基本信息
浏览量:37
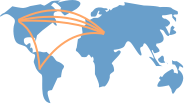
个人简介
Data and Information Visualization
Main research interest is in the area of scientific data visualization. Initially our focus was in looking at ways of controlling mapping artifacts arising from various ad-hoc and ill-defined assumptions in existing data visualization tools. One possible solution is to treat data modeling function (interpolation and approximation) in the mapping techniques as a separate process, independent of the mapping process itself. Toward this end, an alternative model named as Model Centered Approach (MCA) has been proposed. Further research on MCA has extended it usage into internet-based visualization system. In this case, the compact representation of the modeling function has made it more useful for internet-based visualization – the model that will generate the data is send to the peer hosts, rather than the data themselves which are often huge in size.
Recent work concentrates on the development of algorithm to optimize 3D surface(s) reconstruction from a series contour’s slices. In this work we optimizes the reconstruction time while preserving the output at an acceptable level i.e. no clear surface artifacts likes holes. Good balances between reconstruction time and surface quality for a series of dataset have been reported by a Master thesis completed recently. We are now looking for a more thorough study on the slice reduction method, at the same time research on the reconstruction of surfaces from sparse contour slices is high in our research agenda.
Current work in this area is focusing on the extraction of feature lines known as crest-lines from 3D medical dataset. The aim is to use the extracted feature lines in detecting important landmarks on a craniofacial dataset. Automating this landmarks identification and placement is crucial to reduce the tedious and error-prone manual inspection. Feature lines based on crest points (also known as extremal points) are invariant to common transformation, and thus they are good for features registration and matching between objects.
Research Interests :
Network Security
Primary focus is on improving existing IDS/IPS solutions by enriching and enhancing alerts they produced with verifiable and quantifiable data quality metrics. Initial work in this area was the establishment of a model called Intrusion Alert Quality Framework (IAQF). It main purpose is to assist network and security engineers in making effective and efficient decisions about the security status of ICT assets under their control. With IAQF we have enriched the alerts generated by IDS/IPS with data quality metrics (alive-correctness, os-correctness, service-correctness, etc) and quantify them in term of score. These quality metrics are verified using contextual information (host’s profiles – os, patch level, application version, etc) from the network environment being monitored in an iterative fashion following a typical cycle of TDQM process. The verified and enriched alerts are finally encoded in the standard format (Intrusion Detection Message Exchange Format or IDMEF) widely used by IDS/IPS communities.
研究兴趣
论文共 88 篇作者统计合作学者相似作者
按年份排序按引用量排序主题筛选期刊级别筛选合作者筛选合作机构筛选
时间
引用量
主题
期刊级别
合作者
合作机构
CLUSTER COMPUTING-THE JOURNAL OF NETWORKS SOFTWARE TOOLS AND APPLICATIONSno. 9 (2024): 12141-12184
JOURNAL OF BUILDING ENGINEERING (2024)
2024 4th International Conference on Emerging Smart Technologies and Applications (eSmarTA)pp.1-17, (2024)
8TH BRUNEI INTERNATIONAL CONFERENCE ON ENGINEERING AND TECHNOLOGY 2021 AIP Conference Proceedings (2023)
Taief Alaa Al-Amiedy,Mohammed Anbar,Bahari Belaton, Abdullah Ahmed Bahashwan,Iznan Husainy Hasbullah, Mohammad Adnan Aladaileh, Ghada AL Mukhaini
2022 7th International Conference on Communication, Image and Signal Processing (CCISP)pp.541-545, (2022)
Symmetryno. 12 (2022): 2556-2556
加载更多
作者统计
#Papers: 88
#Citation: 893
H-Index: 22
G-Index: 25
Sociability: 5
Diversity: 2
Activity: 6
合作学者
合作机构
D-Core
- 合作者
- 学生
- 导师
数据免责声明
页面数据均来自互联网公开来源、合作出版商和通过AI技术自动分析结果,我们不对页面数据的有效性、准确性、正确性、可靠性、完整性和及时性做出任何承诺和保证。若有疑问,可以通过电子邮件方式联系我们:report@aminer.cn