A Comparative Analysis of Data-Driven Modeling Approaches to Forecast Cyanobacteria Algal Blooms in Eutrophic Lake Discharge Canals.
Journal of environmental management(2025)
South Florida Water Management District
Abstract
This study explored the use of data-driven models to develop management-oriented prediction tools for algae blooms (ABs) represented by Chlorophyll-A (Chla) concentrations, using the Caloosahatchee and St. Lucie canals in Lake Okeechobee, Florida, as case studies. By comparing two modeling approaches, i.e., cascading modeling and time-lag modeling, the study aims to understand the differences in Chla dynamics between the two canals, identify the main drivers and predictors of Chla concentration in each, and develop suitable forecasting models for the canals' operation purposes. Throughout this study, both approaches demonstrated their value in improving the understanding of water quality dynamics in Lake Okeechobee canals. While some water quality parameters such as Dissolved Oxygen (DO) and Nitrate-Nitrite (NOx) were critical to ABs in the Caloosahatchee and St. Lucie canals, respectively, the effect of operation decisions on ABs was more significant on the St. Lucie than on the Caloosahatchee. From a modeling perspective, the time-lag modeling approach achieved higher predictive accuracy for Chla concentrations in both Caloosahatchee and St. Lucie canals. Particularly, at station S80 of St. Lucie canal, the XGBoost (XGB) algorithm achieved R2= 99% and RMSE = 0.001 μg/l in training, and R2= 60.1% and RMSE = 4.58 μg/l in testing. At station S79 of Caloosahatchee canal, Random Forest (RF) appeared to be the best model with R2= 85.7% and RMSE = 5.63 μg/l in training, and R2= 39% with RMSE = 10.06 μg/l in testing. In this study, the time-lag modeling approach was proven to offer decision-makers flexible tools for implementing better management strategies based solely on operation decisions and meteorological conditions.
MoreTranslated text
求助PDF
上传PDF
View via Publisher
AI Read Science
AI Summary
AI Summary is the key point extracted automatically understanding the full text of the paper, including the background, methods, results, conclusions, icons and other key content, so that you can get the outline of the paper at a glance.
Example
Background
Key content
Introduction
Methods
Results
Related work
Fund
Key content
- Pretraining has recently greatly promoted the development of natural language processing (NLP)
- We show that M6 outperforms the baselines in multimodal downstream tasks, and the large M6 with 10 parameters can reach a better performance
- We propose a method called M6 that is able to process information of multiple modalities and perform both single-modal and cross-modal understanding and generation
- The model is scaled to large model with 10 billion parameters with sophisticated deployment, and the 10 -parameter M6-large is the largest pretrained model in Chinese
- Experimental results show that our proposed M6 outperforms the baseline in a number of downstream tasks concerning both single modality and multiple modalities We will continue the pretraining of extremely large models by increasing data to explore the limit of its performance
Upload PDF to Generate Summary
Must-Reading Tree
Example
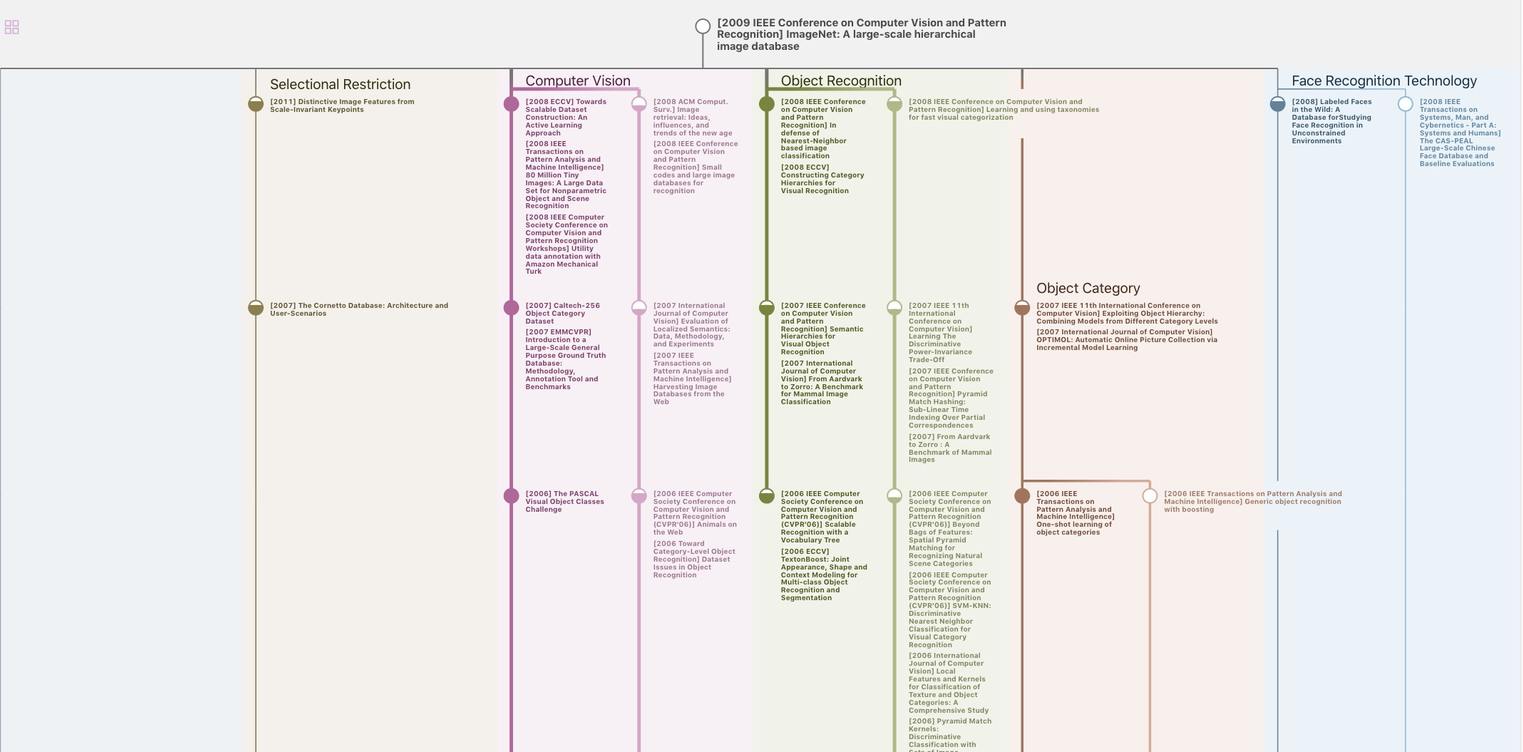
Generate MRT to find the research sequence of this paper
Data Disclaimer
The page data are from open Internet sources, cooperative publishers and automatic analysis results through AI technology. We do not make any commitments and guarantees for the validity, accuracy, correctness, reliability, completeness and timeliness of the page data. If you have any questions, please contact us by email: report@aminer.cn
Chat Paper