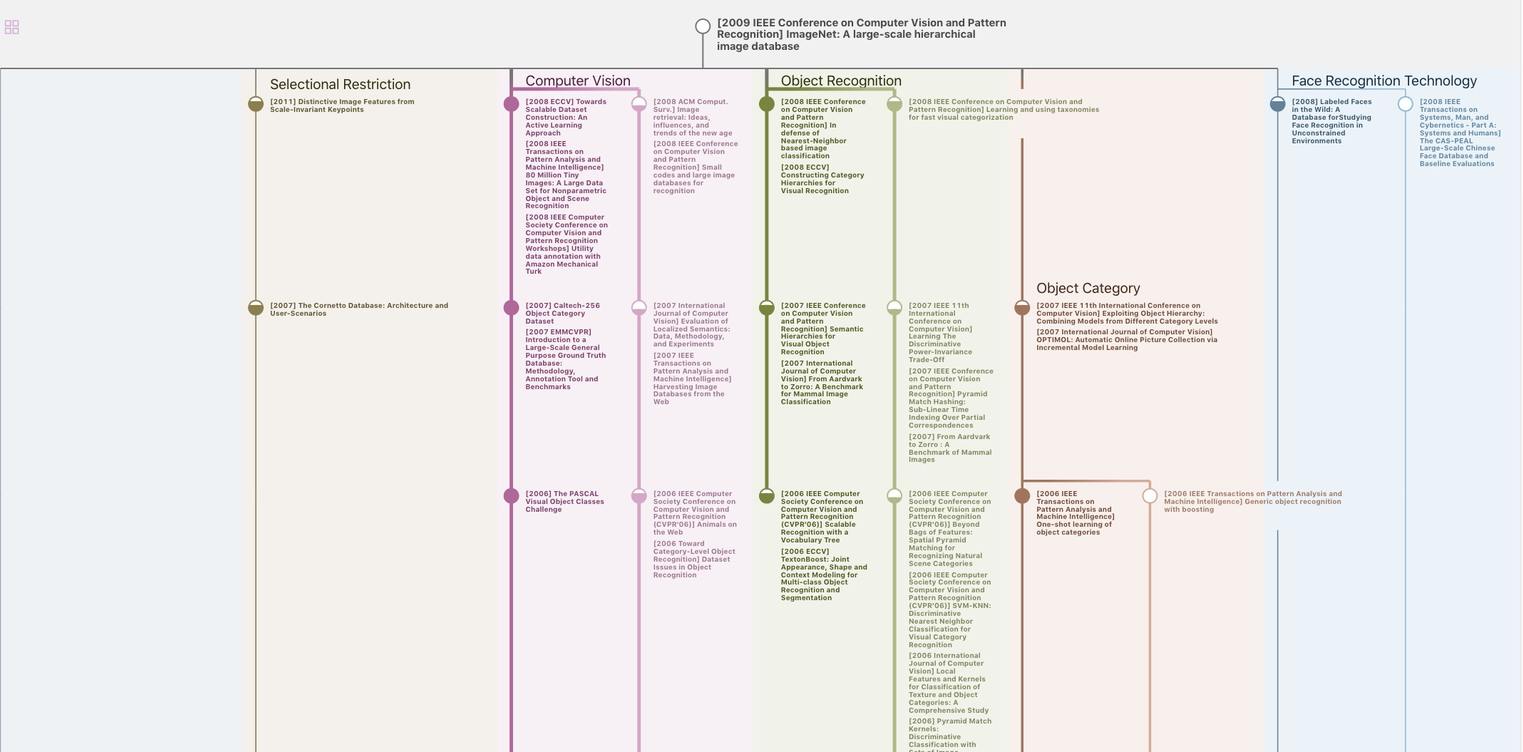
Generate MRT to find the research sequence of this paper
Related Papers
Chinese Traditional and Herbal Drugs 2022
被引用0
China Medical Abstracts of Dermatology 2023
被引用0
Data Disclaimer
The page data are from open Internet sources, cooperative publishers and automatic analysis results through AI technology. We do not make any commitments and guarantees for the validity, accuracy, correctness, reliability, completeness and timeliness of the page data. If you have any questions, please contact us by email: report@aminer.cn
Chat Paper