Exploring “dark matter” protein folds using deep learning
bioRxiv (Cold Spring Harbor Laboratory)(2023)
摘要
Abstract De novo protein design aims to explore uncharted sequence-and structure areas to generate novel proteins that have not been sampled by evolution. One of the main challenges in de novo design involves crafting “designable” structural templates that can guide the sequence search towards adopting the target structures. Here, we present an approach to learn patterns of protein structure based on a convolutional variational autoencoder, dubbed Genesis. We coupled Genesis with trRosetta to design sequences for a set of protein folds and found that Genesis is capable of reconstructing native-like distance-and angle distributions for five native folds and three novel, so-called “dark-matter” folds as a demonstration of generalizability. We used a high-throughput assay to characterize protease resistance of the designs, obtaining encouraging success rates for folded proteins and further biochemically characterized folded designs. The Genesis framework enables the exploration of the protein sequence and fold space within minutes and is not bound to specific protein topologies. Our approach addresses the backbone designability problem, showing that structural patterns in proteins can be efficiently learned by small neural networks and could ultimately contribute to the de novo design of proteins with new functions.
更多查看译文
关键词
dark matter,protein folds,deep learning
AI 理解论文
溯源树
样例
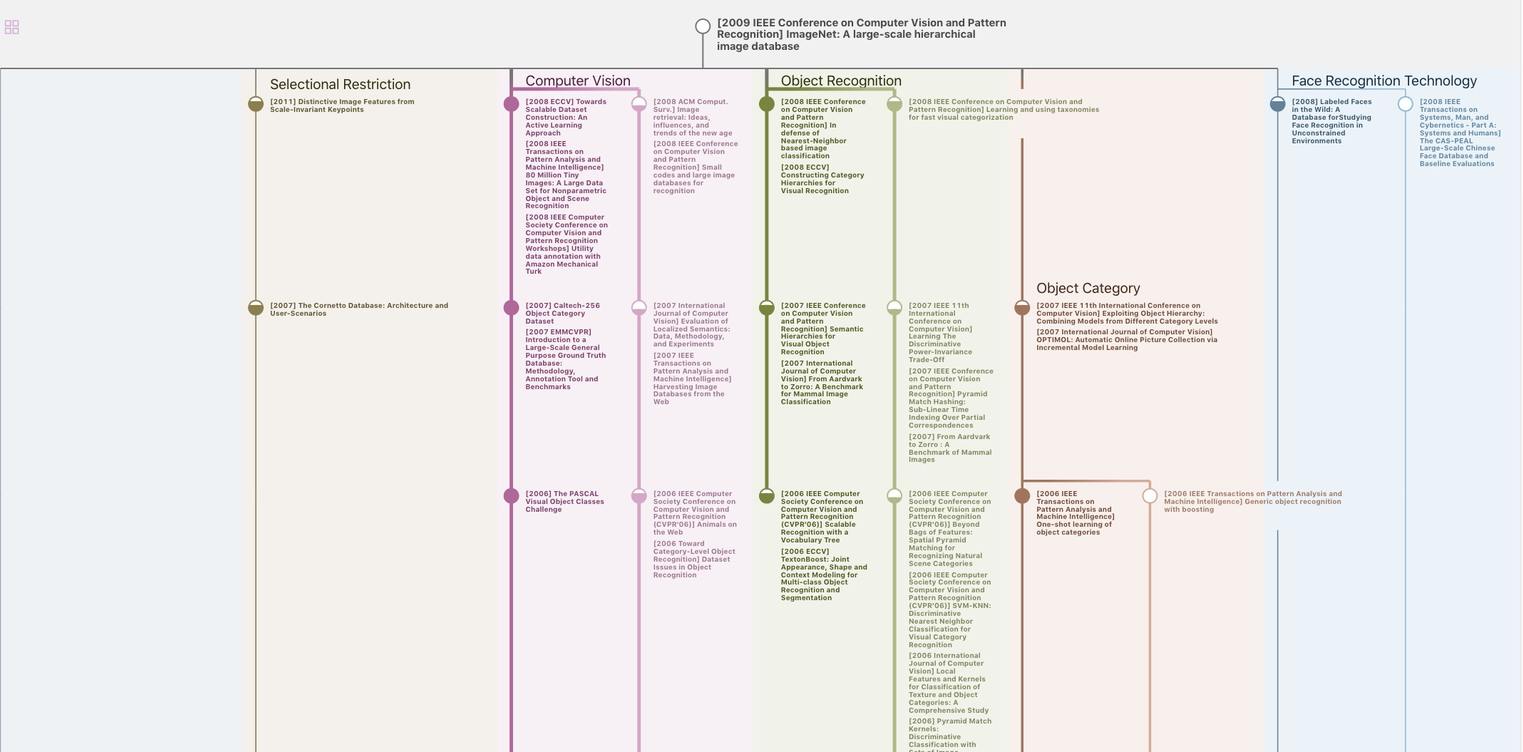
生成溯源树,研究论文发展脉络
Chat Paper
正在生成论文摘要