Machine learning model interpretations explain T cell receptor binding
bioRxiv (Cold Spring Harbor Laboratory)(2023)
摘要
Abstract T cells mediate immune responses against pathogens and cancer through T cell receptors (TCRs) that recognize foreign peptides presented on the cell surface by Major Histocompatibility Complex (MHC) proteins. TCRs carry enormous diversity and differ across individuals, and mechanisms that determine TCR-pMHC binding are poorly understood. The ability to predict TCR-pMHC interactions would accelerate development of cellular therapeutics to design TCRs that specifically bind to a target of interest. We designed a randomized library of 19 6 TCR CDR3 β sequences and experimentally evaluated their affinities for the Tax-A02 peptide-MHC target. We trained ML models that predict TCR binding to Tax-A02 from TCR sequence and used model interpretation to identify TCR sequence features associated with binding. We found these features accurately mirror the true sequence features in our experimental data.
更多查看译文
关键词
receptor,machine learning,model interpretations
AI 理解论文
溯源树
样例
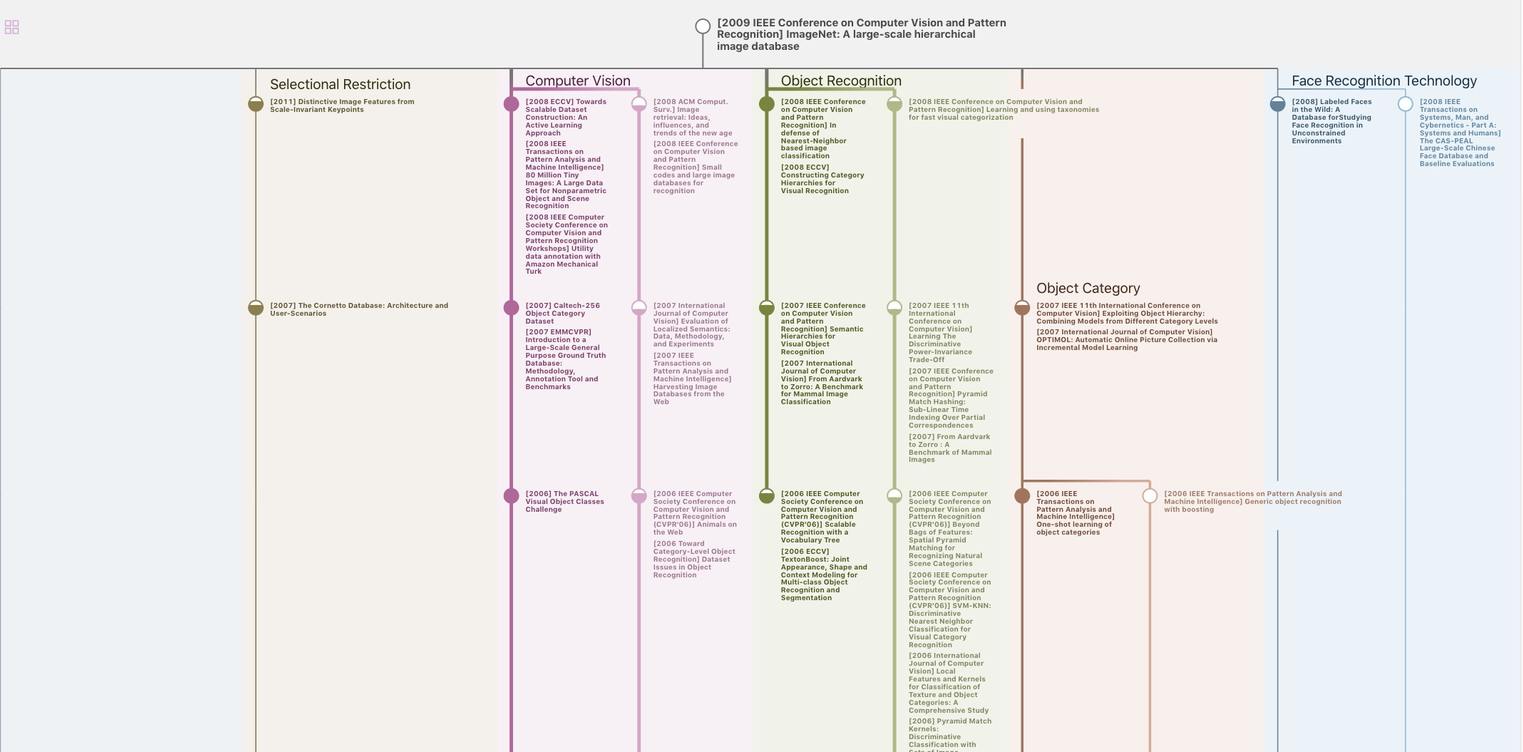
生成溯源树,研究论文发展脉络
Chat Paper
正在生成论文摘要