Benchmarking multi-label prediction of target gene mutation status in glioblastoma using radiogenomics and multi-parametric mr imaging
NEURO-ONCOLOGY(2023)
摘要
PURPOSE In vivo imaging-based prediction of gene mutation status can significantly contribute to the diagnosis and treatment of glioblastoma. Radiogenomics provides a non-invasive approach to capturing the phenotypical characteristics of molecular alterations. We build machine learning (ML) models on radiogenomics and MR imaging data for benchmarking the prediction of multiple target genes in a single institution dataset. METHODS Multi-parametric MRI scans (T1, T1-Gd, T2, T2-FLAIR, DSC, DTI) of 286 individuals with glioblastoma were used to extract radiomics features, including histograms, morphological and textural descriptors. Genetic markers were obtained through a targeted next generation sequencing panel. We built ML models to predict mutation status in two settings: (a) 4 frequently-occurring mutations (EGFR, PTEN, NF1, TP53), (b) 13 mutations including some that are infrequent (PDGFRA, MET, FGFR2, PIK3CA, PIK3R1, BRAF, MDM4, CDKN2A). The models include k-nearest neighbor classifiers, fully-connected neural networks w/o dimensionality reduction trained on radiomics data, convolutional (CNN) and self-attention-based (ViT) networks trained on MR imaging data. All models were trained with 5-fold cross validation on discovery cohort (n=257) and tested on replication cohort (n=29). RESULTS Multi-label prediction models achieved highest accuracies of 75.9% / 88.6% for 4 / 13 mutations using radiomics features. Models trained with radiomics features have a marginally better average F1 score than CNNs and ViTs trained on MR imaging data (by 4.1% / 4.6% for 4 / 13 mutations). CONCLUSION We had modest success in finding highly distinct MR signatures of multiple target mutations in glioblastoma using multi-label ML methods, presumably because of the small sample size and the molecular, the spatial heterogeneity of the tumor, and the difficulty of simultaneously learning multiple imaging signatures. This highlights the importance of pooling and harmonizing radiogenomics data from multiple institutions to increase the sample size, and of incorporating biological domain knowledge into ML models.
更多查看译文
关键词
glioblastoma,radiogenomics,target gene mutation status,mr imaging,multi-label,multi-parametric
AI 理解论文
溯源树
样例
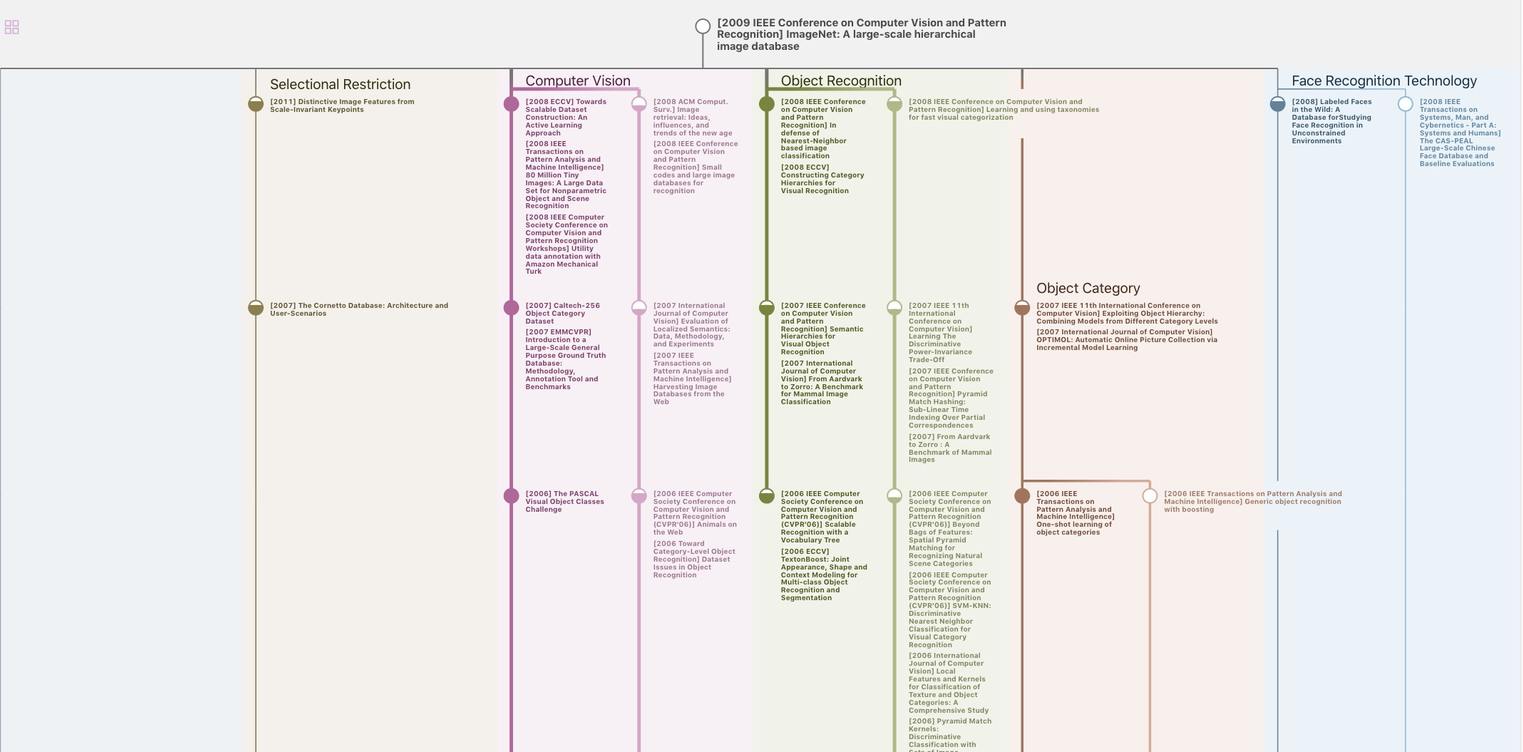
生成溯源树,研究论文发展脉络
Chat Paper
正在生成论文摘要