Spatial Multi-Omic Map of Human Myocardial Infarction
Nature(2022)SCI 1区
RWTH Aachen University | Heidelberg University | Institute for Computational Genomics | Heart and Diabetes Center NRW | Heart and Diabetes Center | Department of Medicine | Division of Nephrology and Clinical Immunology | Cardiopathology | University Medical Center Utrecht | Department of Pathology | Department of Hematology | National Heart and Lung Institute | Institute of Cell and Tumor Biology
Abstract
Myocardial infarction is a leading cause of death worldwide1. Although advances have been made in acute treatment, an incomplete understanding of remodelling processes has limited the effectiveness of therapies to reduce late-stage mortality2. Here we generate an integrative high-resolution map of human cardiac remodelling after myocardial infarction using single-cell gene expression, chromatin accessibility and spatial transcriptomic profiling of multiple physiological zones at distinct time points in myocardium from patients with myocardial infarction and controls. Multi-modal data integration enabled us to evaluate cardiac cell-type compositions at increased resolution, yielding insights into changes of the cardiac transcriptome and epigenome through the identification of distinct tissue structures of injury, repair and remodelling. We identified and validated disease-specific cardiac cell states of major cell types and analysed them in their spatial context, evaluating their dependency on other cell types. Our data elucidate the molecular principles of human myocardial tissue organization, recapitulating a gradual cardiomyocyte and myeloid continuum following ischaemic injury. In sum, our study provides an integrative molecular map of human myocardial infarction, represents an essential reference for the field and paves the way for advanced mechanistic and therapeutic studies of cardiac disease.
MoreTranslated text
Key words
Data integration,Myocardial infarction,Transcriptomics,Science,Humanities and Social Sciences,multidisciplinary
PDF
View via Publisher
AI Read Science
AI Summary
AI Summary is the key point extracted automatically understanding the full text of the paper, including the background, methods, results, conclusions, icons and other key content, so that you can get the outline of the paper at a glance.
Example
Background
Key content
Introduction
Methods
Results
Related work
Fund
Key content
- Pretraining has recently greatly promoted the development of natural language processing (NLP)
- We show that M6 outperforms the baselines in multimodal downstream tasks, and the large M6 with 10 parameters can reach a better performance
- We propose a method called M6 that is able to process information of multiple modalities and perform both single-modal and cross-modal understanding and generation
- The model is scaled to large model with 10 billion parameters with sophisticated deployment, and the 10 -parameter M6-large is the largest pretrained model in Chinese
- Experimental results show that our proposed M6 outperforms the baseline in a number of downstream tasks concerning both single modality and multiple modalities We will continue the pretraining of extremely large models by increasing data to explore the limit of its performance
Try using models to generate summary,it takes about 60s
Must-Reading Tree
Example
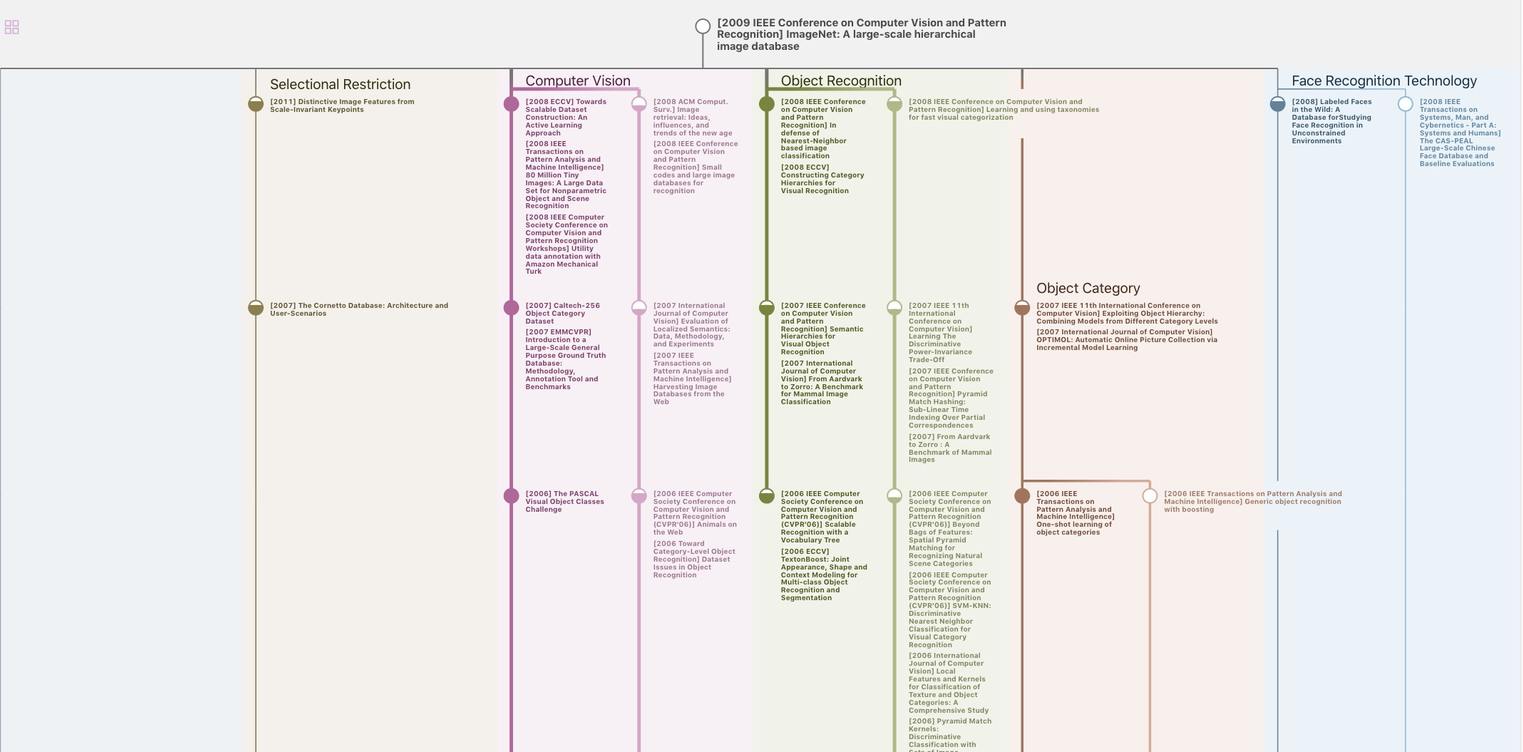
Generate MRT to find the research sequence of this paper
Related Papers
2005
被引用137 | 浏览
1999
被引用485 | 浏览
2011
被引用104 | 浏览
1988
被引用785 | 浏览
2008
被引用69 | 浏览
1995
被引用437 | 浏览
2016
被引用122 | 浏览
2016
被引用610 | 浏览
2018
被引用62 | 浏览
2018
被引用182 | 浏览
2018
被引用12 | 浏览
2018
被引用100 | 浏览
2016
被引用1411 | 浏览
2019
被引用78 | 浏览
2019
被引用55 | 浏览
2020
被引用245 | 浏览
2019
被引用194 | 浏览
Data Disclaimer
The page data are from open Internet sources, cooperative publishers and automatic analysis results through AI technology. We do not make any commitments and guarantees for the validity, accuracy, correctness, reliability, completeness and timeliness of the page data. If you have any questions, please contact us by email: report@aminer.cn
Chat Paper
去 AI 文献库 对话