Differentially private enhanced permissioned blockchain for private data sharing in industrial IoT
INFORMATION SCIENCES(2024)
摘要
The integration of permissioned blockchain such as Hyperledger fabric (HF) and Industrial internet of Things (IIoT) has opened new opportunities for interdependent supply chain partners to improve their performance through data sharing and coordination. The multichannel mechanism, private data collection and querying mechanism of HF enable private data sharing, transparency, traceability, and verification across the supply chain. However, the existing querying mechanism of HF needs further improvement for statistical data sharing because the query is evaluated on the original data recorded on the ledger. As a result, it gives rise to privacy issues such as leaking of business secrets, tracking of resources and assets, and disclosing of personal information. Therefore, we solve this problem by proposing a differentially private enhanced permissioned blockchain for private data sharing in the context of supply chain in IIoT which is known as (EDH-IIoT). First, we integrate differential privacy into the chaincode (smart contract) of HF which evaluates the query and adds a calibrated noise into it. Second, we propose an algorithm to efficiently utilize epsilon through reuse of the privacy budget for the repeated queries. Third, we also propose an algorithm to track the privacy budget (epsilon) and avoid the degrade of privacy preservation in case of multiple queries on the same portion of the ledger's data. Furthermore, the reuse and tracking of epsilon enables the data owner to ensure that e does not exceed the threshold which is the maximum privacy budget (epsilon(t)). Finally, we model two privacy attacks namely linking attack and composition attack to evaluate and compare privacy preservation, and the efficiency of reuse of epsilon with the default chaincode of HF and traditional differential privacy model, respectively. The results confirm that EDH-IIoT obtains an accuracy of 97% in the shared data for epsilon = 1, and a reduction of 35.96% in spending of epsilon.
更多查看译文
关键词
IIoT,Hyperledger fabric,Privacy preservation,Differential privacy,Supply chain,Industrial data sharing,Privacy budget,Tracking of privacy budget
AI 理解论文
溯源树
样例
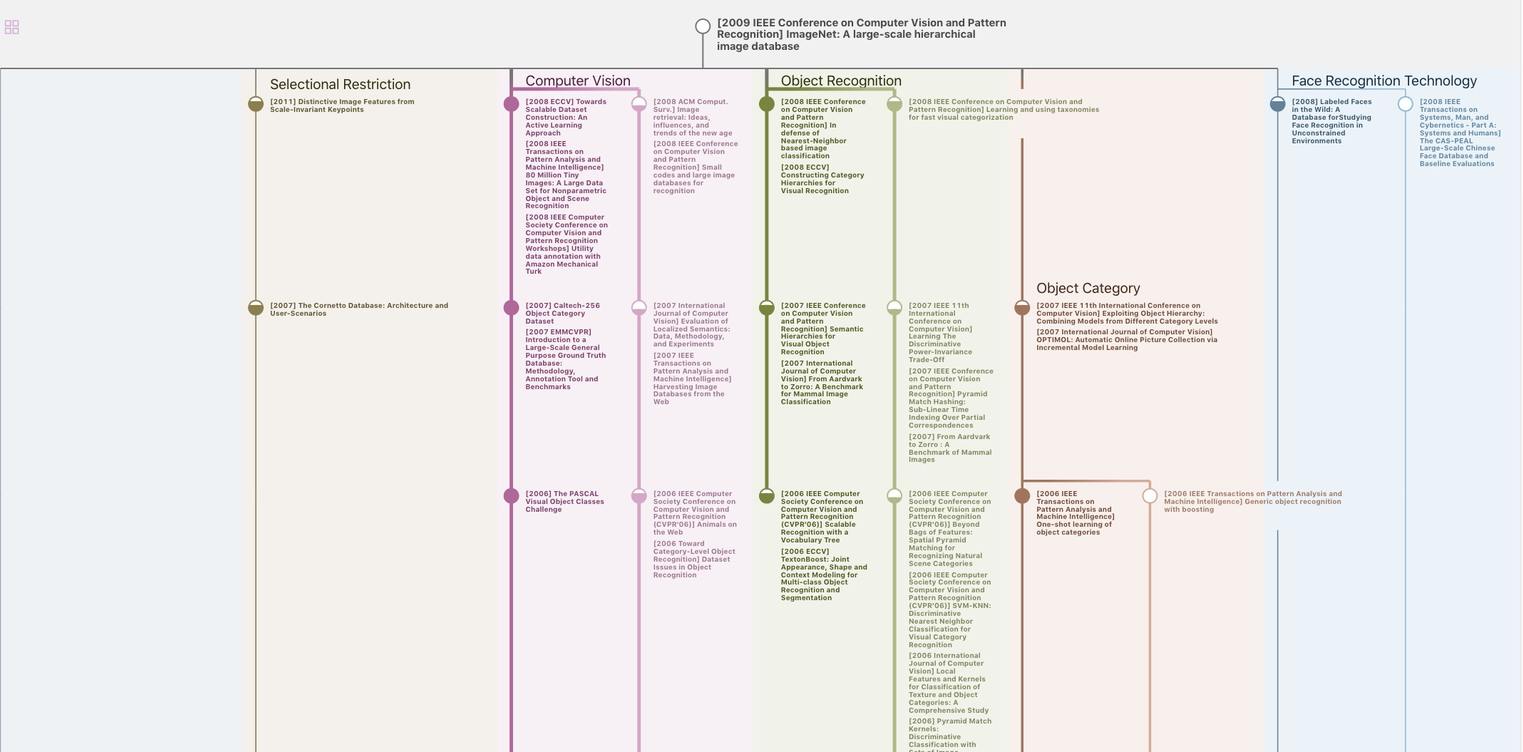
生成溯源树,研究论文发展脉络
Chat Paper
正在生成论文摘要