Dynamic Contrast Enhanced‐magnetic Resonance Imaging Radiomics Combined with a Hybrid Adaptive Neuro‐fuzzy Inference System‐particle Swarm Optimization Approach for Breast Tumour Classification
Expert Systems(2021)CCF CSCI 4区
Natl Tech Univ Athens | NHS Forth Valley | Univ Patras
Abstract
The authors propose a method for breast dynamic contrast enhanced‐magnetic resonance imaging classification by combining radiomic texture analysis with a hybrid adaptive neuro‐fuzzy inference system (ANFIS)‐particle swarm optimization (PSO) classifier. The fast discrete curvelet transform is utilized as a decomposition scheme in multiple scales. The mean and entropy features extracted from the produced scheme are used as texture descriptors. Principal component analysis (PCA) involves reduction of the dimensionality of the initial feature set. The transformed feature vector is subsequently introduced to a hybrid ANFIS‐PSO classifier. The average overall classification power of the proposed hybrid ANFIS‐PSO classifier is comparatively assessed to that obtained using several classifiers (ANFIS, linear discriminant analysis, Naïve Bayes, artificial neural networks, random forest and support vector machine) by using the 70 training‐30 testing data ratio. The comparison performed highlights the superiority of the proposed methodology, thus underlying the potential of ANFIS‐PSO for the breast cancer diagnosis with a classification accuracy of 94%.
MoreTranslated text
Key words
adaptive neuro-fuzzy inference system,breast cancer,dynamic contrast enhanced magnetic resonance imaging,fast discrete curvelet transform,particle swarm optimization,principal component analysis,radiomics,texture
求助PDF
上传PDF
View via Publisher
AI Read Science
AI Summary
AI Summary is the key point extracted automatically understanding the full text of the paper, including the background, methods, results, conclusions, icons and other key content, so that you can get the outline of the paper at a glance.
Example
Background
Key content
Introduction
Methods
Results
Related work
Fund
Key content
- Pretraining has recently greatly promoted the development of natural language processing (NLP)
- We show that M6 outperforms the baselines in multimodal downstream tasks, and the large M6 with 10 parameters can reach a better performance
- We propose a method called M6 that is able to process information of multiple modalities and perform both single-modal and cross-modal understanding and generation
- The model is scaled to large model with 10 billion parameters with sophisticated deployment, and the 10 -parameter M6-large is the largest pretrained model in Chinese
- Experimental results show that our proposed M6 outperforms the baseline in a number of downstream tasks concerning both single modality and multiple modalities We will continue the pretraining of extremely large models by increasing data to explore the limit of its performance
Upload PDF to Generate Summary
Must-Reading Tree
Example
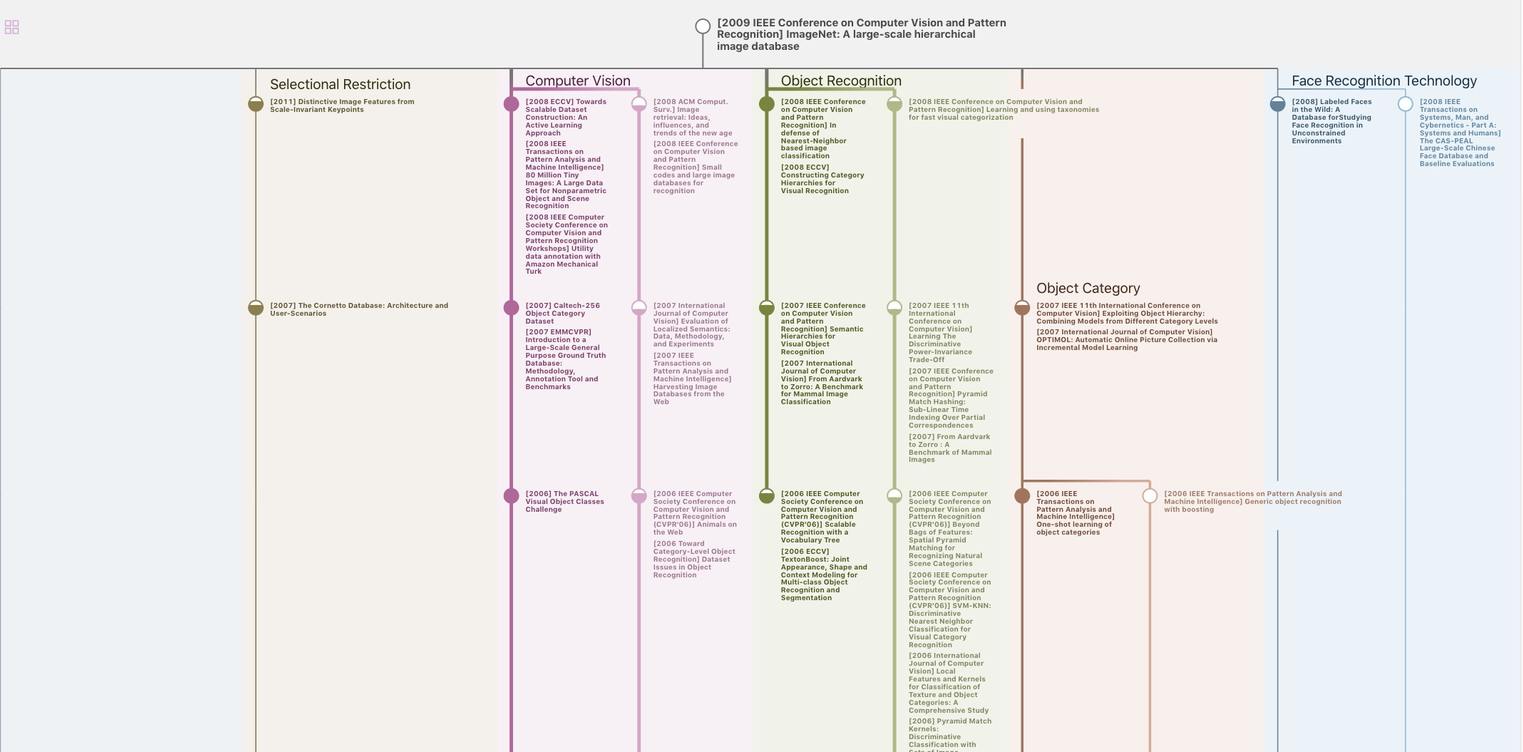
Generate MRT to find the research sequence of this paper
Related Papers
Machine Learning in Supply Chain: Prediction of Real-Time E-Order Arrivals Using ANFIS
International Journal of Systems Assurance Engineering and Management 2023
被引用2
IEEE ACCESS 2023
被引用7
Intelligent Identification and Classification of Diabetic Retinopathy Using Fuzzy Inference System
COMPUTER METHODS IN BIOMECHANICS AND BIOMEDICAL ENGINEERING-IMAGING AND VISUALIZATION 2023
被引用0
COVID-19 Detection from Chest X-ray Images Using CLAHE-YCrCb, LBP, and Machine Learning Algorithms
BMC BIOINFORMATICS 2024
被引用4
Data Disclaimer
The page data are from open Internet sources, cooperative publishers and automatic analysis results through AI technology. We do not make any commitments and guarantees for the validity, accuracy, correctness, reliability, completeness and timeliness of the page data. If you have any questions, please contact us by email: report@aminer.cn
Chat Paper
GPU is busy, summary generation fails
Rerequest