Targeted Password Guessing Using Neural Language Models
ICASSP 2025 - 2025 IEEE International Conference on Acoustics, Speech and Signal Processing (ICASSP)(2025)
Peking University | Beijing Institute of Graphic Communication
Abstract
With the increasing prevalence of personal information breaches, targeted password guessing based on user-specific data has emerged as a serious security threat. Existing targeted password guessing attacks primarily rely on traditional statistical language models, which have limited capability in addressing the complexities of password structures and user behavior. Recent advancements in neural language models, particularly Transformer-based architectures, have achieved significant success in natural language processing tasks by capturing complex patterns and dependencies. However, their potential for improving targeted password guessing remains largely unexplored.To address this gap, we conduct a systematic evaluation of several widely used neural language models from NLP and assess their effectiveness in targeted password guessing. Experimental results on multiple real-world password datasets show that neural language models outperform existing approaches. Our proposed models achieve an improvement of 1.4%–4.6% compared to RFGuess-PII model, and 18%–40% compared to TarPCFG model. This work provides new insights into the potential of neural language models to enhance the effectiveness of targeted password guessing attacks.
MoreTranslated text
Key words
Password security,targeted password guessing,neural language models,personal information,password generation
求助PDF
上传PDF
View via Publisher
AI Read Science
AI Summary
AI Summary is the key point extracted automatically understanding the full text of the paper, including the background, methods, results, conclusions, icons and other key content, so that you can get the outline of the paper at a glance.
Example
Background
Key content
Introduction
Methods
Results
Related work
Fund
Key content
- Pretraining has recently greatly promoted the development of natural language processing (NLP)
- We show that M6 outperforms the baselines in multimodal downstream tasks, and the large M6 with 10 parameters can reach a better performance
- We propose a method called M6 that is able to process information of multiple modalities and perform both single-modal and cross-modal understanding and generation
- The model is scaled to large model with 10 billion parameters with sophisticated deployment, and the 10 -parameter M6-large is the largest pretrained model in Chinese
- Experimental results show that our proposed M6 outperforms the baseline in a number of downstream tasks concerning both single modality and multiple modalities We will continue the pretraining of extremely large models by increasing data to explore the limit of its performance
Upload PDF to Generate Summary
Must-Reading Tree
Example
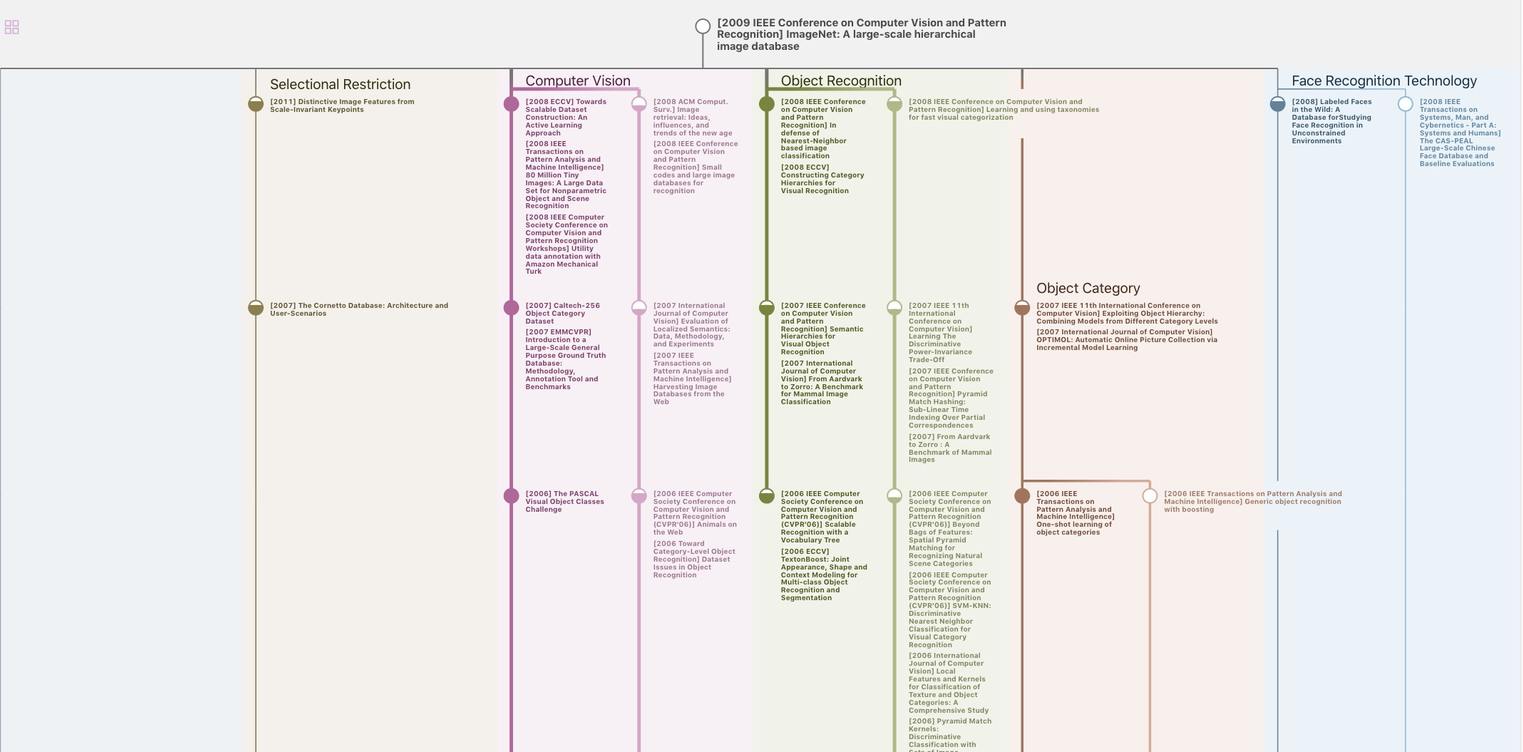
Generate MRT to find the research sequence of this paper
Data Disclaimer
The page data are from open Internet sources, cooperative publishers and automatic analysis results through AI technology. We do not make any commitments and guarantees for the validity, accuracy, correctness, reliability, completeness and timeliness of the page data. If you have any questions, please contact us by email: report@aminer.cn
Chat Paper