Factors Associated with Mortality in Nosocomial Lower Respiratory Tract Infections: an ENIRRI Analysis
Antibiotics (Basel, Switzerland)(2025)
School of Medicine | Médecine Intensive-Réanimation | NOVA Medical School | Intensive Care | Hospital Joan XXIII de Tarragona | "Dr Fran Mihaljevic" University Hospital for Infectious Diseases | Medical Faculty | Hospital Nacional Alejandro Posadas | Hospital del Mar | Hospital Arnau de Vilanova de Lleida | ASST Santi Paolo e Carlo | Chest Department | St James's University Hospital
Abstract
Background: Nosocomial lower respiratory tract infections (nLRTIs) are associated with unfavorable clinical outcomes and significant healthcare costs. nLRTIs include hospital-acquired pneumonia (HAP), ventilator-associated pneumonia (VAP), and other ICU-acquired pneumonia phenotypes. While risk factors for mortality in these infections are critical to guide preventive strategies, it remains unclear whether they vary based on their requirement of invasive mechanical ventilation (IMV) at any point during the hospitalization. Objectives: This study aims to identify risk factors associated with short- and long-term mortality in patients with nLRTIs, considering differences between those requiring IMV and those who do not. Methods: This multinational prospective cohort study included ICU-admitted patients diagnosed with nLRTI from 28 hospitals across 13 countries in Europe and South America between May 2016 and August 2019. Patients were selected based on predefined inclusion and exclusion criteria, and clinical data were collected from medical records. A random forest classifier determined the most optimal clustering strategy when comparing pneumonia site acquisition [ward or intensive care unit (ICU)] versus intensive mechanical ventilation (IMV) necessity at any point during hospitalization to enhance the accuracy and generalizability of the regression models. Results: A total of 1060 patients were included. The random forest classifier identified that the most efficient clustering strategy was based on ventilation necessity. In total, 76.4% of patients [810/1060] received IMV at some point during the hospitalization. Diabetes mellitus was identified to be associated with 28-day mortality in the non-IMV group (OR [IQR]: 2.96 [1.28–6.80], p = 0.01). The 90-day mortality-associated factor was MDRP infection (1.98 [1.13–3.44], p = 0.01). For ventilated patients, chronic liver disease was associated with 28-day mortality (2.38 [1.06–5.31] p = 0.03), with no variable showing statistical and clinical significance at 90 days. Conclusions: The risk factors associated with 28-day mortality differ from those linked to 90-day mortality. Additionally, these factors vary between patients receiving invasive mechanical ventilation and those in the non-invasive ventilation group. This underscores the necessity of tailoring therapeutic objectives and preventive strategies with a personalized approach.
MoreTranslated text
Key words
critical care,mechanical ventilation,nosocomial lower respiratory tract infections
求助PDF
上传PDF
View via Publisher
AI Read Science
AI Summary
AI Summary is the key point extracted automatically understanding the full text of the paper, including the background, methods, results, conclusions, icons and other key content, so that you can get the outline of the paper at a glance.
Example
Background
Key content
Introduction
Methods
Results
Related work
Fund
Key content
- Pretraining has recently greatly promoted the development of natural language processing (NLP)
- We show that M6 outperforms the baselines in multimodal downstream tasks, and the large M6 with 10 parameters can reach a better performance
- We propose a method called M6 that is able to process information of multiple modalities and perform both single-modal and cross-modal understanding and generation
- The model is scaled to large model with 10 billion parameters with sophisticated deployment, and the 10 -parameter M6-large is the largest pretrained model in Chinese
- Experimental results show that our proposed M6 outperforms the baseline in a number of downstream tasks concerning both single modality and multiple modalities We will continue the pretraining of extremely large models by increasing data to explore the limit of its performance
Upload PDF to Generate Summary
Must-Reading Tree
Example
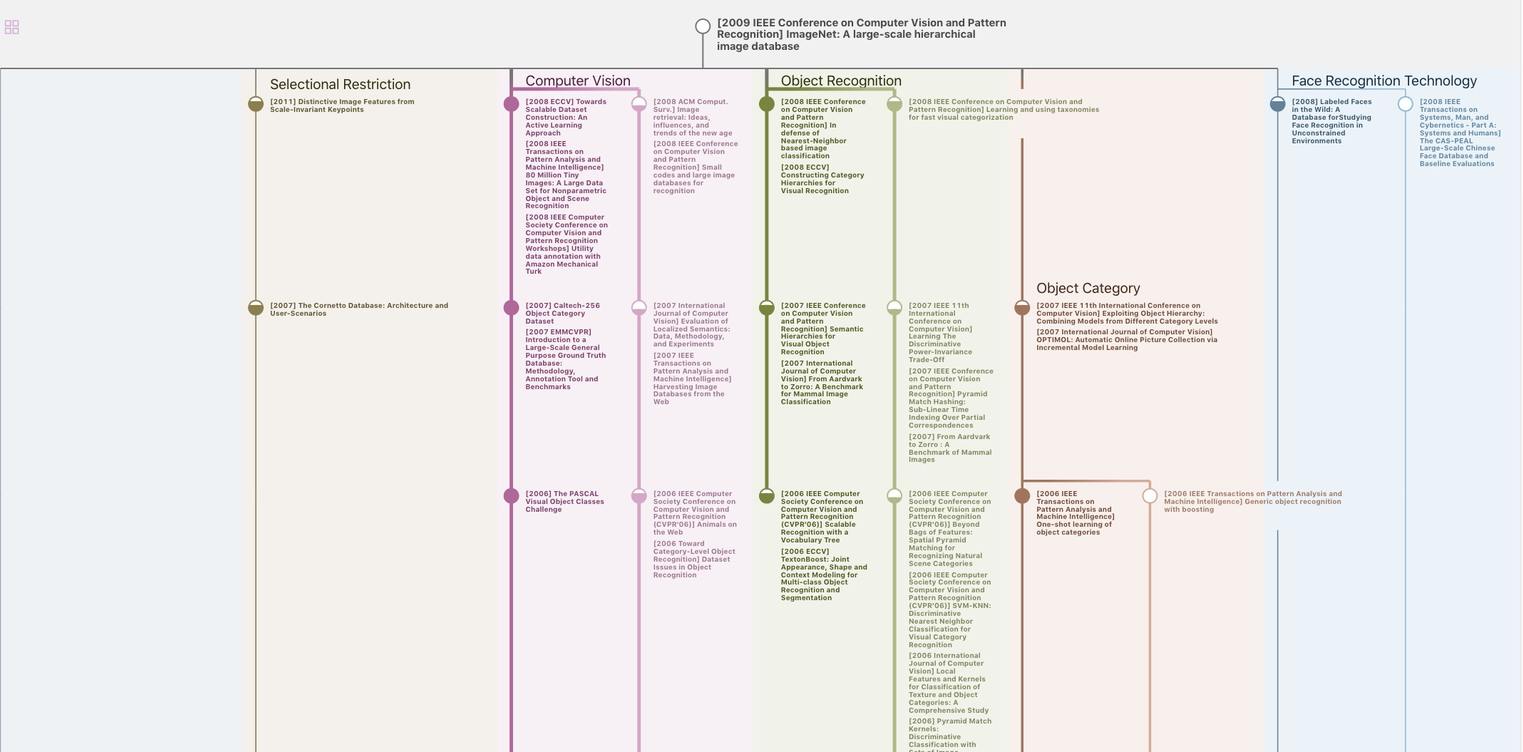
Generate MRT to find the research sequence of this paper
Data Disclaimer
The page data are from open Internet sources, cooperative publishers and automatic analysis results through AI technology. We do not make any commitments and guarantees for the validity, accuracy, correctness, reliability, completeness and timeliness of the page data. If you have any questions, please contact us by email: report@aminer.cn
Chat Paper