Non-invasive Diagnosis of Non-Alcoholic Steatohepatitis and Fibrosis with the Use of Omics and Supervised Learning: A Proof of Concept Study.
Metabolism: Clinical and Experimental(2019)
Beth Israel Deaconess Med Ctr | Aristotle Univ Thessaloniki | Brown Univ | Inst Salud Carlos III | 424 Gen Mil Hosp
Abstract
Background: Non-alcoholic fatty liver disease (NAFLD) affects 25-30% of the general population and is characterized by the presence of non-alcoholic fatty liver (NAFL) that can progress to non-alcoholic steatohepatitis (NASH), liver fibrosis and cirrhosis leading to hepatocellular carcinoma. To date, liver biopsy is the gold standard for the diagnosis of NASH and for staging liver fibrosis. This study aimed to train models for the non-invasive diagnosis of NASH and liver fibrosis based on measurements of lipids, glycans and biochemical parameters in peripheral blood and with the use of different machine learning methods. Methods: We performed a lipidomic, glycomic and free fatty acid analysis in serum samples of 49 healthy subjects and 31 patients with biopsy-proven NAFLD (15 with NAFL and 16 with NASH). The data from the above measurements combined with measurements of 4 hormonal parameters were analyzed with two different platforms and five different machine learning tools. Results: 365 lipids, 61 glycans and 23 fatty adds were identified with mass-spectrometry and liquid chromatography. Robust differences in the concentrations of specific lipid species were observed between healthy, NMI. and NASH subjects. One-vs-Rest (OvR) support vector machine (SVM) models with recursive feature elimination (RFE) including 29 lipids or combining lipids with glycans and/or hormones (20 or 10 variables total) could differentiate with very high accuracy (up to 90%) between the three conditions. In an exploratory analysis, a model consisting of 10 lipid species could robustly discriminate between the presence of liver fibrosis or not (98% accuracy). Conclusion: We propose novel models utilizing lipids, hormones and glycans that can diagnose with high accuracy the presence of NASH, NAFL or healthy status. Additionally, we report a combination of lipids that can diagnose the presence of liver fibrosis. Both models should be further trained prospectively and validated in large independent cohorts. (C) 2019 Elsevier Inc. All rights reserved.
MoreTranslated text
Key words
Non-alcoholic fatty liver disease,Non-alcoholic steatohepatitis,Liver fibrosis,Metabolomics,Lipidomics,Glycomics,Machine learning,Non-invasive
PDF
View via Publisher
AI Read Science
AI Summary
AI Summary is the key point extracted automatically understanding the full text of the paper, including the background, methods, results, conclusions, icons and other key content, so that you can get the outline of the paper at a glance.
Example
Background
Key content
Introduction
Methods
Results
Related work
Fund
Key content
- Pretraining has recently greatly promoted the development of natural language processing (NLP)
- We show that M6 outperforms the baselines in multimodal downstream tasks, and the large M6 with 10 parameters can reach a better performance
- We propose a method called M6 that is able to process information of multiple modalities and perform both single-modal and cross-modal understanding and generation
- The model is scaled to large model with 10 billion parameters with sophisticated deployment, and the 10 -parameter M6-large is the largest pretrained model in Chinese
- Experimental results show that our proposed M6 outperforms the baseline in a number of downstream tasks concerning both single modality and multiple modalities We will continue the pretraining of extremely large models by increasing data to explore the limit of its performance
Try using models to generate summary,it takes about 60s
Must-Reading Tree
Example
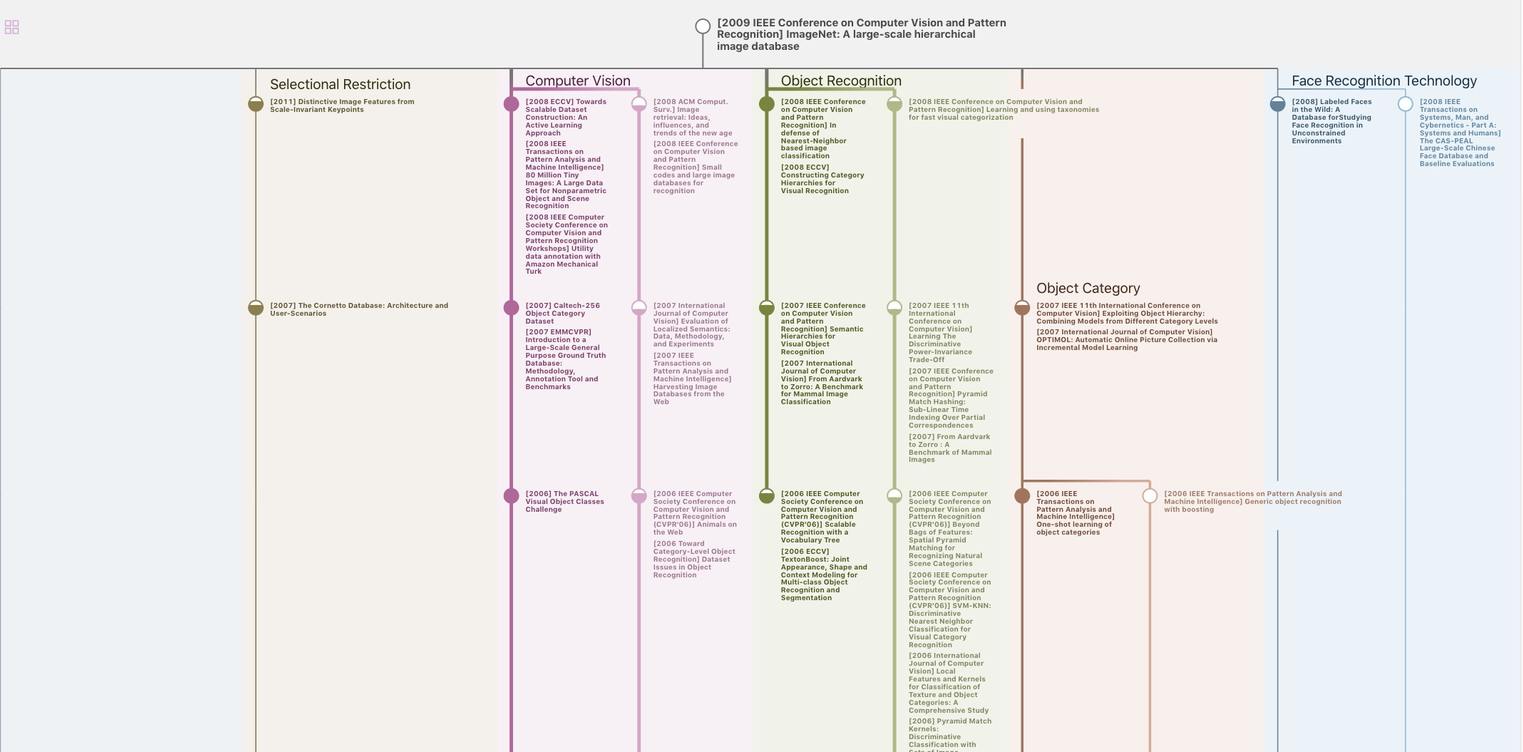
Generate MRT to find the research sequence of this paper
Related Papers
2006
被引用1969 | 浏览
1996
被引用52 | 浏览
2004
被引用86 | 浏览
2002
被引用56 | 浏览
2009
被引用517 | 浏览
2011
被引用1160 | 浏览
2011
被引用39 | 浏览
2014
被引用237 | 浏览
2014
被引用26 | 浏览
2015
被引用8 | 浏览
2018
被引用7425 | 浏览
2017
被引用63 | 浏览
2018
被引用31 | 浏览
2018
被引用30 | 浏览
2018
被引用467 | 浏览
2018
被引用10 | 浏览
2018
被引用215 | 浏览
2019
被引用899 | 浏览
2019
被引用76 | 浏览
Data Disclaimer
The page data are from open Internet sources, cooperative publishers and automatic analysis results through AI technology. We do not make any commitments and guarantees for the validity, accuracy, correctness, reliability, completeness and timeliness of the page data. If you have any questions, please contact us by email: report@aminer.cn
Chat Paper
Summary is being generated by the instructions you defined