COSMOS Spectroscopic Redshift Compilation (first Data Release): 165k Redshifts Encompassing Two Decades of Spectroscopy
arXiv · Astrophysics of Galaxies(2025)
Abstract
We present the COSMOS Spectroscopic Redshift Compilation encompassing 20 years of spectroscopic redshifts within the 2 deg^2 COSMOS legacy field. This compilation contains 165,312 redshifts of 97,929 unique objects from 108 individual observing programs up to z ∼ 8 with median stellar mass ∼ 10^9 to 10^10 M_⊙ (redshift dependent). Rest-frame NUVrJ colors and SFR – stellar mass correlations show the compilation primarily contains low- to intermediate-mass star-forming and massive, quiescent galaxies at z < 1.25 and mostly low-mass bursty star-forming galaxies at z > 2. Sources in the compilation cover a diverse range of environments, including protoclusters such as “Hyperion”. The full compilation is 50% spectroscopically complete by i ∼ 23.2 and K_s ∼ 21.3 mag; however, this is redshift dependent. Spatially, the compilation is >50% complete within the CANDELS area, while the outer regions of COSMOS are >10% complete limited to i < 24 mag and K_S < 22.5 mag, separately. We demonstrate how the compilation can be used to validate photometric redshifts and investigate calibration metrics. By training self-organizing maps on COSMOS2020/Classic and projecting the compilation onto it, we find key galaxy subpopulations that currently lack spectroscopic coverage including z < 1 intermediate-mass quiescent galaxies and low-/intermediate-mass bursty star-forming galaxies, z ∼ 2 massive quiescent galaxies, and z > 3 massive star-forming galaxies. This approach highlights how combining self-organizing maps with our compilation can provide guidance for future spectroscopic observations to get a complete spectroscopic view of galaxy populations. Lastly, the compilation will undergo periodic data releases that incorporate new spectroscopic redshift measurements, providing a lasting legacy resource for the community.
MoreTranslated text
PDF
View via Publisher
AI Read Science
AI Summary
AI Summary is the key point extracted automatically understanding the full text of the paper, including the background, methods, results, conclusions, icons and other key content, so that you can get the outline of the paper at a glance.
Example
Background
Key content
Introduction
Methods
Results
Related work
Fund
Key content
- Pretraining has recently greatly promoted the development of natural language processing (NLP)
- We show that M6 outperforms the baselines in multimodal downstream tasks, and the large M6 with 10 parameters can reach a better performance
- We propose a method called M6 that is able to process information of multiple modalities and perform both single-modal and cross-modal understanding and generation
- The model is scaled to large model with 10 billion parameters with sophisticated deployment, and the 10 -parameter M6-large is the largest pretrained model in Chinese
- Experimental results show that our proposed M6 outperforms the baseline in a number of downstream tasks concerning both single modality and multiple modalities We will continue the pretraining of extremely large models by increasing data to explore the limit of its performance
Try using models to generate summary,it takes about 60s
Must-Reading Tree
Example
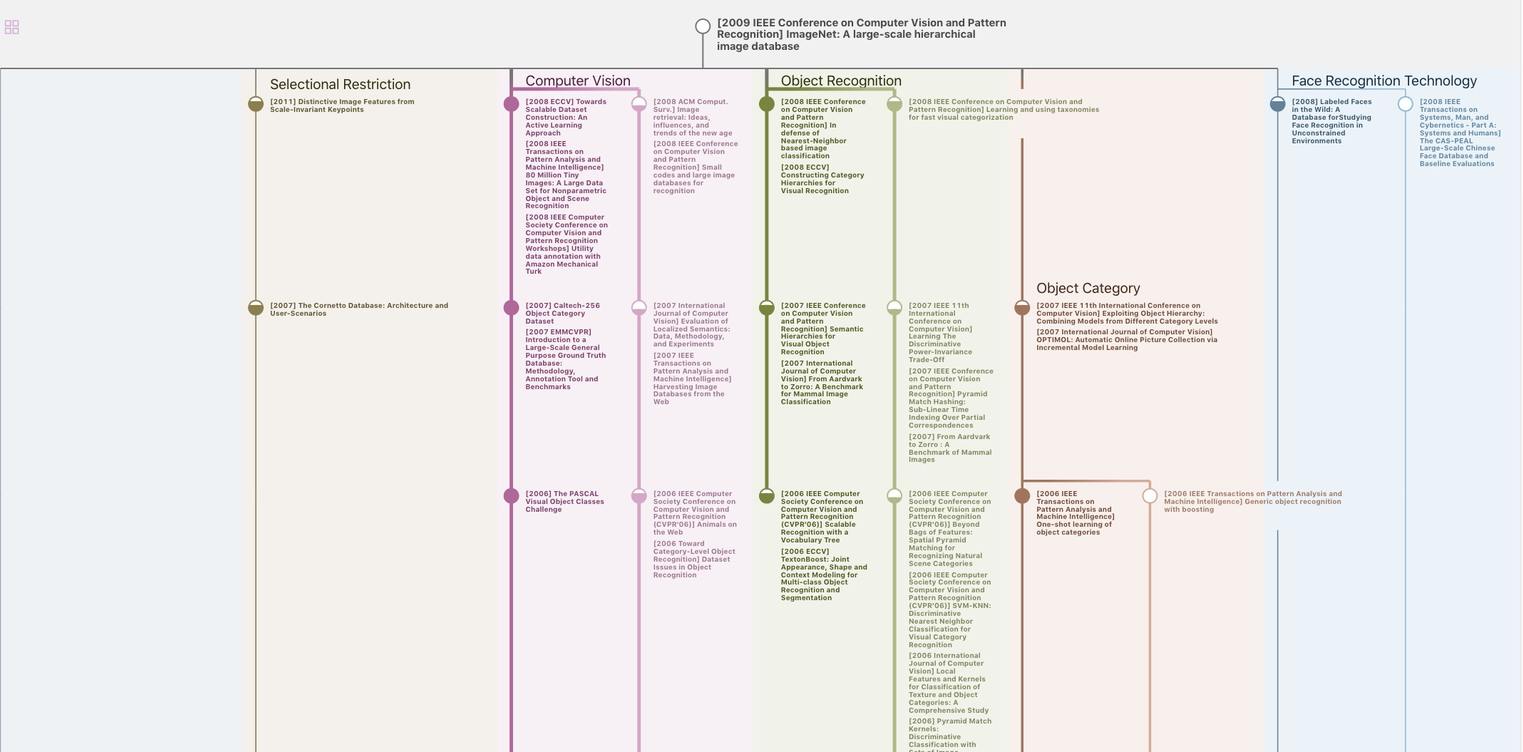
Generate MRT to find the research sequence of this paper
Data Disclaimer
The page data are from open Internet sources, cooperative publishers and automatic analysis results through AI technology. We do not make any commitments and guarantees for the validity, accuracy, correctness, reliability, completeness and timeliness of the page data. If you have any questions, please contact us by email: report@aminer.cn
Chat Paper
去 AI 文献库 对话