A 28-nm RRAM Computing-in-Memory Macro Using Weighted Hybrid 2T1R Cell Array and Reference Subtracting Sense Amplifier for AI Edge Inference
IEEE JOURNAL OF SOLID-STATE CIRCUITS(2023)
摘要
Non-volatile computing-in-memory (nvCIM) can potentially meet the ever-increasing demands on improving the energy efficiency (EF) for intelligent edge devices. However, it still suffers from limited input parallelism due to the parasitic effects, signal margin degradation due to device non-idealities, and large hardware cost for analog readout. In this work, we present a two-transistor-one-resistor (2T1R) resistive memory (RRAM) nvCIM macro featuring: 1) a macro structure with decoupled memory and computing data paths; 2) the weighted hybrid 2T1R (WH-2T1R) cell array; 3) the redundant sub-array mapping scheme of the most-significant-bit (RSM-MSB); and 4) reference-subtracting current sense amplifier (RS-CSA). A test-chip is silicon-verified using the 28-nm high-k/metal-gate (HKMG) logic process with foundry-developed RRAM. The test-chip performs linear analog multiply-and-accumulate (MAC) operations over 32 accumulation channels and achieves 30.34–154.04 TOPS/W with 1-bit input (IN), 3-bit weight (W), and 4-bit output (O). Evaluations with the ResNet-18 model show that the MSB-RSM scheme results in 0.96% and 2.83% improvement on CIFAR-10 and CIFAR-100 inference accuracy, respectively.
更多查看译文
关键词
Analog readout,artificial intelligence (AI),computing-in-memory,edge computing,resistive memory (RRAM),sense amplifier (SA)
AI 理解论文
溯源树
样例
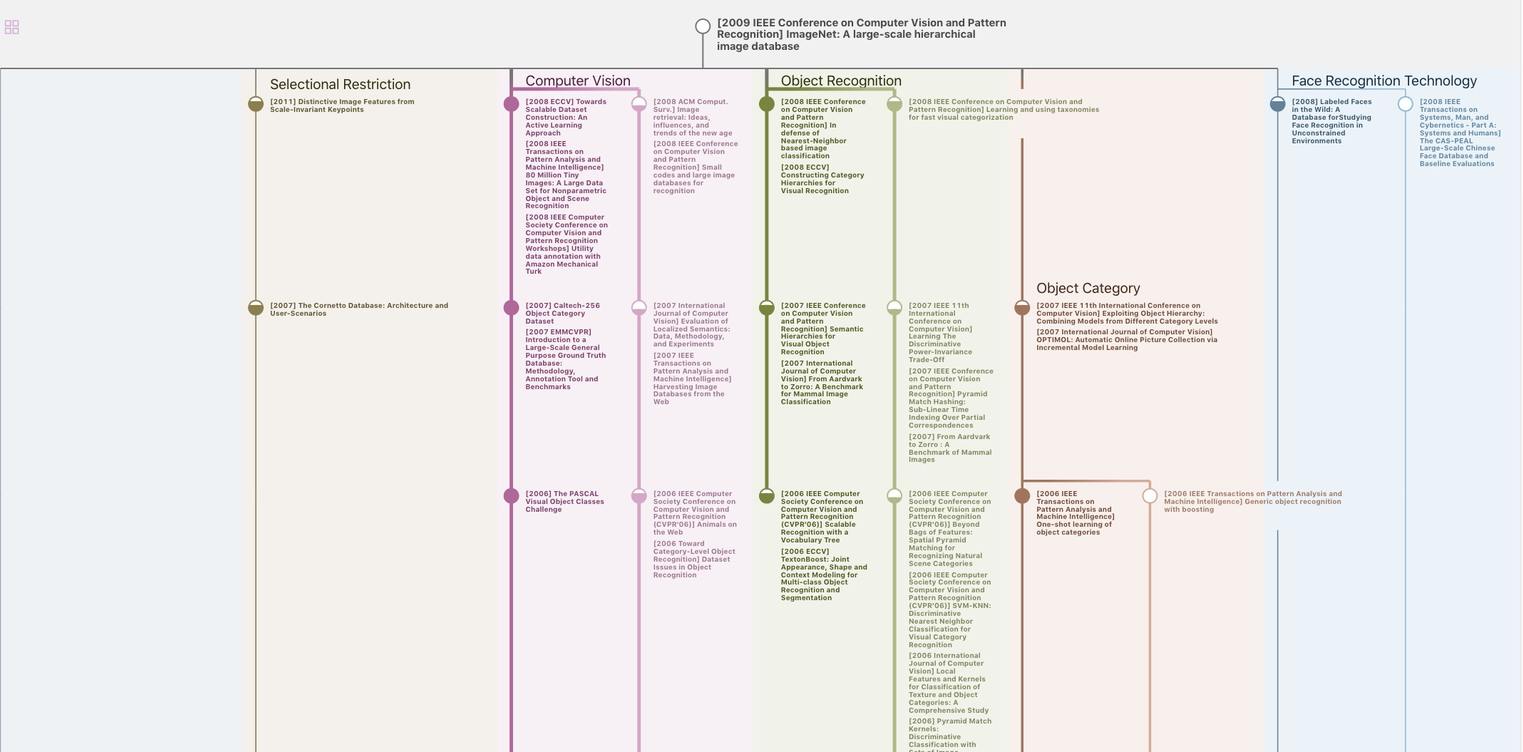
生成溯源树,研究论文发展脉络
Chat Paper
正在生成论文摘要