Routing Entanglement in the Quantum Internet
Department of Electrical Engineering and Computer Science | Quantum Information Processing group | College of Information and Computer Sciences | School of Engineering and Applied Science | Departments of Applied Physics and Physics | Advanced Networking Systems
Abstract
Remote quantum entanglement can enable numerous applications including distributed quantum computation, secure communication, and precision sensing. We consider how a quantum network-nodes equipped with limited quantum processing capabilities connected via lossy optical links-can distribute high-rate entanglement simultaneously between multiple pairs of users. We develop protocols for such quantum "repeater" nodes, which enable a pair of users to achieve large gains in entanglement rates over using a linear chain of quantum repeaters, by exploiting the diversity of multiple paths in the network. Additionally, we develop repeater protocols that enable multiple user pairs to generate entanglement simultaneously at rates that can far exceed what is possible with repeaters time sharing among assisting individual entanglement flows. Our results suggest that the early-stage development of quantum memories with short coherence times and implementations of probabilistic Bell-state measurements can have a much more profound impact on quantum networks than may be apparent from analyzing linear repeater chains. This framework should spur the development of a general quantum network theory, bringing together quantum memory physics, quantum information theory, quantum error correction, and computer network theory.
MoreTranslated text
Key words
Fibre optics and optical communications,Quantum information,Physics,general,Quantum Physics,Quantum Information Technology,Spintronics,Quantum Computing,Quantum Field Theories,String Theory,Classical and Quantum Gravitation,Relativity Theory
PDF
View via Publisher
AI Read Science
AI Summary
AI Summary is the key point extracted automatically understanding the full text of the paper, including the background, methods, results, conclusions, icons and other key content, so that you can get the outline of the paper at a glance.
Example
Background
Key content
Introduction
Methods
Results
Related work
Fund
Key content
- Pretraining has recently greatly promoted the development of natural language processing (NLP)
- We show that M6 outperforms the baselines in multimodal downstream tasks, and the large M6 with 10 parameters can reach a better performance
- We propose a method called M6 that is able to process information of multiple modalities and perform both single-modal and cross-modal understanding and generation
- The model is scaled to large model with 10 billion parameters with sophisticated deployment, and the 10 -parameter M6-large is the largest pretrained model in Chinese
- Experimental results show that our proposed M6 outperforms the baseline in a number of downstream tasks concerning both single modality and multiple modalities We will continue the pretraining of extremely large models by increasing data to explore the limit of its performance
Try using models to generate summary,it takes about 60s
Must-Reading Tree
Example
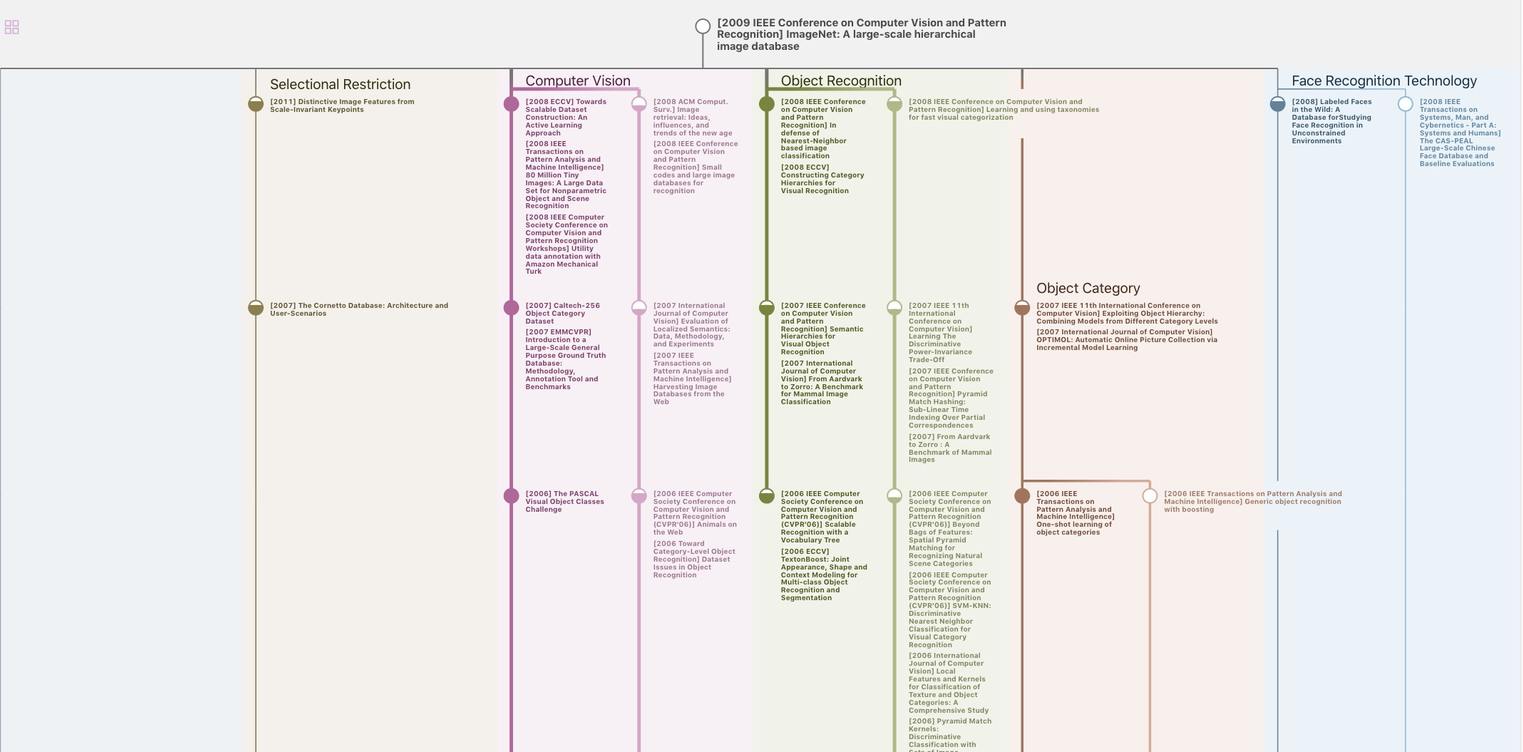
Generate MRT to find the research sequence of this paper
Related Papers
2019
被引用7 | 浏览
2021
被引用18 | 浏览
2021
被引用19 | 浏览
2021
被引用42 | 浏览
2022
被引用14 | 浏览
2022
被引用4 | 浏览
2022
被引用28 | 浏览
2022
被引用15 | 浏览
2022
被引用7 | 浏览
Data Disclaimer
The page data are from open Internet sources, cooperative publishers and automatic analysis results through AI technology. We do not make any commitments and guarantees for the validity, accuracy, correctness, reliability, completeness and timeliness of the page data. If you have any questions, please contact us by email: report@aminer.cn
Chat Paper
去 AI 文献库 对话