Constraints on Non-Thermal Pressure at Galaxy Cluster Outskirts from a Joint SPT and XMM-Newton Analysis
arxiv(2025)
Abstract
We present joint South Pole Telescope (SPT) and XMM-Newton observations of 8 massive galaxy clusters (0.8–1.7×10^15 M_⊙) spanning a redshift range of 0.16 to 0.35. Employing a novel SZ+X-ray fitting technique, we effectively constrain the thermodynamic properties of these clusters out to the virial radius. The resulting best-fit electron density, deprojected temperature, and deprojected pressure profiles are in good agreement with previous observations of massive clusters. For the majority of the cluster sample (5 out of 8 clusters), the entropy profiles exhibit a self-similar behavior near the virial radius. We further derive hydrostatic mass, gas mass, and gas fraction profiles for all clusters up to the virial radius. Comparing the enclosed gas fraction profiles with the universal gas fraction profile, we obtain non-thermal pressure fraction profiles for our cluster sample at >R_500, demonstrating a steeper increase between R_500 and R_200 that is consistent with the hydrodynamical simulations. Our analysis yields non-thermal pressure fraction ranges of 8–28 R_500 and 21–35 weak-lensing mass measurements are available for only four clusters in our sample, and our recovered total cluster masses, after accounting for non-thermal pressure, are consistent with these measurements.
MoreTranslated text
PDF
View via Publisher
AI Read Science
AI Summary
AI Summary is the key point extracted automatically understanding the full text of the paper, including the background, methods, results, conclusions, icons and other key content, so that you can get the outline of the paper at a glance.
Example
Background
Key content
Introduction
Methods
Results
Related work
Fund
Key content
- Pretraining has recently greatly promoted the development of natural language processing (NLP)
- We show that M6 outperforms the baselines in multimodal downstream tasks, and the large M6 with 10 parameters can reach a better performance
- We propose a method called M6 that is able to process information of multiple modalities and perform both single-modal and cross-modal understanding and generation
- The model is scaled to large model with 10 billion parameters with sophisticated deployment, and the 10 -parameter M6-large is the largest pretrained model in Chinese
- Experimental results show that our proposed M6 outperforms the baseline in a number of downstream tasks concerning both single modality and multiple modalities We will continue the pretraining of extremely large models by increasing data to explore the limit of its performance
Try using models to generate summary,it takes about 60s
Must-Reading Tree
Example
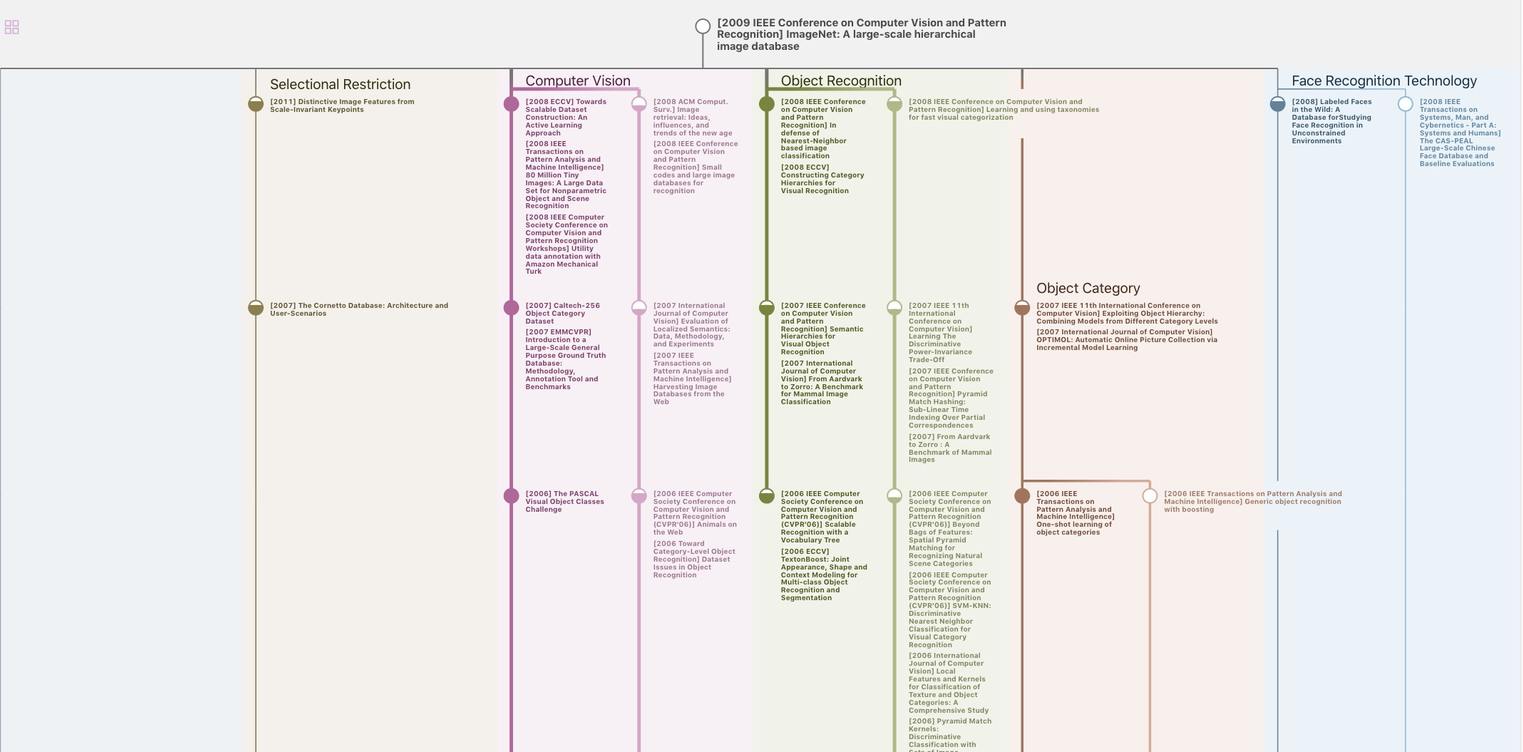
Generate MRT to find the research sequence of this paper
Data Disclaimer
The page data are from open Internet sources, cooperative publishers and automatic analysis results through AI technology. We do not make any commitments and guarantees for the validity, accuracy, correctness, reliability, completeness and timeliness of the page data. If you have any questions, please contact us by email: report@aminer.cn
Chat Paper
去 AI 文献库 对话