Construction of Quasi-Localized Dual Bases in Reproducing Kernel Hilbert Spaces
Journal of Computational and Applied Mathematics(2025)
Departement Mathematik und Informatik | Istituto Eulero
Abstract
A straightforward way to represent the kernel approximant of a function, known by a finite set of samples, within a reproducing kernel Hilbert space is through the canonical dual pair. The canonical dual pair consists of the basis of kernel translates and the corresponding Lagrange basis. From a numerical perspective, one is particularly interested in dual pairs such that the dual bases are quasi-local meaning that they can be well approximated using only a small subset of the data sites. This implies that the inverse Gramian is approximately sparse. In this case, the kernel approximant is efficiently computable by multiplying a sparse matrix with the data vector. We present two methods for finding such quasi-localized dual bases. First, we adapt the idea of localizing the Lagrange basis which yields an approximate canonical dual pair and extend this idea to derive a new, symmetric preconditioner for kernel matrices. Second, we use samplets to obtain multiresolution versions of dual bases. Samplets are localized discrete signed measures constructed such that their respective measure integrals of polynomials up to a certain degree vanish. Therefore, the kernel matrix and its inverse are compressible to sparse matrices in samplet coordinates for asymptotically smooth kernels. We provide benchmark experiments in two spatial dimensions to demonstrate the compression power of both approaches and apply the new preconditioner to implicit surface reconstruction in computer graphics.
MoreTranslated text
Key words
Reproducing kernel Hilbert spaces,Dual pairs,Preconditioning
求助PDF
上传PDF
View via Publisher
AI Read Science
AI Summary
AI Summary is the key point extracted automatically understanding the full text of the paper, including the background, methods, results, conclusions, icons and other key content, so that you can get the outline of the paper at a glance.
Example
Background
Key content
Introduction
Methods
Results
Related work
Fund
Key content
- Pretraining has recently greatly promoted the development of natural language processing (NLP)
- We show that M6 outperforms the baselines in multimodal downstream tasks, and the large M6 with 10 parameters can reach a better performance
- We propose a method called M6 that is able to process information of multiple modalities and perform both single-modal and cross-modal understanding and generation
- The model is scaled to large model with 10 billion parameters with sophisticated deployment, and the 10 -parameter M6-large is the largest pretrained model in Chinese
- Experimental results show that our proposed M6 outperforms the baseline in a number of downstream tasks concerning both single modality and multiple modalities We will continue the pretraining of extremely large models by increasing data to explore the limit of its performance
Upload PDF to Generate Summary
Must-Reading Tree
Example
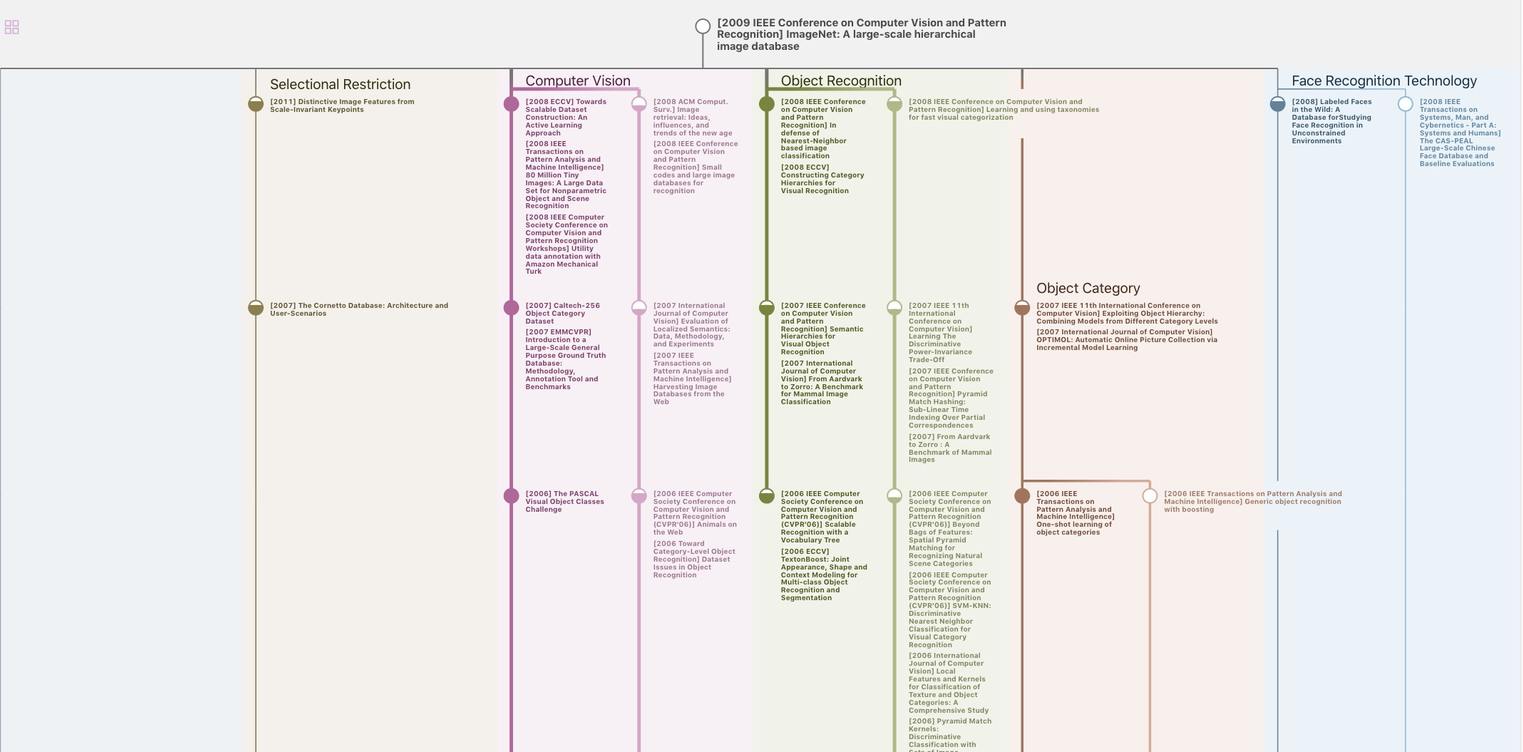
Generate MRT to find the research sequence of this paper
Data Disclaimer
The page data are from open Internet sources, cooperative publishers and automatic analysis results through AI technology. We do not make any commitments and guarantees for the validity, accuracy, correctness, reliability, completeness and timeliness of the page data. If you have any questions, please contact us by email: report@aminer.cn
Chat Paper