Two-Class In Silico Categorization Of Intermediate Epileptic Eeg Data
PATTERN RECOGNITION AND MACHINE INTELLIGENCE, PREMI 2019, PT II(2019)
摘要
Epilepsy treatment depends on multiple instances of EEG recordings. Often, clinicians encounter intermediate/borderline EEG signals in the recordings, and as a result, inconclusiveness arises regarding the epilepsy status of a patient. In this paper, we have addressed this issue with a computational solution. We have classified and created class-specific clusters of the EEG data belonging to epileptic patients and normal individuals using a scaled conjugate feed forward neural network (FNN) and average silhouette based k-means clustering algorithm respectively. Thereafter, we have categorized the intermediate data into the clusters of these two classes for a better clinical decision making using minimum squared Euclidean distance. The methodology proposed here can help the clinicians in dealing with intermediate EEG signals found in individuals suspected as suffering from epilepsy. It will also help in categorizing intermediate EEG data, and in turn facilitate clear diagnosis and better patient care in the case of undecided patients.
更多查看译文
关键词
Epilepsy, Epileptic network, Feed forward neural network, k-means clustering, Borderline EEG data
AI 理解论文
溯源树
样例
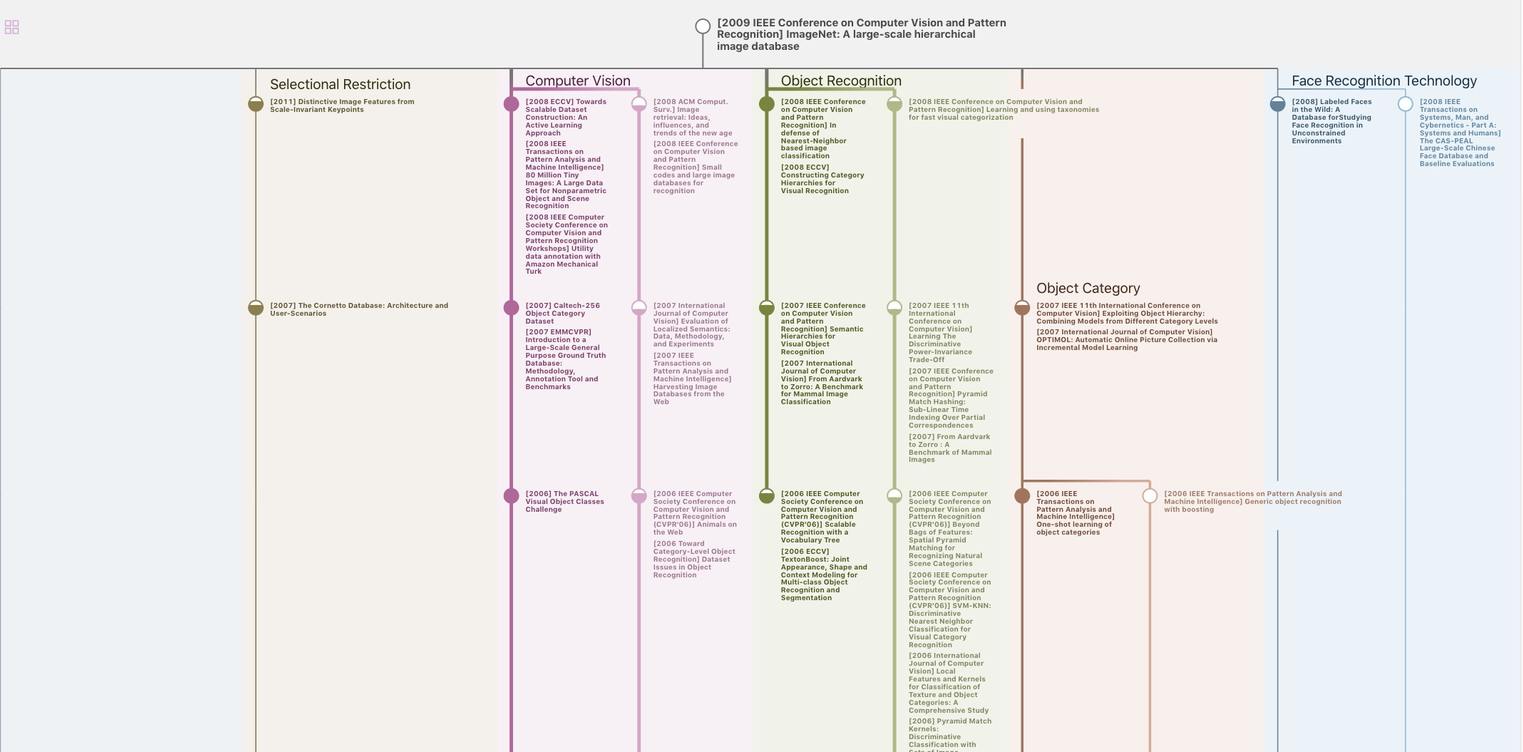
生成溯源树,研究论文发展脉络
Chat Paper
正在生成论文摘要