DeepPeep: Exploiting Design Ramifications to Decipher the Architecture of Compact DNNs
ACM Journal on Emerging Technologies in Computing Systems(2020)
摘要
AbstractThe remarkable predictive performance of deep neural networks (DNNs) has led to their adoption in service domains of unprecedented scale and scope. However, the widespread adoption and growing commercialization of DNNs have underscored the importance of intellectual property (IP) protection. Devising techniques to ensure IP protection has become necessary due to the increasing trend of outsourcing the DNN computations on the untrusted accelerators in cloud-based services. The design methodologies and hyper-parameters of DNNs are crucial information, and leaking them may cause massive economic loss to the organization. Furthermore, the knowledge of DNN’s architecture can increase the success probability of an adversarial attack where an adversary perturbs the inputs and alters the prediction.In this work, we devise a two-stage attack methodology “DeepPeep,” which exploits the distinctive characteristics of design methodologies to reverse-engineer the architecture of building blocks in compact DNNs. We show the efficacy of “DeepPeep” on P100 and P4000 GPUs. Additionally, we propose intelligent design maneuvering strategies for thwarting IP theft through the DeepPeep attack and proposed “Secure MobileNet-V1.” Interestingly, compared to vanilla MobileNet-V1, secure MobileNet-V1 provides a significant reduction in inference latency (≈60%) and improvement in predictive performance (≈2%) with very low memory and computation overheads.
更多查看译文
关键词
Deep neural networks, intellectual property, side-channel attacks
AI 理解论文
溯源树
样例
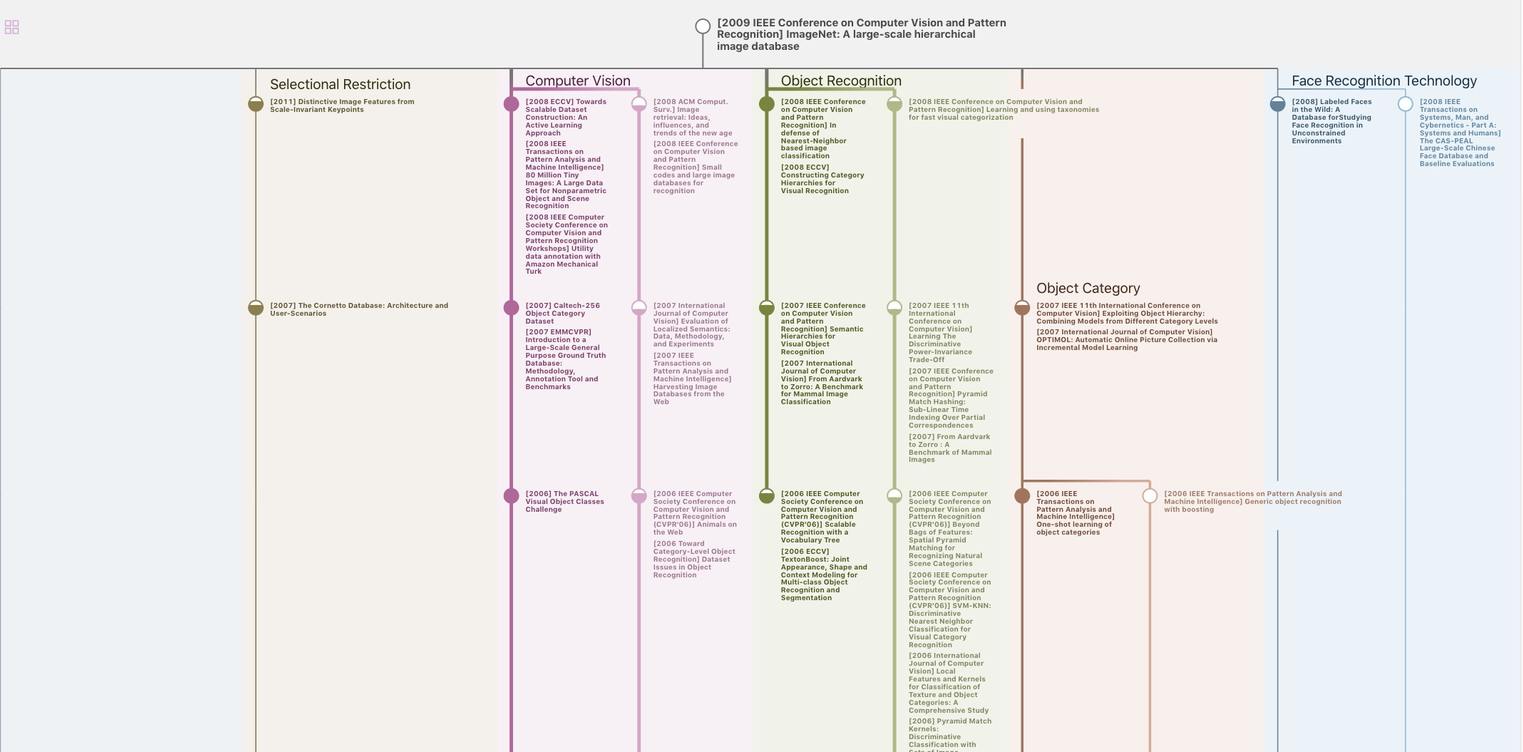
生成溯源树,研究论文发展脉络
Chat Paper
正在生成论文摘要