Regularized Three-Dimensional Generative Adversarial Nets for Unsupervised Metal Artifact Reduction in Head and Neck CT Images
IEEE ACCESS(2020)
摘要
The reduction of metal artifacts in computed tomography (CT) images, specifically for strong artifacts generated from multiple metal objects, is a challenging issue in medical imaging research. Although there have been some studies on supervised metal artifact reduction through the learning of synthesized artifacts, it is difficult for simulated artifacts to cover the complexity of the real physical phenomena that may be observed in X-ray propagation. In this paper, we introduce metal artifact reduction methods based on an unsupervised volume-to-volume translation learned from clinical CT images. We construct three-dimensional adversarial nets with a regularized loss function designed for metal artifacts from multiple dental fillings. The results of experiments using a CT volume database of 361 patients demonstrate that the proposed framework has an outstanding capacity to reduce strong artifacts and to recover underlying missing voxels, while preserving the anatomical features of soft tissues and tooth structures from the original images.
更多查看译文
关键词
Computed tomography,Metals,Three-dimensional displays,Training,Databases,Surgery,Biomedical imaging,Computed tomography,generative adversarial network,metal artifact reduction,unsupervised image translation
AI 理解论文
溯源树
样例
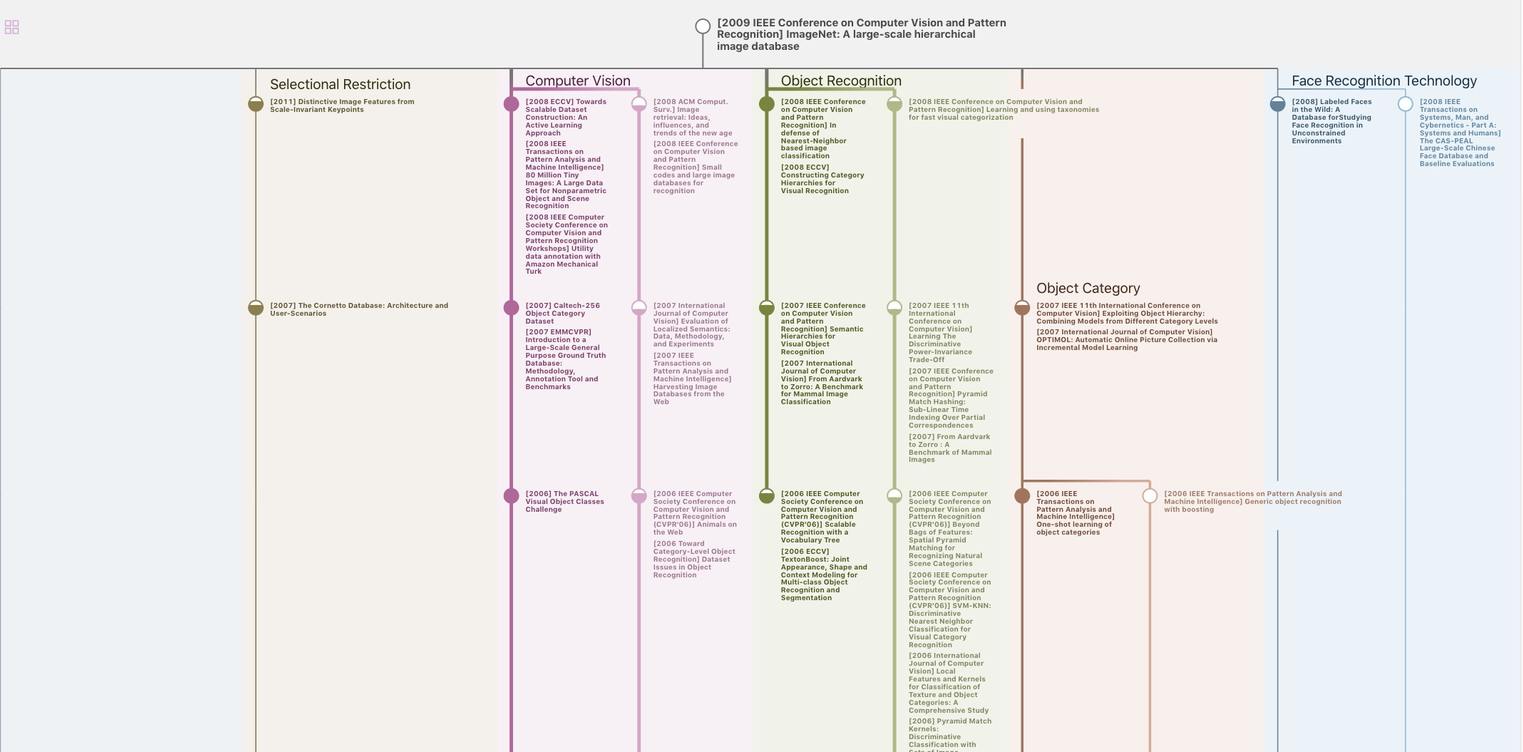
生成溯源树,研究论文发展脉络
Chat Paper
正在生成论文摘要