Sparse Bayesian prediction of disordered residues and disordered regions based on amino-acid composition
Neural Networks(2011)
摘要
This paper presents some initial results of an investigation into the use of machine learning methods to detect natively disordered regions in proteins from sequence information. A committee of Relevance Vector Machines is used to select the optimal window size for residue-by-residue prediction of disordered regions, based on local amino-acid composition. The minimal error rate of ≈ 15% is achieved using very long (205 residue) window lengths, with the classifier making little use of more local sequence information. This suggests that disorder arises principally due to large scale diffuse changes in mean hydropathy and to a lesser extent mean charge. We also demonstrate that the proportion of proteins having long disordered regions in operational conditions cannot be reliably estimated using a classifier trained on a balanced dataset.
更多查看译文
关键词
Bayes methods,biology computing,learning (artificial intelligence),pattern classification,proteins,disordered regions,disordered residues,local amino-acid composition,machine learning methods,optimal window size,relevance vector machines,residue-by-residue prediction,sparse Bayesian prediction
AI 理解论文
溯源树
样例
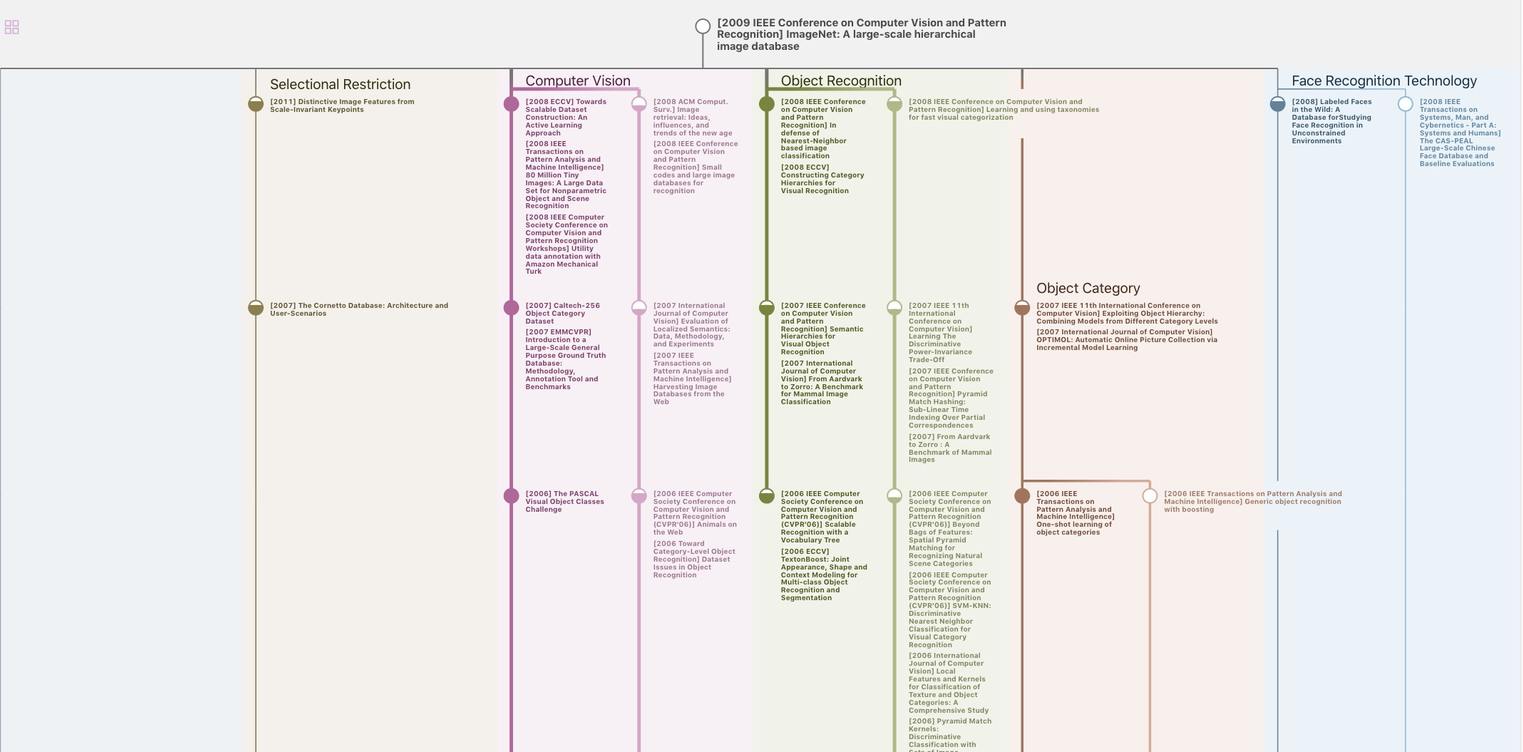
生成溯源树,研究论文发展脉络
Chat Paper
正在生成论文摘要