Clinicopathological Prognostic Model for Survival in Adult Patients with Secondary Hemophagocytic Lymphohistiocytosis.
European journal of haematology(2025)
Department of Pharmacy
Abstract
BACKGROUND:Data on bone marrow (BM) findings in secondary hemophagocytic lymphohistiocytosis (sHLH) and their association with overall survival (OS) are limited. OBJECTIVES:This study aimed to develop a prognostic model incorporating BM findings and clinico-laboratory factors affecting OS. METHODS:We retrospectively evaluated 50 adults with sHLH and developed a clinicopathological prognostic model based on survival-associated factors. RESULTS:Most patients demonstrated normocellular BM (46.3%) and mild hemophagocytic activity (44.2%). Factors associated with survival in multivariable analyses (MVA) were age above 70 years (hazard ratio [HR] 3.89, p = 0.016), infection-related (HR 4.62, p = 0.006), hemoglobin < 7 g/dL (HR 5.21, p < 0.001), and hypocellular marrow (HR 3.07, p = 0.04). A clinicopathological HLH risk model assigned 1 point to each MVA-identified survival factor, categorizing patients into low- (score 0-1), intermediate- (score 2-3), and high-risk (score 4) groups. The 6-month OS from bootstrapping internal validation among the low-, intermediate-, and high-risk groups were 84.2%, 55.6% (p < 0.001) and 7.7% (p < 0.001), respectively. The area under the receiver operating characteristic curve (AuROC) was 0.87. CONCLUSIONS:This model stratified sHLH patients into three risk groups with distinct survival outcomes, potentially guiding future therapy.
MoreTranslated text
求助PDF
上传PDF
View via Publisher
AI Read Science
AI Summary
AI Summary is the key point extracted automatically understanding the full text of the paper, including the background, methods, results, conclusions, icons and other key content, so that you can get the outline of the paper at a glance.
Example
Background
Key content
Introduction
Methods
Results
Related work
Fund
Key content
- Pretraining has recently greatly promoted the development of natural language processing (NLP)
- We show that M6 outperforms the baselines in multimodal downstream tasks, and the large M6 with 10 parameters can reach a better performance
- We propose a method called M6 that is able to process information of multiple modalities and perform both single-modal and cross-modal understanding and generation
- The model is scaled to large model with 10 billion parameters with sophisticated deployment, and the 10 -parameter M6-large is the largest pretrained model in Chinese
- Experimental results show that our proposed M6 outperforms the baseline in a number of downstream tasks concerning both single modality and multiple modalities We will continue the pretraining of extremely large models by increasing data to explore the limit of its performance
Upload PDF to Generate Summary
Must-Reading Tree
Example
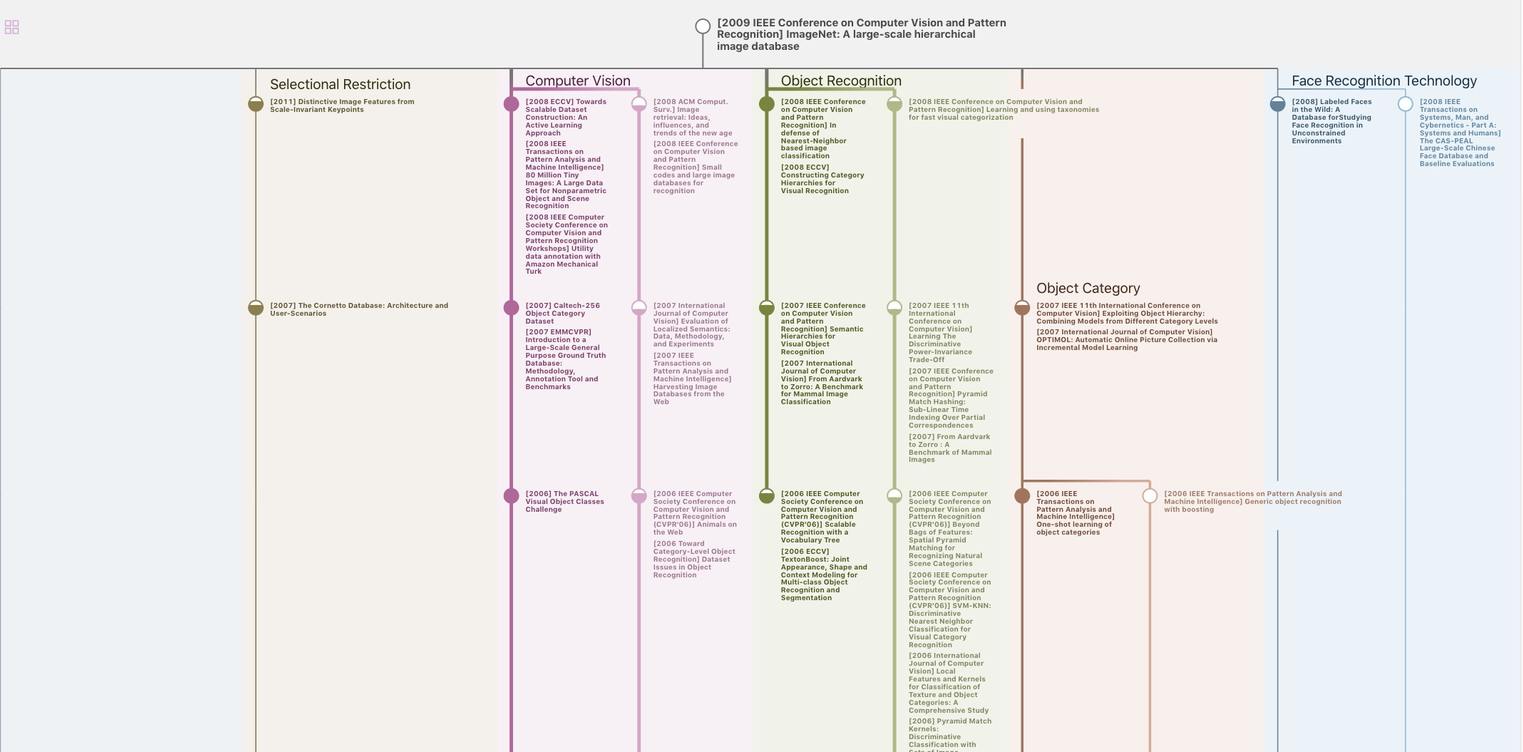
Generate MRT to find the research sequence of this paper
Data Disclaimer
The page data are from open Internet sources, cooperative publishers and automatic analysis results through AI technology. We do not make any commitments and guarantees for the validity, accuracy, correctness, reliability, completeness and timeliness of the page data. If you have any questions, please contact us by email: report@aminer.cn
Chat Paper
GPU is busy, summary generation fails
Rerequest