The 200 Gbps Challenge: Imagining HL-LHC Analysis Facilities
arXiv · Experiment(2025)
Abstract
The IRIS-HEP software institute, as a contributor to the broader HEP Python ecosystem, is developing scalable analysis infrastructure and software tools to address the upcoming HL-LHC computing challenges with new approaches and paradigms, driven by our vision of what HL-LHC analysis will require. The institute uses a "Grand Challenge" format, constructing a series of increasingly large, complex, and realistic exercises to show the vision of HL-LHC analysis. Recently, the focus has been demonstrating the IRIS-HEP analysis infrastructure at scale and evaluating technology readiness for production. As a part of the Analysis Grand Challenge activities, the institute executed a "200 Gbps Challenge", aiming to show sustained data rates into the event processing of multiple analysis pipelines. The challenge integrated teams internal and external to the institute, including operations and facilities, analysis software tools, innovative data delivery and management services, and scalable analysis infrastructure. The challenge showcases the prototypes - including software, services, and facilities - built to process around 200 TB of data in both the CMS NanoAOD and ATLAS PHYSLITE data formats with test pipelines. The teams were able to sustain the 200 Gbps target across multiple pipelines. The pipelines focusing on event rate were able to process at over 30 MHz. These target rates are demanding; the activity revealed considerations for future testing at this scale and changes necessary for physicists to work at this scale in the future. The 200 Gbps Challenge has established a baseline on today's facilities, setting the stage for the next exercise at twice the scale.
MoreTranslated text
PDF
View via Publisher
AI Read Science
AI Summary
AI Summary is the key point extracted automatically understanding the full text of the paper, including the background, methods, results, conclusions, icons and other key content, so that you can get the outline of the paper at a glance.
Example
Background
Key content
Introduction
Methods
Results
Related work
Fund
Key content
- Pretraining has recently greatly promoted the development of natural language processing (NLP)
- We show that M6 outperforms the baselines in multimodal downstream tasks, and the large M6 with 10 parameters can reach a better performance
- We propose a method called M6 that is able to process information of multiple modalities and perform both single-modal and cross-modal understanding and generation
- The model is scaled to large model with 10 billion parameters with sophisticated deployment, and the 10 -parameter M6-large is the largest pretrained model in Chinese
- Experimental results show that our proposed M6 outperforms the baseline in a number of downstream tasks concerning both single modality and multiple modalities We will continue the pretraining of extremely large models by increasing data to explore the limit of its performance
Try using models to generate summary,it takes about 60s
Must-Reading Tree
Example
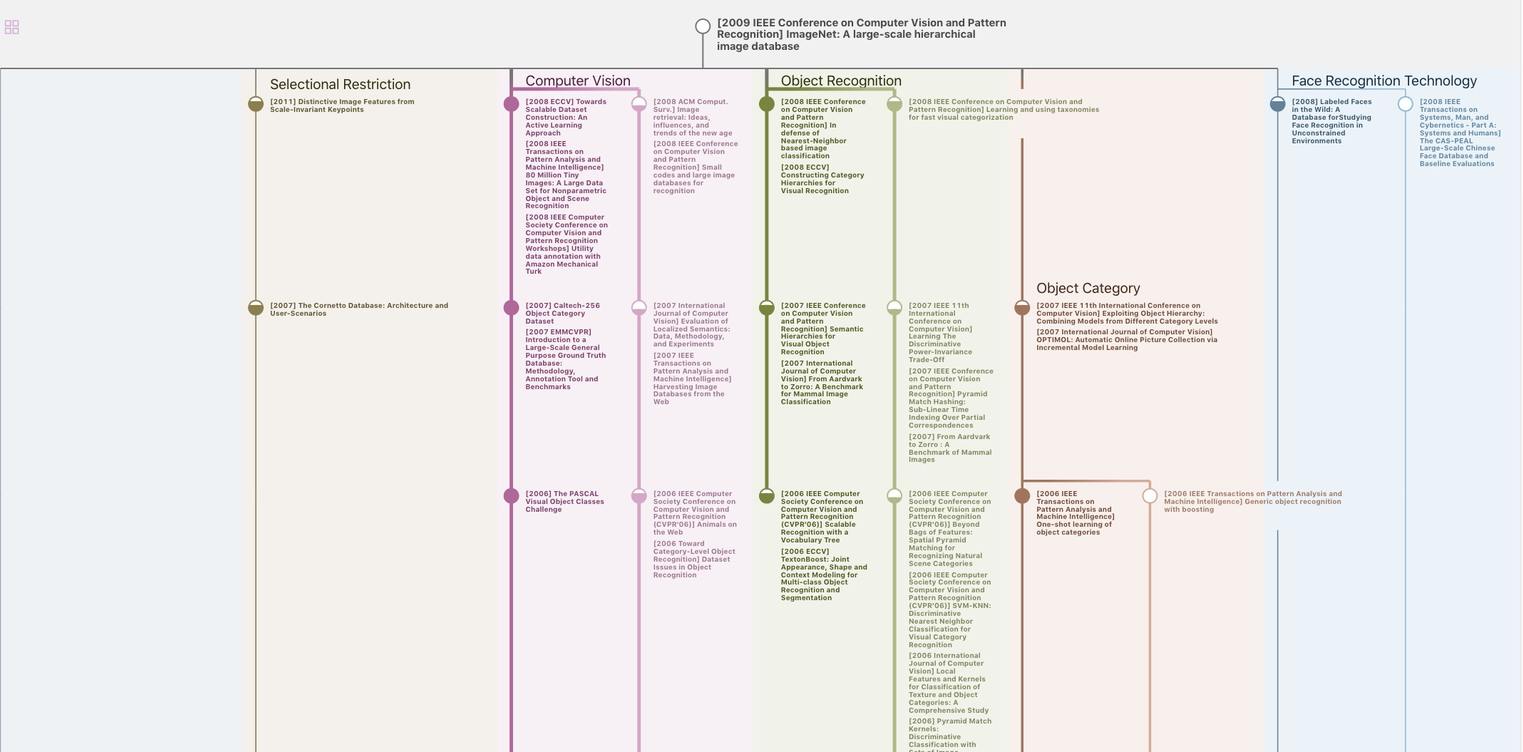
Generate MRT to find the research sequence of this paper
Data Disclaimer
The page data are from open Internet sources, cooperative publishers and automatic analysis results through AI technology. We do not make any commitments and guarantees for the validity, accuracy, correctness, reliability, completeness and timeliness of the page data. If you have any questions, please contact us by email: report@aminer.cn
Chat Paper
GPU is busy, summary generation fails
Rerequest