Identification of Putative Serum Autoantibodies Associated with Post-Acute Sequelae of COVID-19 Via Comprehensive Protein Array Analysis
International journal of molecular sciences(2025)
Department of Microbiology | Advanced Medical Research Center | Hirahata Clinic | Department of Public Health
Abstract
Post-acute sequelae of SARS-CoV-2 infection (PASC), commonly known as “Long COVID”, represents a significant clinical challenge characterized by persistent symptoms following acute COVID-19 infection. We conducted a comprehensive retrospective cohort study to identify serum autoantibody biomarkers associated with PASC. Initial screening using a protein bead array comprising approximately 20,000 human proteins identified several candidate PASC-associated autoantibodies. Subsequent validation by enzyme-linked immunosorbent assay (ELISA) in an expanded cohort—consisting of PASC patients, non-PASC COVID-19 convalescents, and pre-pandemic healthy controls—revealed two promising biomarkers: autoantibodies targeting PITX2 and FBXO2. PITX2 autoantibodies demonstrated high accuracy in distinguishing PASC patients from both non-PASC convalescents (area under the curve [AUC] = 0.891) and healthy controls (AUC = 0.866), while FBXO2 autoantibodies showed moderate accuracy (AUC = 0.762 and 0.786, respectively). Notably, the levels of these autoantibodies were associated with several PASC symptoms, including fever, dyspnea, palpitations, loss of appetite, and brain fog. The identification of PITX2 and FBXO2 autoantibodies as biomarkers not only enhances our understanding of PASC pathophysiology but also provides promising candidates for further investigation.
MoreTranslated text
Key words
SARS-CoV-2,autoantibody,protein array,long COVID
求助PDF
上传PDF
View via Publisher
AI Read Science
AI Summary
AI Summary is the key point extracted automatically understanding the full text of the paper, including the background, methods, results, conclusions, icons and other key content, so that you can get the outline of the paper at a glance.
Example
Background
Key content
Introduction
Methods
Results
Related work
Fund
Key content
- Pretraining has recently greatly promoted the development of natural language processing (NLP)
- We show that M6 outperforms the baselines in multimodal downstream tasks, and the large M6 with 10 parameters can reach a better performance
- We propose a method called M6 that is able to process information of multiple modalities and perform both single-modal and cross-modal understanding and generation
- The model is scaled to large model with 10 billion parameters with sophisticated deployment, and the 10 -parameter M6-large is the largest pretrained model in Chinese
- Experimental results show that our proposed M6 outperforms the baseline in a number of downstream tasks concerning both single modality and multiple modalities We will continue the pretraining of extremely large models by increasing data to explore the limit of its performance
Upload PDF to Generate Summary
Must-Reading Tree
Example
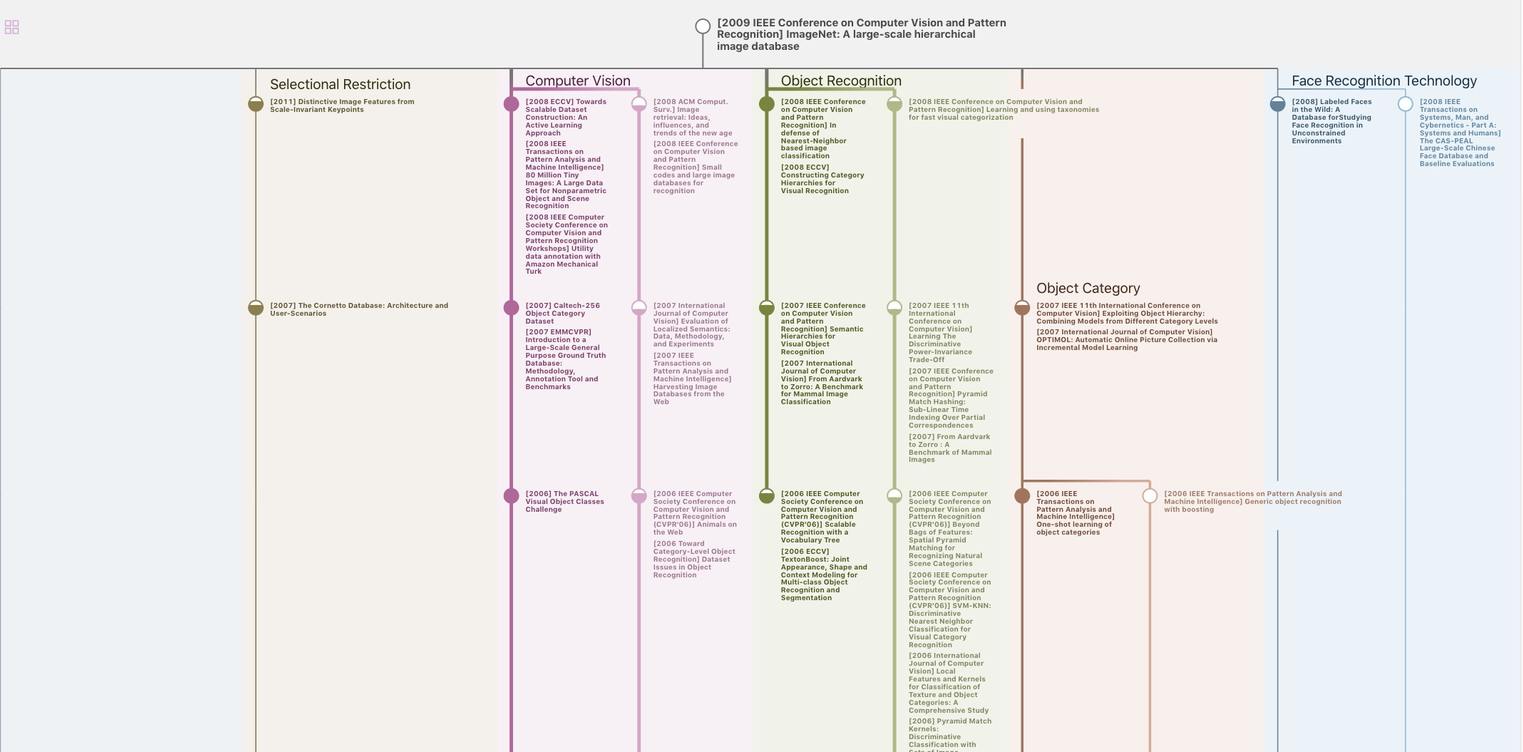
Generate MRT to find the research sequence of this paper
Data Disclaimer
The page data are from open Internet sources, cooperative publishers and automatic analysis results through AI technology. We do not make any commitments and guarantees for the validity, accuracy, correctness, reliability, completeness and timeliness of the page data. If you have any questions, please contact us by email: report@aminer.cn
Chat Paper
GPU is busy, summary generation fails
Rerequest