Improving Representation Learning of Complex Critical Care Data with ICU-BERT
arXiv · Machine Learning(2025)
Abstract
The multivariate, asynchronous nature of real-world clinical data, such as that generated in Intensive Care Units (ICUs), challenges traditional AI-based decision-support systems. These often assume data regularity and feature independence and frequently rely on limited data scopes and manual feature engineering. The potential of generative AI technologies has not yet been fully exploited to analyze clinical data. We introduce ICU-BERT, a transformer-based model pre-trained on the MIMIC-IV database using a multi-task scheme to learn robust representations of complex ICU data with minimal preprocessing. ICU-BERT employs a multi-token input strategy, incorporating dense embeddings from a biomedical Large Language Model to learn a generalizable representation of complex and multivariate ICU data. With an initial evaluation of five tasks and four additional ICU datasets, ICU-BERT results indicate that ICU-BERT either compares to or surpasses current performance benchmarks by leveraging fine-tuning. By integrating structured and unstructured data, ICU-BERT advances the use of foundational models in medical informatics, offering an adaptable solution for clinical decision support across diverse applications.
MoreTranslated text
PDF
View via Publisher
AI Read Science
AI Summary
AI Summary is the key point extracted automatically understanding the full text of the paper, including the background, methods, results, conclusions, icons and other key content, so that you can get the outline of the paper at a glance.
Example
Background
Key content
Introduction
Methods
Results
Related work
Fund
Key content
- Pretraining has recently greatly promoted the development of natural language processing (NLP)
- We show that M6 outperforms the baselines in multimodal downstream tasks, and the large M6 with 10 parameters can reach a better performance
- We propose a method called M6 that is able to process information of multiple modalities and perform both single-modal and cross-modal understanding and generation
- The model is scaled to large model with 10 billion parameters with sophisticated deployment, and the 10 -parameter M6-large is the largest pretrained model in Chinese
- Experimental results show that our proposed M6 outperforms the baseline in a number of downstream tasks concerning both single modality and multiple modalities We will continue the pretraining of extremely large models by increasing data to explore the limit of its performance
Try using models to generate summary,it takes about 60s
Must-Reading Tree
Example
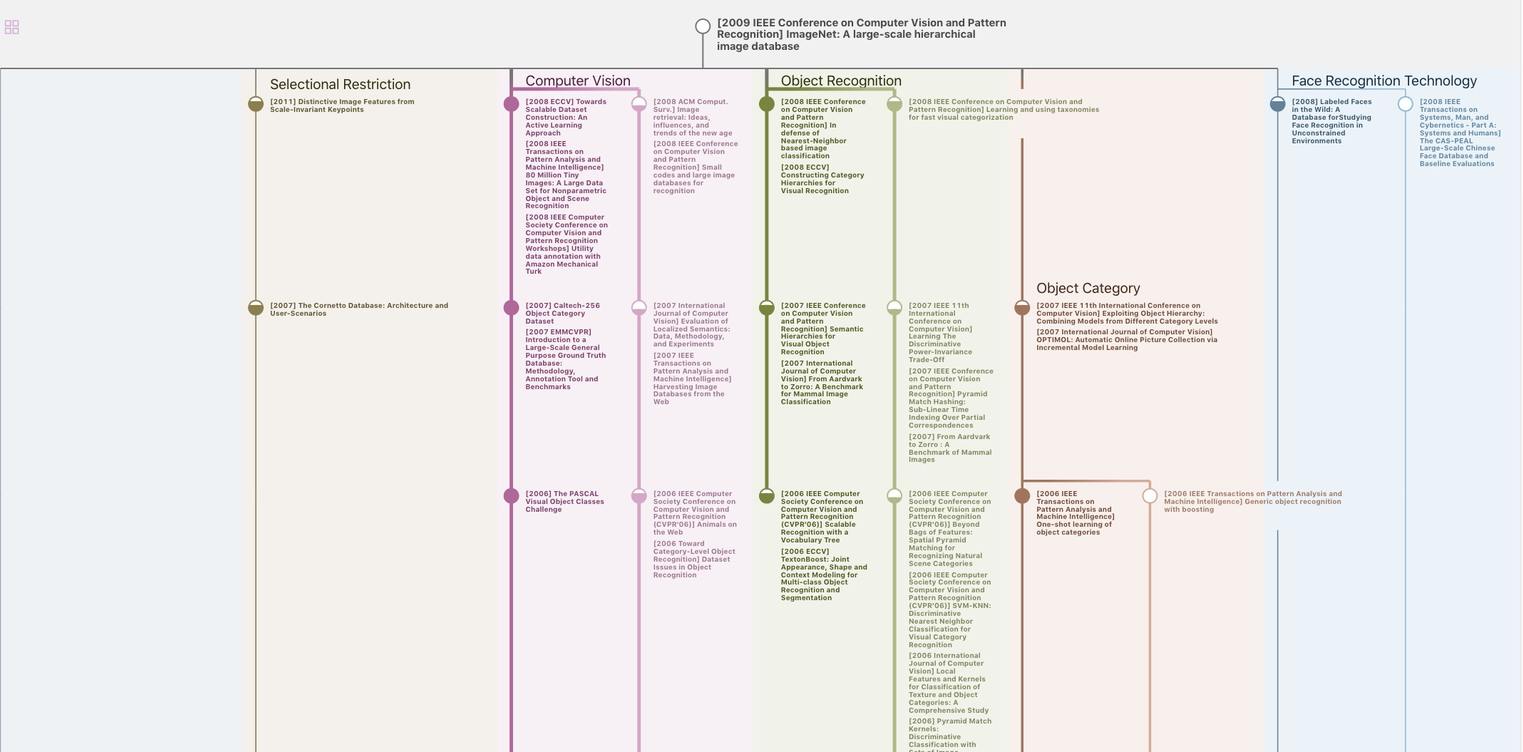
Generate MRT to find the research sequence of this paper
Data Disclaimer
The page data are from open Internet sources, cooperative publishers and automatic analysis results through AI technology. We do not make any commitments and guarantees for the validity, accuracy, correctness, reliability, completeness and timeliness of the page data. If you have any questions, please contact us by email: report@aminer.cn
Chat Paper
去 AI 文献库 对话