Decoupled Contrastive Learning for Long-Tailed Distribution
PATTERN RECOGNITION AND COMPUTER VISION, PRCV 2023, PT IX(2024)
摘要
Self-supervised contrastive learning is popularly used to obtain powerful representation models. However, unlabeled data in the real world naturally exhibits a long-tailed distribution, making the traditional instance-wise contrastive learning unfair to tail samples. Recently, some improvements have been made from the perspective of model, loss, and data to make tail samples highly evaluated during training, but most of them explicitly or implicitly assume that the sample with a large loss is the tail. We argue that due to the lack of hard negatives, tail samples usually occupy a small loss at the initial stage of training, which will make them eliminated at the beginning of training. To address this issue, we propose a simple but effective two-stage learning scheme that decouples traditional contrastive learning to discover and enhance tail samples. Specifically, we identify the sample with a small loss in Stage I while a large loss in Stage II as the tail. With the discovered tail samples, we generate hard negatives for them based on their neighbors, which will balance the distribution of the hard negatives in training and help learn better representation. Additionally, we design the weight inversely proportional or proportional to the loss in each stage to achieve fairer training by reweighting. Extensive experiments on multiple unlabeled long-tailed datasets demonstrate the superiority of our DCL compared with the state-of-the-art methods. The code will be released soon.
更多查看译文
关键词
Long-tailed distribution,Unsupervised contrastive learning,Hard negative samples generation
AI 理解论文
溯源树
样例
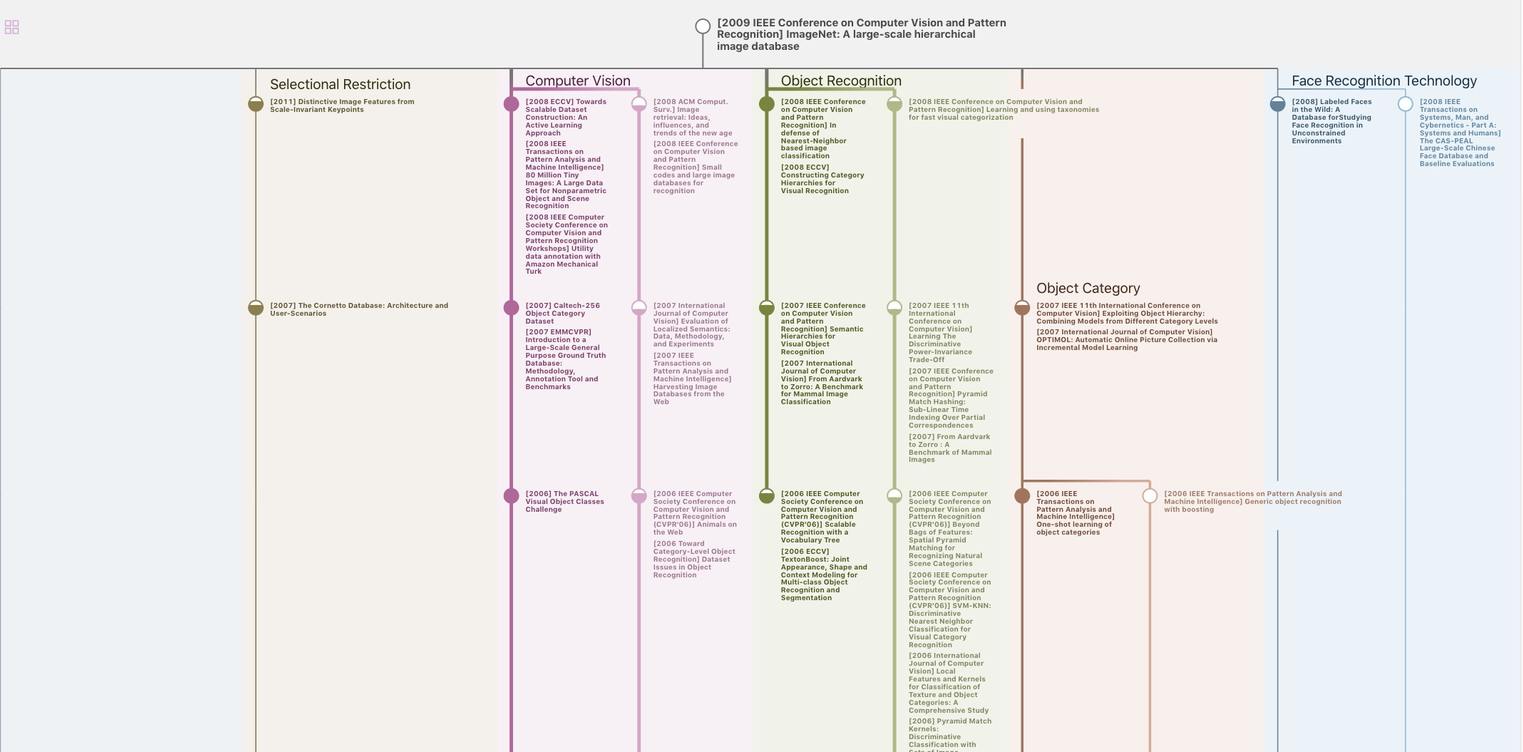
生成溯源树,研究论文发展脉络
Chat Paper
正在生成论文摘要