HSC Year 1 Cosmology Results with the Minimal Bias Method: HSC×BOSS Galaxy-Galaxy Weak Lensing and BOSS Galaxy Clustering
Physical Review D(2022)SCI 2区
Univ Tokyo | Natl Astron Observ Japan | Carnegie Mellon Univ | Hirosaki Univ | Kyoto Univ | Univ Geneva | Acad Sinica | Hosei Univ | Univ Calif Santa Cruz | Univ Sci & Technol China | Princeton Univ | Nagoya Univ | CALTECH | Univ Toronto
Abstract
We present cosmological parameter constraints from a blinded joint analysis of galaxy-galaxy weak lensing, $\Delta\!\Sigma(R)$, and projected correlation function, $w_\mathrm{p}(R)$, measured from the first-year HSC (HSC-Y1) data and SDSS spectroscopic galaxies over $0.15 0.75$ for the $\Delta\!\Sigma$ measurements, selected based on their photometric redshifts. For theoretical template, we use the minimal bias model for the cosmological clustering observables for the flat $\Lambda$CDM cosmological model. We compare the model predictions with the measurements in each redshift bin on large scales, $R>12$ and $8~h^{-1}\mathrm{Mpc}$ for $\Delta\!\Sigma(R)$ and $w_\mathrm{p}(R)$, respectively, where the perturbation theory-inspired model is valid. When we employ weak priors on cosmological parameters, without CMB information, we find $S_8=0.936^{+0.092}_{-0.086}$, $\sigma_8=0.85^{+0.16}_{-0.11}$, and $\Omega_\mathrm{m}=0.283^{+0.12}_{-0.035}$ for the flat $\Lambda$CDM model. Although the central value of $S_8$ appears to be larger than those inferred from other cosmological experiments, we find that the difference is consistent with expected differences due to sample variance, and our results are consistent with the other results to within the statistical uncertainties. (abriged)
MoreTranslated text
Key words
Cosmology
PDF
View via Publisher
AI Read Science
AI Summary
AI Summary is the key point extracted automatically understanding the full text of the paper, including the background, methods, results, conclusions, icons and other key content, so that you can get the outline of the paper at a glance.
Example
Background
Key content
Introduction
Methods
Results
Related work
Fund
Key content
- Pretraining has recently greatly promoted the development of natural language processing (NLP)
- We show that M6 outperforms the baselines in multimodal downstream tasks, and the large M6 with 10 parameters can reach a better performance
- We propose a method called M6 that is able to process information of multiple modalities and perform both single-modal and cross-modal understanding and generation
- The model is scaled to large model with 10 billion parameters with sophisticated deployment, and the 10 -parameter M6-large is the largest pretrained model in Chinese
- Experimental results show that our proposed M6 outperforms the baseline in a number of downstream tasks concerning both single modality and multiple modalities We will continue the pretraining of extremely large models by increasing data to explore the limit of its performance
Try using models to generate summary,it takes about 60s
Must-Reading Tree
Example
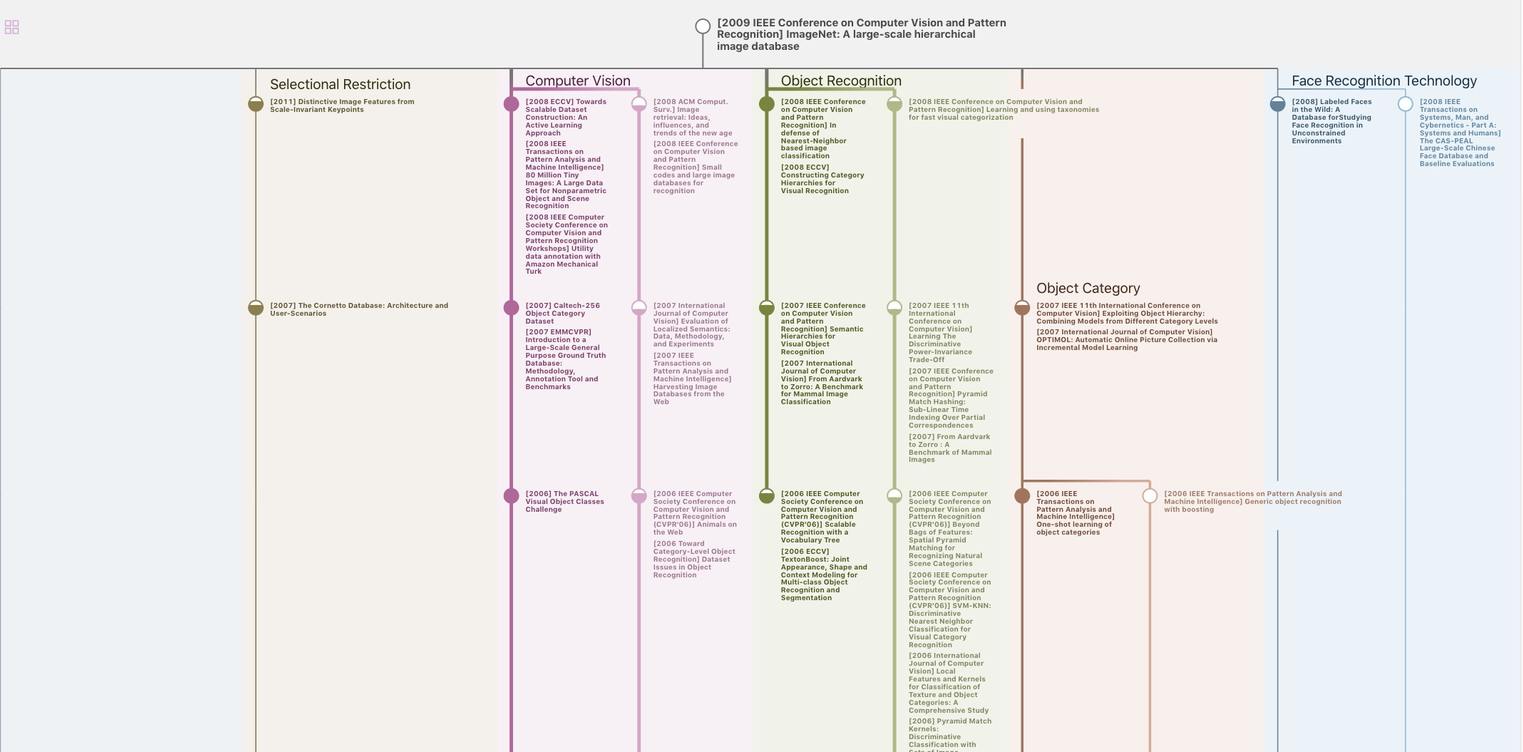
Generate MRT to find the research sequence of this paper
Related Papers
Annalen der Physik 2023
被引用1
MONTHLY NOTICES OF THE ROYAL ASTRONOMICAL SOCIETY 2023
被引用10
A Simulation-Based Inference Pipeline for Cosmic Shear with the Kilo-Degree Survey
MONTHLY NOTICES OF THE ROYAL ASTRONOMICAL SOCIETY 2023
被引用5
Hyper Suprime-Cam Year 3 Results: Cosmology from Cosmic Shear Two-point Correlation Functions
Physical review D/Physical review D 2023
被引用54
Physical review D/Physical review D 2023
被引用14
SCIENCE CHINA-PHYSICS MECHANICS & ASTRONOMY 2023
被引用4
ASTRONOMY & ASTROPHYSICS 2024
被引用1
Constraining Cosmology with the Gaia-unWISE Quasar Catalog and CMB Lensing: Structure Growth
JOURNAL OF COSMOLOGY AND ASTROPARTICLE PHYSICS 2023
被引用7
KiDS-SBI : Simulation-based Inference Analysis of KiDS-1000 Cosmic Shear
Astronomy & Astrophysics 2025
被引用0
ASTRONOMY & ASTROPHYSICS 2025
被引用0
Data Disclaimer
The page data are from open Internet sources, cooperative publishers and automatic analysis results through AI technology. We do not make any commitments and guarantees for the validity, accuracy, correctness, reliability, completeness and timeliness of the page data. If you have any questions, please contact us by email: report@aminer.cn
Chat Paper
GPU is busy, summary generation fails
Rerequest