The Atacama Cosmology Telescope: the Development of Machine Learning Tools for Detecting Millimeter Sources in Timestream Pre-processing
arXiv · Instrumentation and Methods for Astrophysics(2025)
Abstract
We present a new machine learning algorithm for classifying short-duration features in raw time ordered data (TODs) of cosmic microwave background survey observations. The algorithm, specifically designed for the Atacama Cosmology Telescope (ACT), works in conjunction with the previous TOD preprocessing techniques that employ statistical thresholding to indiscriminately remove all large spikes in the data, whether they are due to noise features, cosmic rays, or true astrophysical sources in a process called "data cuts". This has the undesirable effect of excising real astrophysical sources, including transients, from the data. The machine learning algorithm demonstrated in this work uses the output from these data cuts and is able to differentiate between electronic noise, cosmic rays, and point sources, enabling the removal of undesired signals while retaining true astrophysical signals during TOD pre-processing. We achieve an overall accuracy of 90 spikes of different origin and, importantly, 94 by astrophysical sources. Our algorithm also measures the amplitude of any detected source seen more than once and produces a sub-minute to minute light curve, providing information on its short timescale variability. This automated algorithm for source detection and amplitude estimation will be particularly useful for upcoming surveys with large data volumes, such as the Simons Observatory.
MoreTranslated text
PDF
View via Publisher
AI Read Science
AI Summary
AI Summary is the key point extracted automatically understanding the full text of the paper, including the background, methods, results, conclusions, icons and other key content, so that you can get the outline of the paper at a glance.
Example
Background
Key content
Introduction
Methods
Results
Related work
Fund
Key content
- Pretraining has recently greatly promoted the development of natural language processing (NLP)
- We show that M6 outperforms the baselines in multimodal downstream tasks, and the large M6 with 10 parameters can reach a better performance
- We propose a method called M6 that is able to process information of multiple modalities and perform both single-modal and cross-modal understanding and generation
- The model is scaled to large model with 10 billion parameters with sophisticated deployment, and the 10 -parameter M6-large is the largest pretrained model in Chinese
- Experimental results show that our proposed M6 outperforms the baseline in a number of downstream tasks concerning both single modality and multiple modalities We will continue the pretraining of extremely large models by increasing data to explore the limit of its performance
Try using models to generate summary,it takes about 60s
Must-Reading Tree
Example
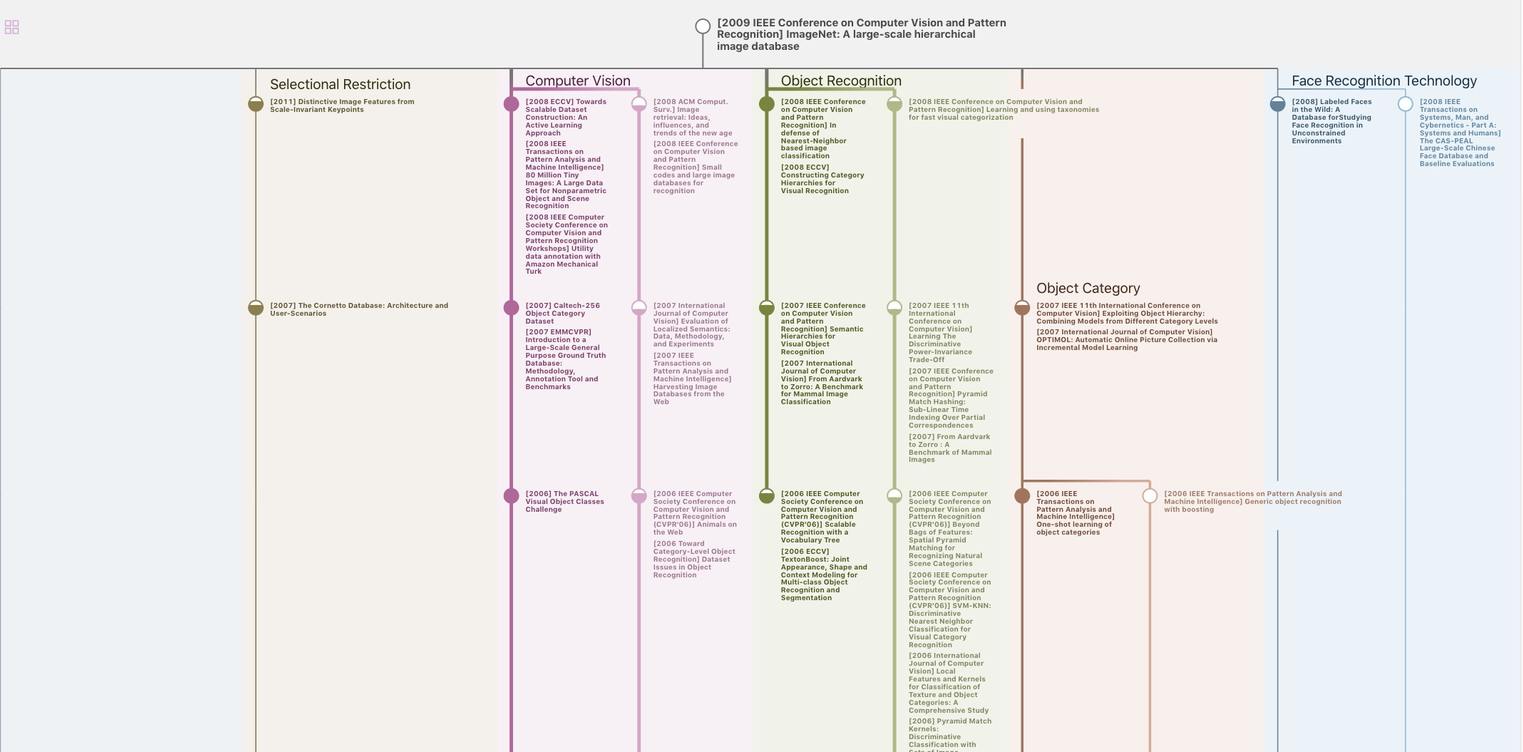
Generate MRT to find the research sequence of this paper
Data Disclaimer
The page data are from open Internet sources, cooperative publishers and automatic analysis results through AI technology. We do not make any commitments and guarantees for the validity, accuracy, correctness, reliability, completeness and timeliness of the page data. If you have any questions, please contact us by email: report@aminer.cn
Chat Paper
去 AI 文献库 对话