Construction of the gene expression subgroups of patients with coronary artery disease through bioinformatics approach
Mathematical biosciences and engineering : MBE(2021)SCI 4区
Abstract
Coronary artery disease (CAD) is a heterogeneous disease that has placed a heavy burden on public health due to its considerable morbidity, mortality and high costs. Better understanding of the genetic drivers and gene expression clustering behind CAD will be helpful for the development of genetic diagnosis of CAD patients. The transcriptome of 352 CAD patients and 263 normal controls were obtained from the Gene Expression Omnibus (GEO) database. We performed a modified unsupervised machine learning algorithm to group CAD patients. The relationship between gene modules obtained through weighted gene co-expression network analysis (WGCNA) and clinical features was identified by the Pearson correlation analysis. The annotation of gene modules and subgroups was done by the gene ontology (GO) and Kyoto Encyclopedia of Genes and Genomes (KEGG) pathway analysis. Three gene expression subgroups with the clustering score of greater than 0.75 were constructed. Subgroup I may experience coronary artery disease of an in-creased severity, while subgroup III is milder. Subgroup I was found to be closely related to the upregulation of the mitochondrial autophagy pathway, whereas the genes of subgroup II were shown to be related to the upregulation of the ribosome pathway. The high expression of APOE, NOS1 and NOS3 in the subgroup I suggested that the patients had more severe coronary artery disease. The construction of genetic subgroups of CAD patients has enabled clinicians to improve their understanding of CAD pathogenesis and provides potential tools for disease diagnosis, classification and assessment of prognosis.
MoreTranslated text
Key words
coronary artery disease, coronary heart disease, myocardial infarction, gene, RNA-Seq, subgroup
求助PDF
上传PDF
View via Publisher
AI Read Science
AI Summary
AI Summary is the key point extracted automatically understanding the full text of the paper, including the background, methods, results, conclusions, icons and other key content, so that you can get the outline of the paper at a glance.
Example
Background
Key content
Introduction
Methods
Results
Related work
Fund
Key content
- Pretraining has recently greatly promoted the development of natural language processing (NLP)
- We show that M6 outperforms the baselines in multimodal downstream tasks, and the large M6 with 10 parameters can reach a better performance
- We propose a method called M6 that is able to process information of multiple modalities and perform both single-modal and cross-modal understanding and generation
- The model is scaled to large model with 10 billion parameters with sophisticated deployment, and the 10 -parameter M6-large is the largest pretrained model in Chinese
- Experimental results show that our proposed M6 outperforms the baseline in a number of downstream tasks concerning both single modality and multiple modalities We will continue the pretraining of extremely large models by increasing data to explore the limit of its performance
Upload PDF to Generate Summary
Must-Reading Tree
Example
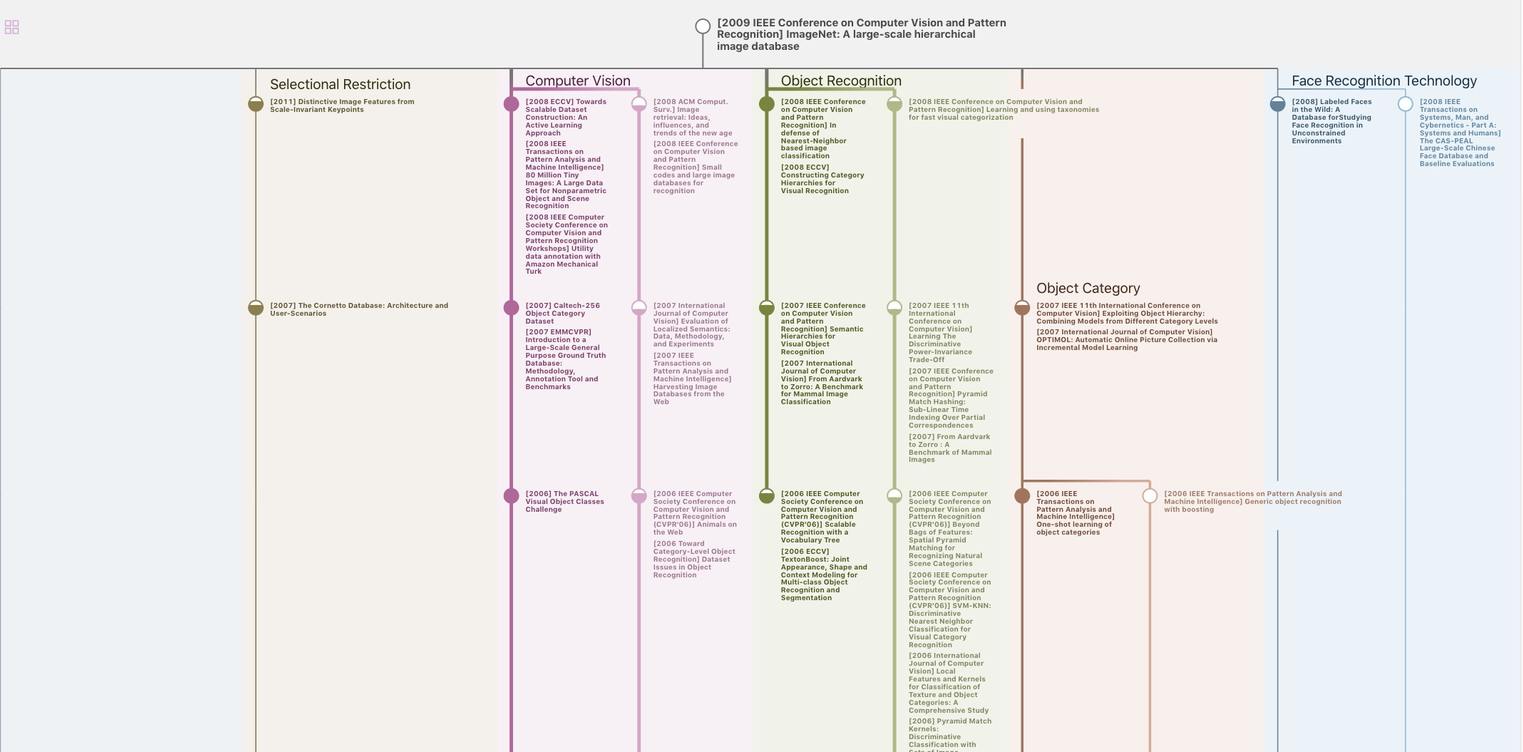
Generate MRT to find the research sequence of this paper
Related Papers
BMC Cardiovascular Disorders 2022
被引用5
Mediators of Inflammation 2022
被引用0
Frontiers in genetics 2022
被引用3
Data Disclaimer
The page data are from open Internet sources, cooperative publishers and automatic analysis results through AI technology. We do not make any commitments and guarantees for the validity, accuracy, correctness, reliability, completeness and timeliness of the page data. If you have any questions, please contact us by email: report@aminer.cn
Chat Paper