HDACs and the Epigenetic Plasticity of Cancer Cells: Target the Complexity.
Pharmacology and Therapeutics(2022)SCI 1区
Abstract
Cancer cells must adapt to the hostile conditions of the microenvironment in terms of nutrition, space, and immune system attack. Mutations of DNA are the drivers of the tumorigenic process, but mutations must be able to hijack cellular functions to sustain the spread of mutant genomes. Transcriptional control is a key function in this context and is controlled by the rearrangement of the epigenome. Unlike genomic mutations, the epigenome of cancer cells can in principle be reversed. The discovery of the first epigenetic drugs triggered a contaminating enthusiasm. Unfortunately, the complexity of the epigenetic machinery has frustrated this enthusiasm. To develop efficient patient-oriented epigenetic therapies, we need to better understand the nature of this complexity. In this review, we will discuss recent advances in understanding the contribution of HDACs to the maintenance of the transformed state and the rational for their selective targeting.
MoreTranslated text
Key words
HDACs,Epigenomics,Cancer,Therapy,Immune-therapy,Inhibitors,Acetylation
求助PDF
上传PDF
View via Publisher
AI Read Science
AI Summary
AI Summary is the key point extracted automatically understanding the full text of the paper, including the background, methods, results, conclusions, icons and other key content, so that you can get the outline of the paper at a glance.
Example
Background
Key content
Introduction
Methods
Results
Related work
Fund
Key content
- Pretraining has recently greatly promoted the development of natural language processing (NLP)
- We show that M6 outperforms the baselines in multimodal downstream tasks, and the large M6 with 10 parameters can reach a better performance
- We propose a method called M6 that is able to process information of multiple modalities and perform both single-modal and cross-modal understanding and generation
- The model is scaled to large model with 10 billion parameters with sophisticated deployment, and the 10 -parameter M6-large is the largest pretrained model in Chinese
- Experimental results show that our proposed M6 outperforms the baseline in a number of downstream tasks concerning both single modality and multiple modalities We will continue the pretraining of extremely large models by increasing data to explore the limit of its performance
Upload PDF to Generate Summary
Must-Reading Tree
Example
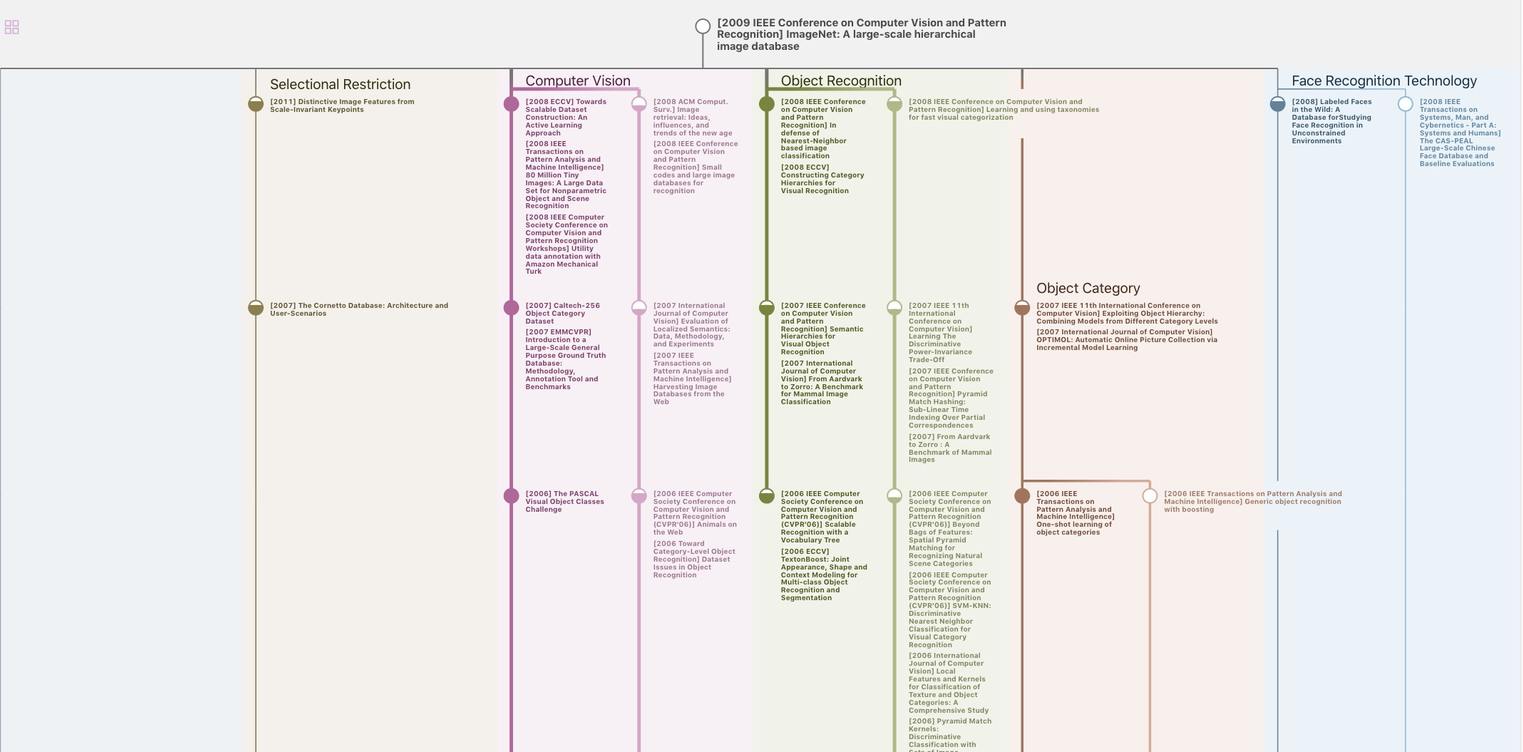
Generate MRT to find the research sequence of this paper
Related Papers
2004
被引用659 | 浏览
2011
被引用128 | 浏览
2002
被引用659 | 浏览
2011
被引用43 | 浏览
2001
被引用244 | 浏览
2013
被引用207 | 浏览
2016
被引用64 | 浏览
2015
被引用73 | 浏览
2017
被引用435 | 浏览
2017
被引用268 | 浏览
2018
被引用22 | 浏览
2016
被引用96 | 浏览
2019
被引用253 | 浏览
2020
被引用32 | 浏览
Data Disclaimer
The page data are from open Internet sources, cooperative publishers and automatic analysis results through AI technology. We do not make any commitments and guarantees for the validity, accuracy, correctness, reliability, completeness and timeliness of the page data. If you have any questions, please contact us by email: report@aminer.cn
Chat Paper
GPU is busy, summary generation fails
Rerequest