Predicting Lymph Node Metastasis in Head and Neck Cancer by Combining Many-objective Radiomics and 3-Dimensioal Convolutional Neural Network Through Evidential Reasoning.
International Conference of the IEEE Engineering in Medicine and Biology Society (EMBC)(2018)
Univ Texas Southwestern Med Ctr Dallas | Sichuan Univ
Abstract
Lymph node metastasis (LNM) is a significant prognostic factor in patients with head and neck cancer, and the ability to predict it accurately is essential for treatment optimization. PET and CT imaging are routinely used for LNM identification. However, uncertainties of LNM always exist especially for small size or reactive nodes. Radiomics and deep learning are the two preferred imaging-based strategies for node malignancy prediction. Radiomics models are built based on handcrafted features, and deep learning can learn the features automatically. We proposed a hybrid predictive model that combines many-objective radiomics (MO-radiomics) and 3-dimensional convolutional neural network (3D-CNN) through evidential reasoning (ER) approach. To build a more reliable model, we proposed a new many-objective radiomics model. Meanwhile, we designed a 3D-CNN that fully utilizes spatial contextual information. Finally, the outputs were fused through the ER approach. To study the predictability of the two modalities, three models were built for PET, CT, and PET& CT. The results showed that the model performed best when the two modalities were combined. Moreover, we showed that the quantitative results obtained from the hybrid model were better than those obtained from MO-radiomics and 3D-CNN.
MoreTranslated text
Key words
radiomics,convolutional neural network,evidential reasoning,lymph node metastasis,head and neck cancer
PDF
View via Publisher
AI Read Science
AI Summary
AI Summary is the key point extracted automatically understanding the full text of the paper, including the background, methods, results, conclusions, icons and other key content, so that you can get the outline of the paper at a glance.
Example
Background
Key content
Introduction
Methods
Results
Related work
Fund
Key content
- Pretraining has recently greatly promoted the development of natural language processing (NLP)
- We show that M6 outperforms the baselines in multimodal downstream tasks, and the large M6 with 10 parameters can reach a better performance
- We propose a method called M6 that is able to process information of multiple modalities and perform both single-modal and cross-modal understanding and generation
- The model is scaled to large model with 10 billion parameters with sophisticated deployment, and the 10 -parameter M6-large is the largest pretrained model in Chinese
- Experimental results show that our proposed M6 outperforms the baseline in a number of downstream tasks concerning both single modality and multiple modalities We will continue the pretraining of extremely large models by increasing data to explore the limit of its performance
Try using models to generate summary,it takes about 60s
Must-Reading Tree
Example
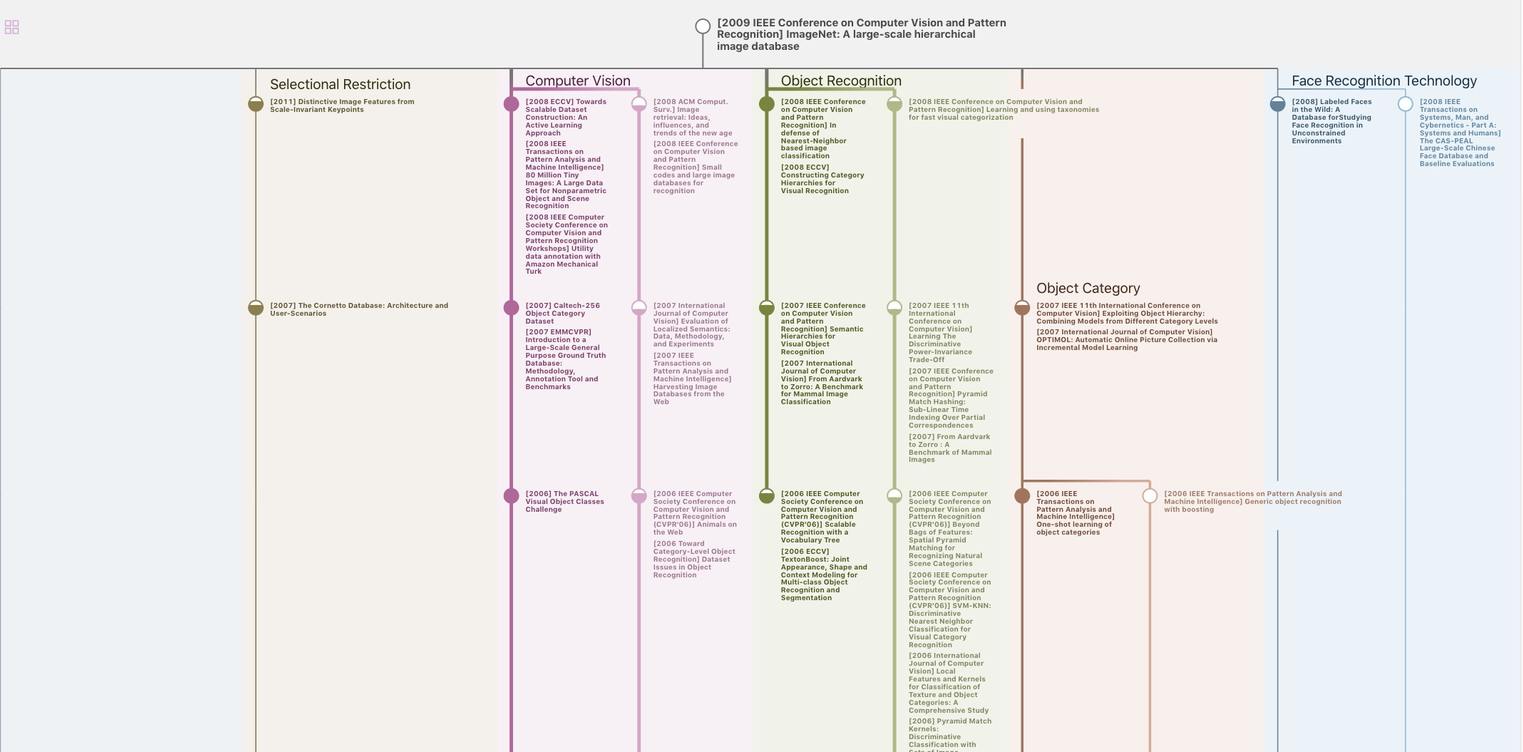
Generate MRT to find the research sequence of this paper
Related Papers
2001
被引用2978 | 浏览
2002
被引用1145 | 浏览
2015
被引用706 | 浏览
2016
被引用257 | 浏览
2016
被引用1711 | 浏览
2017
被引用296 | 浏览
2018
被引用34 | 浏览
2016
被引用582 | 浏览
IEEE Transactions on Systems, Man, and Cybernetics 2002
被引用670 | 浏览
2015
被引用598 | 浏览
2020
被引用1117 | 浏览
Data Disclaimer
The page data are from open Internet sources, cooperative publishers and automatic analysis results through AI technology. We do not make any commitments and guarantees for the validity, accuracy, correctness, reliability, completeness and timeliness of the page data. If you have any questions, please contact us by email: report@aminer.cn
Chat Paper
去 AI 文献库 对话