Efficient Monocular Pose Estimation For Complex 3d Models
2015 IEEE International Conference on Robotics and Automation (ICRA)(2015)
摘要
We propose a robust and efficient method to estimate the pose of a camera with respect to complex 3D textured models of the environment that can potentially contain more than 1 0 0; 0 0 0 points. To tackle this problem we follow a top down approach where we combine high-level deep network classifiers with low level geometric approaches to come up with a solution that is fast, robust and accurate. Given an input image, we initially use a pre-trained deep network to compute a rough estimation of the camera pose. This initial estimate constrains the number of 3D model points that can be seen from the camera viewpoint. We then establish 3D-to-2D correspondences between these potentially visible points of the model and the 2D detected image features. Accurate pose estimation is finally obtained from the 2D-to-3D correspondences using a novel PnP algorithm that rejects outliers without the need to use a RANSAC strategy, and which is between 1 0 and 1 0 0 times faster than other methods that use it. Two real experiments dealing with very large and complex 3D models demonstrate the effectiveness of the approach.
更多查看译文
关键词
monocular pose estimation,complex 3D textured models,high-level deep network classifiers,low level geometric approaches,pretrained deep network,2D detected image features,2D-to-3D correspondences,PnP algorithm
AI 理解论文
溯源树
样例
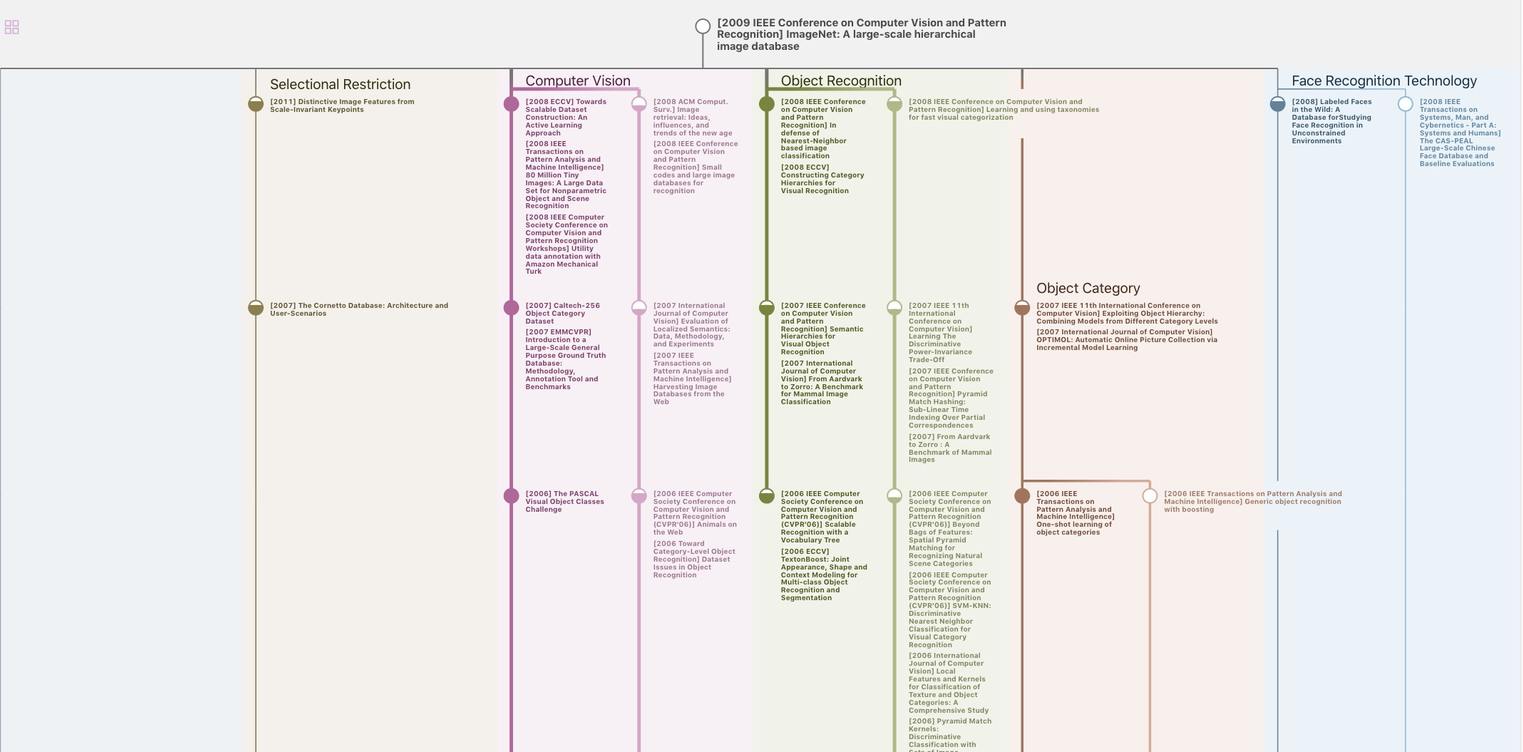
生成溯源树,研究论文发展脉络
Chat Paper
正在生成论文摘要