Event-Triggered Newton-Based Extremum Seeking Control
Computing Research Repository (CoRR)(2025)
Abstract
This paper proposes the incorporation of static event-triggered control in the actuation path of Newton-based extremum seeking and its comparison with the earlier gradient version. As in the continuous methods, the convergence rate of the gradient approach depends on the unknown Hessian of the nonlinear map to be optimized, whereas the proposed event-triggered Newton-based extremum seeking eliminates this dependence, becoming user-assignable. This is achieved by means of a dynamic estimator for the Hessian's inverse, implemented as a Riccati equation filter. Lyapunov stability and averaging theory for discontinuous systems are applied to analyze the closed-loop system. Local exponential practical stability is guaranteed to a small neighborhood of the extremum point of scalar and static maps. Numerical simulations illustrate the advantages of the proposed approach over the previous gradient method, including improved convergence speed, followed by a reduction in the amplitude and updating frequency of the control signals.
MoreTranslated text
PDF
View via Publisher
AI Read Science
AI Summary
AI Summary is the key point extracted automatically understanding the full text of the paper, including the background, methods, results, conclusions, icons and other key content, so that you can get the outline of the paper at a glance.
Example
Background
Key content
Introduction
Methods
Results
Related work
Fund
Key content
- Pretraining has recently greatly promoted the development of natural language processing (NLP)
- We show that M6 outperforms the baselines in multimodal downstream tasks, and the large M6 with 10 parameters can reach a better performance
- We propose a method called M6 that is able to process information of multiple modalities and perform both single-modal and cross-modal understanding and generation
- The model is scaled to large model with 10 billion parameters with sophisticated deployment, and the 10 -parameter M6-large is the largest pretrained model in Chinese
- Experimental results show that our proposed M6 outperforms the baseline in a number of downstream tasks concerning both single modality and multiple modalities We will continue the pretraining of extremely large models by increasing data to explore the limit of its performance
Try using models to generate summary,it takes about 60s
Must-Reading Tree
Example
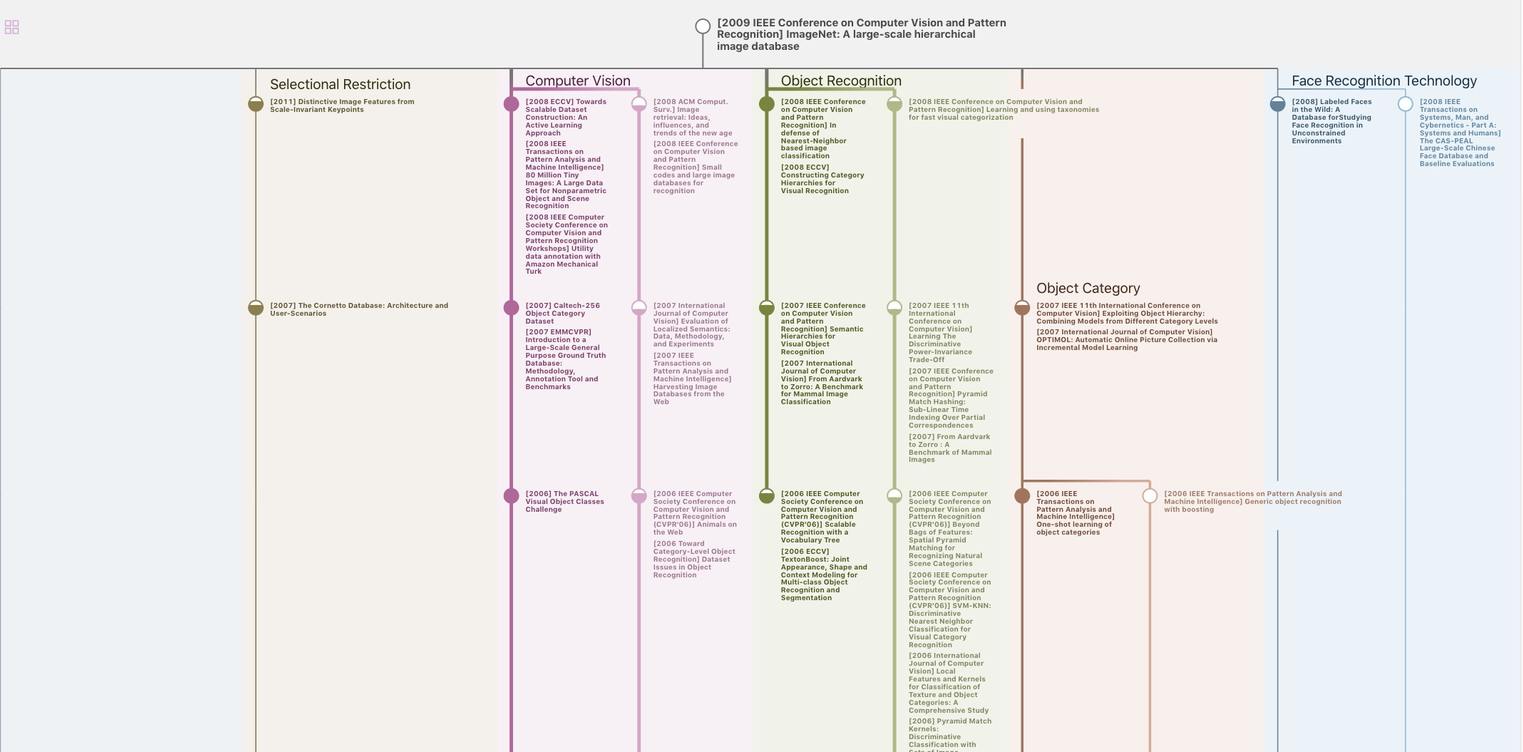
Generate MRT to find the research sequence of this paper
Data Disclaimer
The page data are from open Internet sources, cooperative publishers and automatic analysis results through AI technology. We do not make any commitments and guarantees for the validity, accuracy, correctness, reliability, completeness and timeliness of the page data. If you have any questions, please contact us by email: report@aminer.cn
Chat Paper
去 AI 文献库 对话