Leveraging Temporal Context in Low Representational Power Regimes
CVPR 2023(2023)
摘要
Computer vision models are excellent at identifying and exploiting regularities in the world. However, it is computationally costly to learn these regularities from scratch. This presents a challenge for low-parameter models, like those running on edge devices (e.g. smartphones). Can the performance of models with low representational power be improved by supplementing training with additional information about these statistical regularities? We explore this in the domains of action recognition and action anticipation, leveraging the fact that actions are typically embedded in stereotypical sequences. We introduce the Event Transition Matrix (ETM), computed from action labels in an untrimmed video dataset, which captures the temporal context of a given action, operationalized as the likelihood that it was preceded or followed by each other action in the set. We show that including information from the ETM during training improves action recognition and anticipation performance on various egocentric video datasets. Through ablation and control studies, we show that the coherent sequence of information captured by our ETM is key to this effect, and we find that the benefit of this explicit representation of temporal context is most pronounced for smaller models. Code, matrices and models are available in our project page: https://camilofosco.com/etm_website.
更多查看译文
AI 理解论文
溯源树
样例
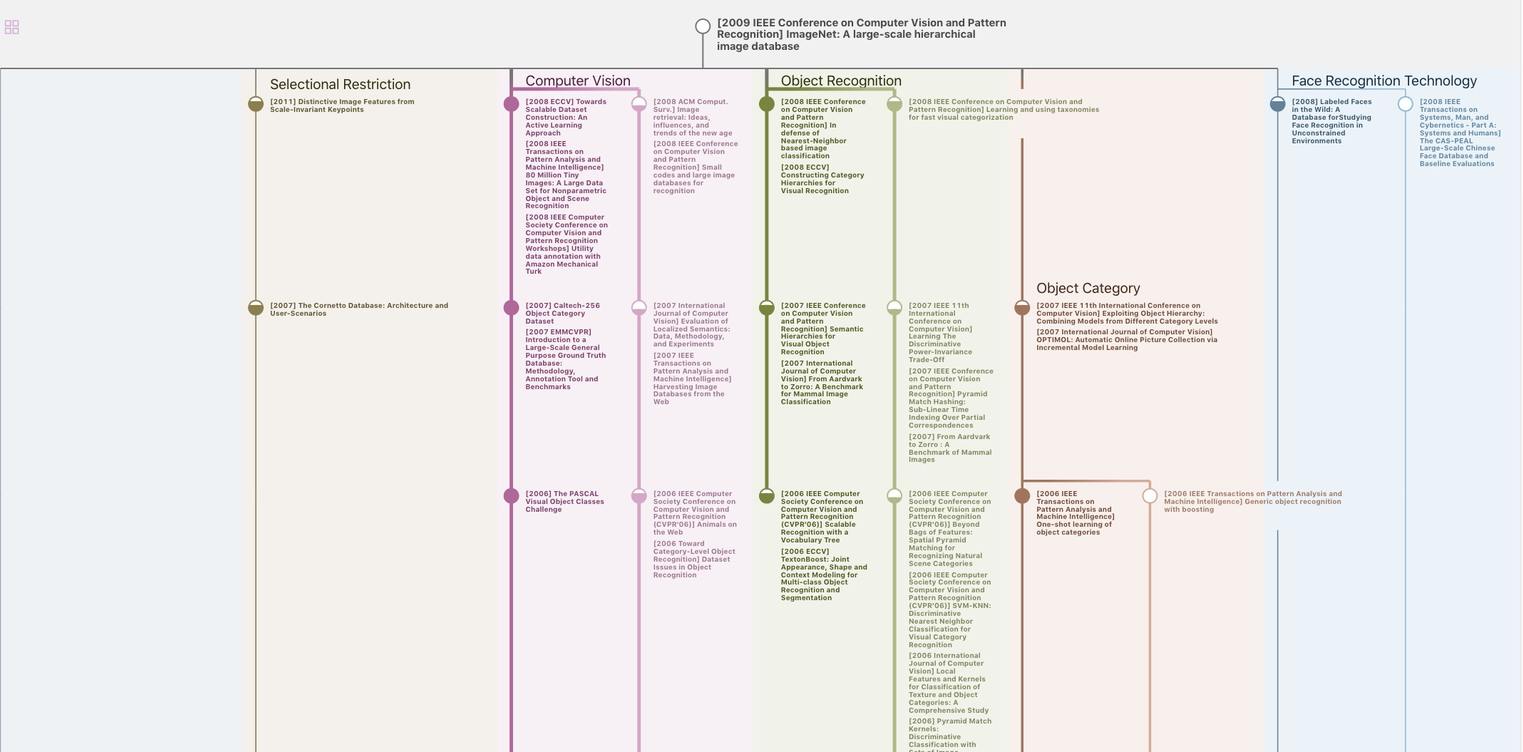
生成溯源树,研究论文发展脉络
Chat Paper
正在生成论文摘要