Classical to Quantum Transfer Learning Framework for Wireless Sensing Under Domain Shift.
Global Communications Conference(2023)
摘要
To implement ubiquitous wireless sensing, the domain shift problem (e.g., different environments, users, devices) for machine learning based approaches should be addressed. Some existing methods are proven to be effective, such as transfer learning and domain adaptation. Meanwhile, quantum machine learning, a combination of quantum computing and machine learning, has attracted much attention. More importantly, quantum transfer learning (QTL) has been successful for certain applications, e.g., image classification. In this paper, we explore a classical to quantum (C2Q) framework to address the domain shift problem in wireless sensing by exploiting the great potential of QTL. Specifically, we first analyze the data shift problem in various types of wireless datasets by calculating the Kullback-Leibler (KL) divergence of different domains. Then, a QTL framework is designed to introduce importance weighting and adversarial strategies. We finally evaluate the proposed framework using the representative human activity recognition task on three wireless sensing datasets. Experimental results demonstrate the feasibility of the framework and its great potential for solving the domain shift problem in wireless sensing.
更多查看译文
关键词
wireless sensing,quantum machine learning,quantum neural networks,transfer learning
AI 理解论文
溯源树
样例
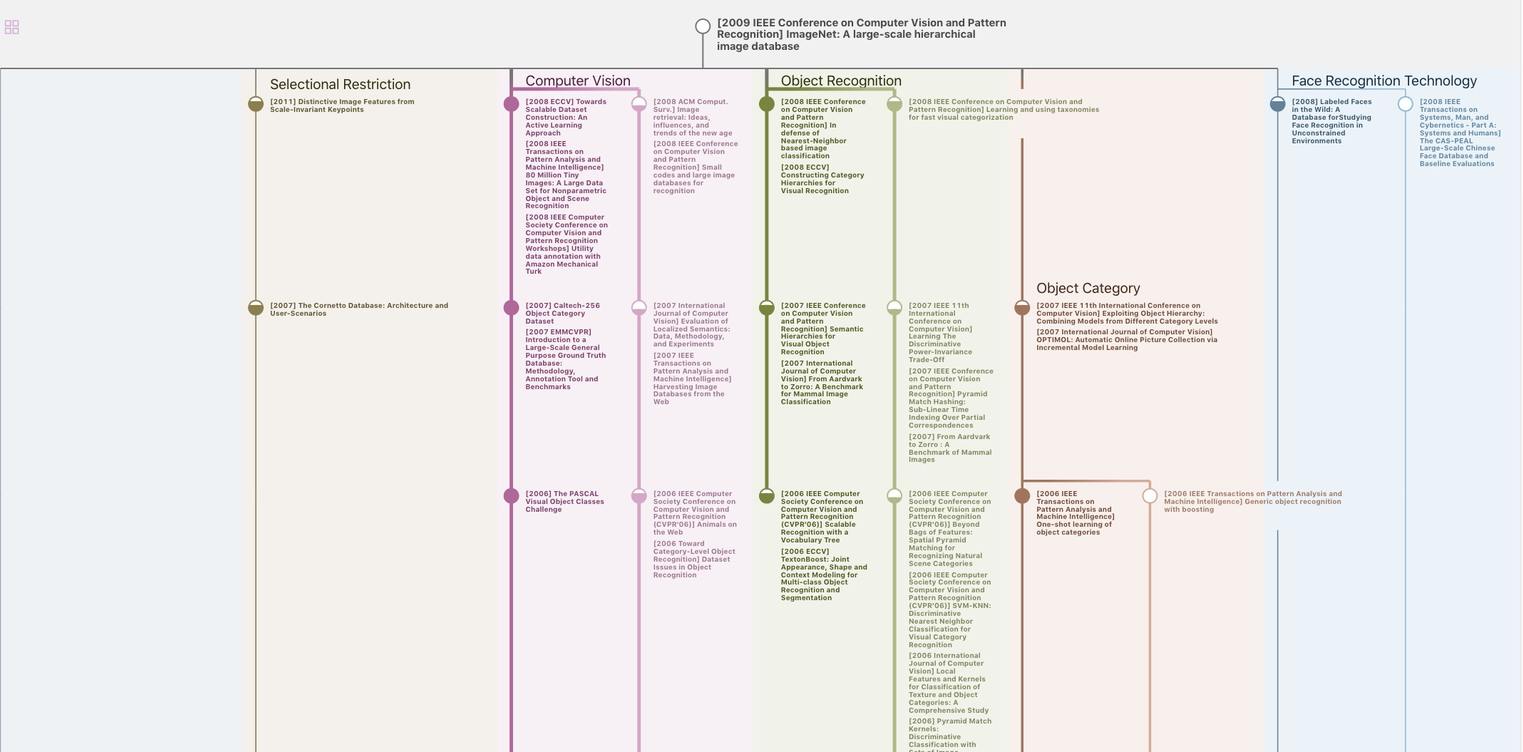
生成溯源树,研究论文发展脉络
Chat Paper
正在生成论文摘要