Adversarial Cheap Talk
International Conference on Machine Learning (ICML)(2023)CCF A
University of Oxford | Oxford university
Abstract
Adversarial attacks in reinforcement learning (RL) often assume highly-privileged access to the victim's parameters, environment, or data. Instead, this paper proposes a novel adversarial setting called a Cheap Talk MDP in which an Adversary can merely append deterministic messages to the Victim's observation, resulting in a minimal range of influence. The Adversary cannot occlude ground truth, influence underlying environment dynamics or reward signals, introduce non-stationarity, add stochasticity, see the Victim's actions, or access their parameters. Additionally, we present a simple meta-learning algorithm called Adversarial Cheap Talk (ACT) to train Adversaries in this setting. We demonstrate that an Adversary trained with ACT still significantly influences the Victim's training and testing performance, despite the highly constrained setting. Affecting train-time performance reveals a new attack vector and provides insight into the success and failure modes of existing RL algorithms. More specifically, we show that an ACT Adversary is capable of harming performance by interfering with the learner's function approximation, or instead helping the Victim's performance by outputting useful features. Finally, we show that an ACT Adversary can manipulate messages during train-time to directly and arbitrarily control the Victim at test-time. Project video and code are available at https://sites.google.com/view/adversarial-cheap-talk
MoreTranslated text
Key words
Meta-Learning,Reinforcement Learning,Meta-Reinforcement Learning
PDF
View via Publisher
AI Read Science
AI Summary
AI Summary is the key point extracted automatically understanding the full text of the paper, including the background, methods, results, conclusions, icons and other key content, so that you can get the outline of the paper at a glance.
Example
Background
Key content
Introduction
Methods
Results
Related work
Fund
Key content
- Pretraining has recently greatly promoted the development of natural language processing (NLP)
- We show that M6 outperforms the baselines in multimodal downstream tasks, and the large M6 with 10 parameters can reach a better performance
- We propose a method called M6 that is able to process information of multiple modalities and perform both single-modal and cross-modal understanding and generation
- The model is scaled to large model with 10 billion parameters with sophisticated deployment, and the 10 -parameter M6-large is the largest pretrained model in Chinese
- Experimental results show that our proposed M6 outperforms the baseline in a number of downstream tasks concerning both single modality and multiple modalities We will continue the pretraining of extremely large models by increasing data to explore the limit of its performance
Try using models to generate summary,it takes about 60s
Must-Reading Tree
Example
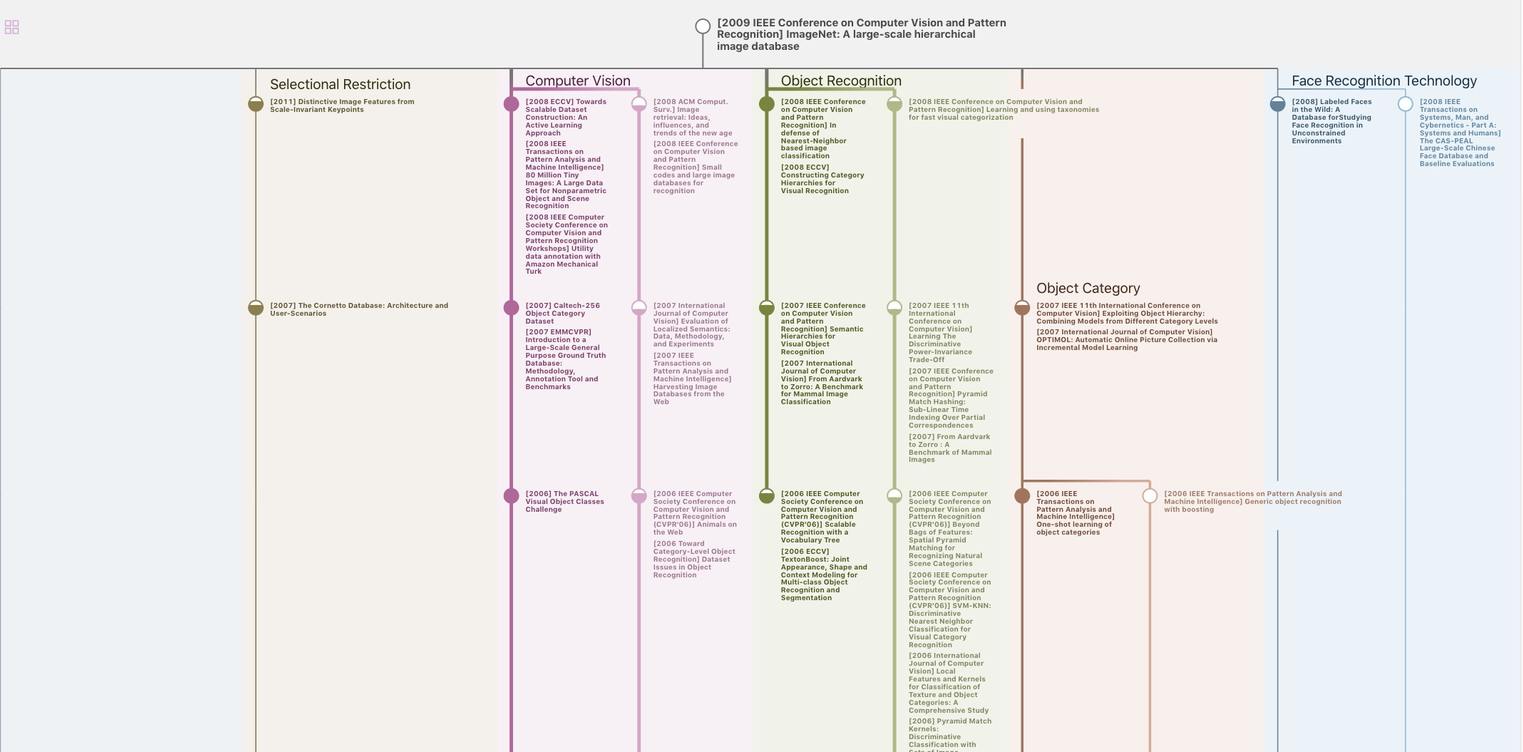
Generate MRT to find the research sequence of this paper
Related Papers
Data Disclaimer
The page data are from open Internet sources, cooperative publishers and automatic analysis results through AI technology. We do not make any commitments and guarantees for the validity, accuracy, correctness, reliability, completeness and timeliness of the page data. If you have any questions, please contact us by email: report@aminer.cn
Chat Paper
去 AI 文献库 对话